Multi-Attribute Self-Attention Guided Vehicle Local Region Detection Based On Convolutional Neural Network Architecture
INTERNATIONAL JOURNAL OF ADVANCED ROBOTIC SYSTEMS(2020)
摘要
Many pieces of information are included in the front region of a vehicle, especially in windshield and bumper regions. Thus, windshield or bumper region detection is making sense to extract useful information. But the existing windshield and bumper detection methods based on traditional artificial features are not robust enough. Those features may become invalid in many real situations (e.g. occlude, illumination change, viewpoint change.). In this article, we propose a multi-attribute-guided vehicle discriminately region detection method based on convolutional neural network and not rely on bounding box regression. We separate the net into two branches, respectively, for identification (ID) and Model attributes training. Therefore, the feature spaces of different attributes become more independent. Additionally, we embed a self-attention block into our framework to improve the performance of local region detection. We train our model onPKU_VDdata set which has a huge number of images inside. Furthermore, we labeled the handcrafted bounding boxes on 5000 randomly picked testing images, and 1020 of them are used for evaluation and 3980 as the training data forYOLOv3. We use Intersection over Union for quantitative evaluation. Experiments were conducted in three different latest convolutional neural network trunks to illustrate the detection performance of the proposed method. Simultaneously, in terms of quantitative evaluation, the performance of our method is close toYOLOv3even without handcrafted bounding boxes.
更多查看译文
关键词
Vehicle local region detection, multi-attribute, self-attention
AI 理解论文
溯源树
样例
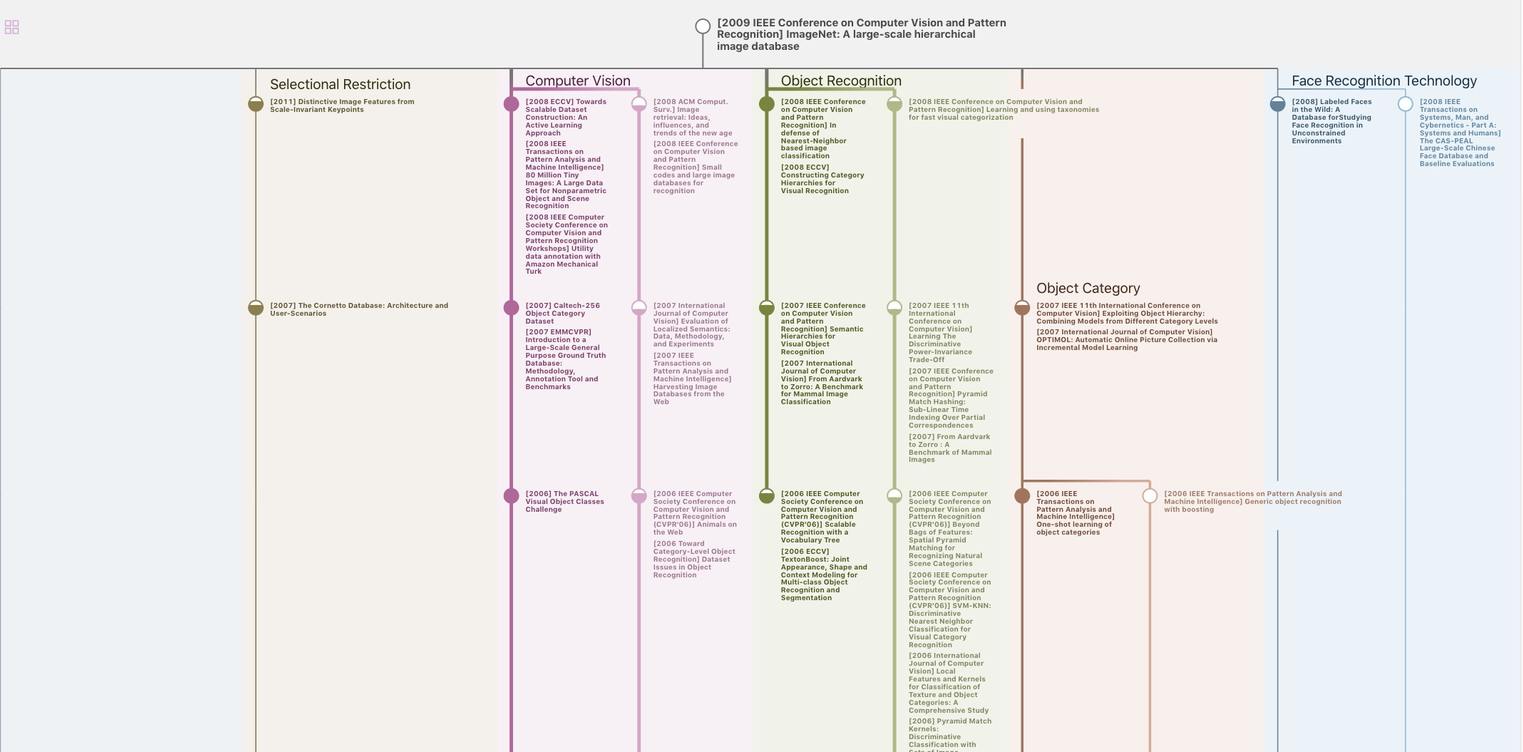
生成溯源树,研究论文发展脉络
Chat Paper
正在生成论文摘要