Is This Possible To Predict Delayed Graft Function After Kidney Transplantation? Preliminary Study On Possible Application Of Machine Learning In Early Routine Post-Kidney Transplant Care
TRANSPLANTATION(2020)
摘要
Introduction: Delayed graft function (DGF) challenges kidney transplantation. Novel computational approaches such as machine learning are capable to provide tools to assess the risk of DGF. These can be based on routinely generated medical data. Objectives: The aim of this study was to stratify the risk for DGF using routinely available data reanalyzed with machine learning algorithms. Patients and Methods: The group of 119 kidney recipients who underwent kidney transplantation between 2011-2015 was analyzed. Analytical pipeline containing both supervised and unsupervised machine learning algorithms in combination with traditional statistics was implemented. DGF identified as the need for heamodialysis during the first week post-transplant and the dynamics of creatinine decraease early post-transplant were targeted. Results: Relevant classification and regression models were obtained. The spectrum of variables was found associated with DGF itself and the dynamics of creatinine decrease. The parameters such as lymphocytes count, pre-transplant dialysis period and cold ischaemia time pointed in the models were also found statistically significant. Conclusions: Routinely generated medical data are relevant to explore in association with DGF onset. Machine learning implementation revealed the parameters which have not been previously strongly considered in the context of DGF. Integrated data collection is needed in order to provide relevant prediction with regard the variations between patients groups.
更多查看译文
关键词
kidney transplantation,delayed graft function,machine learning,post-kidney
AI 理解论文
溯源树
样例
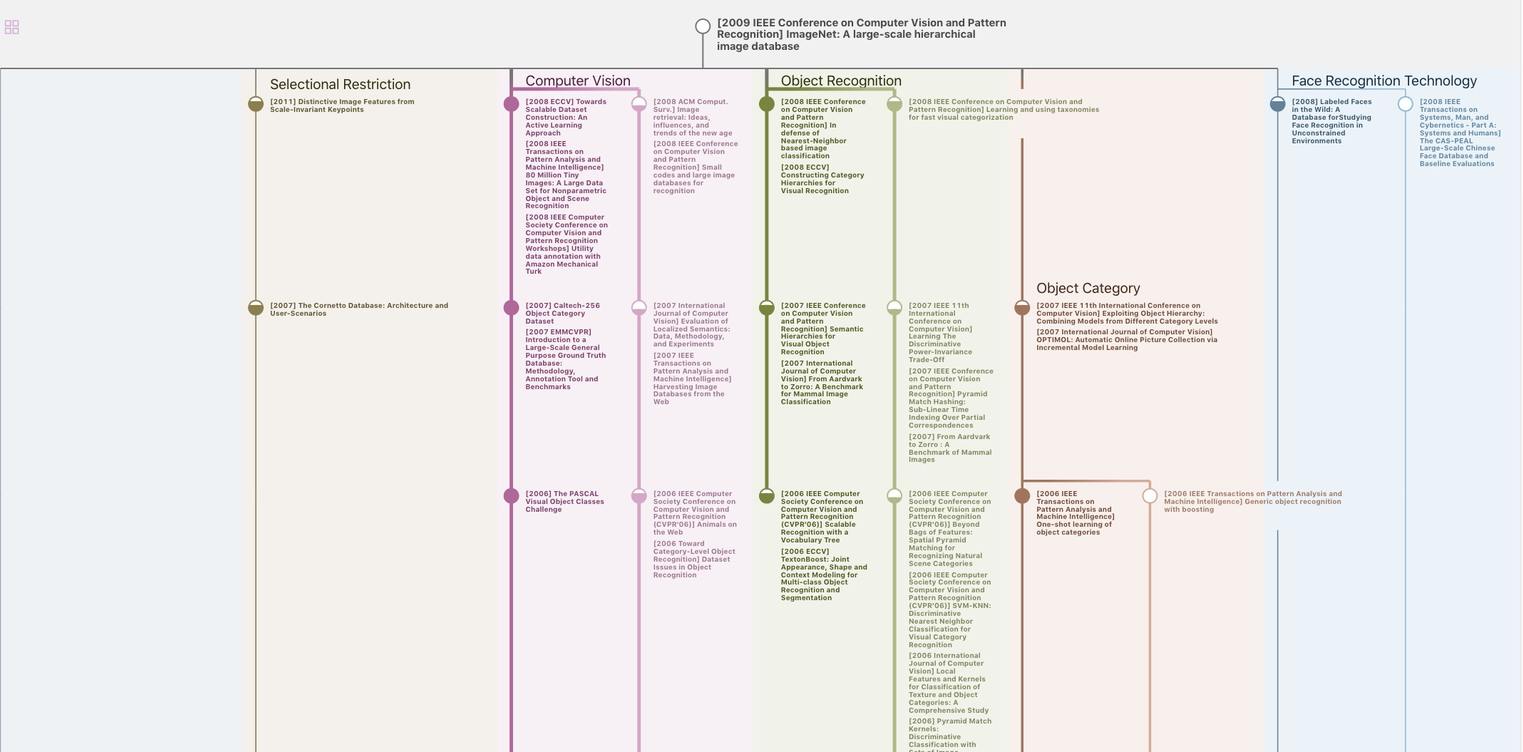
生成溯源树,研究论文发展脉络
Chat Paper
正在生成论文摘要