Assigning Fates In Telemetry Studies Using Hidden Markov Models: An Application To Deepwater Groupers Released With Descender Devices
NORTH AMERICAN JOURNAL OF FISHERIES MANAGEMENT(2020)
摘要
Fate assignment is crucial to the results of survival studies, particularly those that utilize acoustic tagging. Most current methodologies are at least partially subjective; thus, having a means of objectively assigning fates would improve the precision, accuracy, and utility of such studies. We released 57 acoustically tagged fish belonging to six deepwater grouper species off North Carolina, USA, via surface release and recompressed release with descender devices. We applied a three-state hidden Markov model (HMM) in a novel way: to distinguish movement patterns between fish that were alive and fish that were dead (and might have been eaten by predators). We assigned fates using two approaches that differed in their reliance on HMMs. When HMMs were the predominant source of fate assignment, we estimated survival of 40 deepwater groupers released with descender devices at the continental shelf break (66-120-m depth) to be 0.46 (95% CI = 0.33-0.65). When a combination of HMMs and prior information was utilized, we estimated survival of the same 40 groupers to be 0.61 (95% CI = 0.47-0.80). Both estimates represented a substantial improvement over the survival of surface-released groupers (n = 9; survival similar to 0.0). Furthermore, HMMs estimated zero survival for an additional five descended groupers at a wreck site in 240-m depth, although one analysis using prior information suggested that survival was possible in that depth. These estimates were aided by the objectivity of HMMs, and we recommend that future survival studies involving acoustic tagging employ similar methodologies. The improved survival of groupers after descending is an important finding for management, as this taxon contains several species of impaired stock status or fishery status.
更多查看译文
关键词
deepwater groupers,telemetry studies,hidden markov models,descender devices
AI 理解论文
溯源树
样例
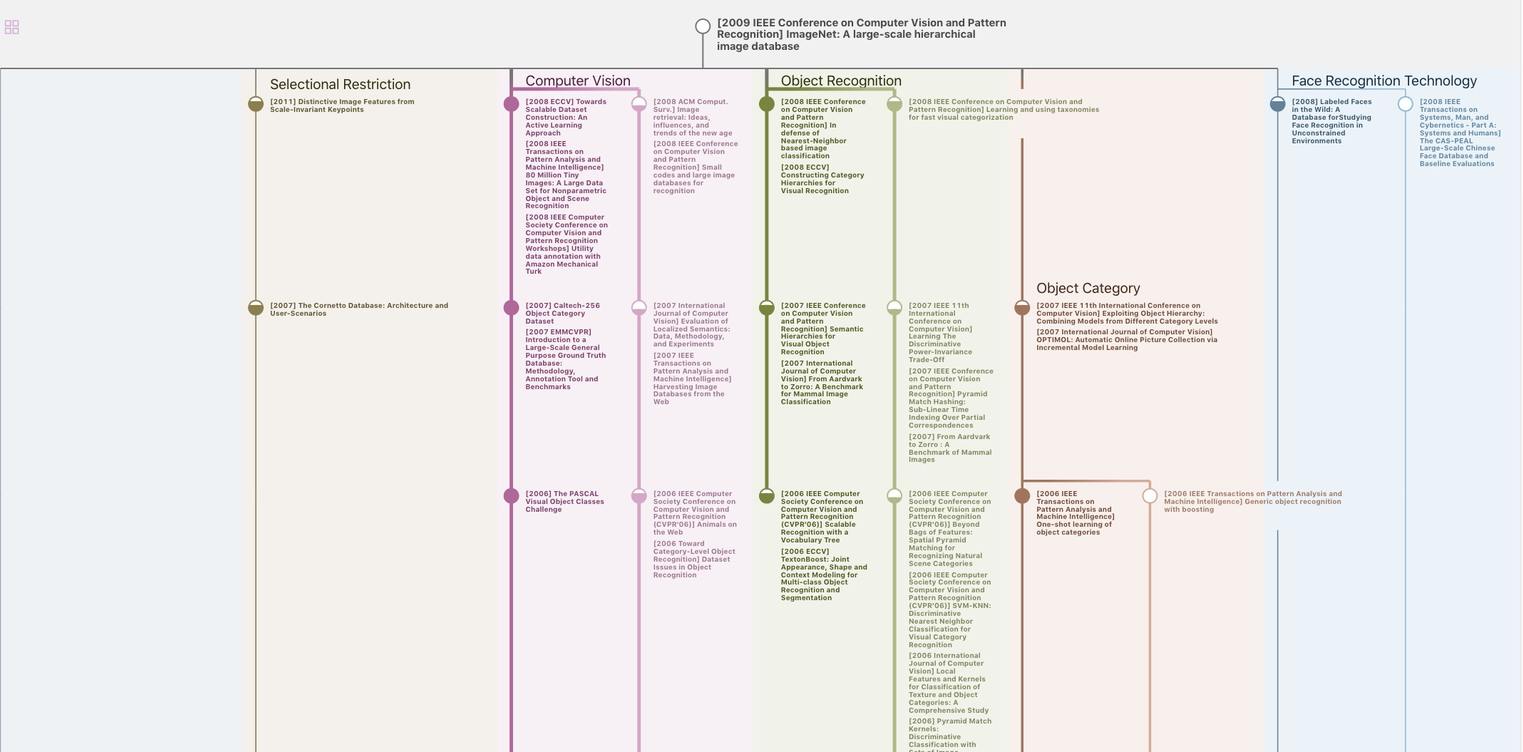
生成溯源树,研究论文发展脉络
Chat Paper
正在生成论文摘要