Using Tree Structure to Mine High Temporal Fuzzy Utility Itemsets
IEEE ACCESS(2020)
摘要
Data mining is a critical technology for extracting valuable knowledge from databases. It has been used in many fields, like retail, finance, biology, etc. In computational intelligence, fuzzy logic has been applied in many intelligent systems widely because it is simple and similar to human inference. Fuzzy utility mining combines utility mining and fuzzy logic for getting linguistic utility knowledge. In this paper, we study a more challenging, complicated, but practical topic called temporal fuzzy utility data mining, which considers the temporal periods in transactions, purchased amounts, item profits, and understandable linguistic terms as important factors. Although an Apriori-based algorithm was proposed previously, its execution was not efficient. We thus use a modified tree structure based on the classical frequent-pattern tree to improve its performance. A tree-based mining algorithm is also proposed to mine temporal fuzzy utility itemsets from quantitative transactional databases. The tree structure is built to keep all temporal fuzzy utility 1-itemsets in a database. All the high temporal fuzzy utility itemsets in a database can be obtained by traversing the tree-based structure. The proposed algorithm gets the final results through two phases. In the first phase, a procedure like FP-Growth is used to find the candidate itemsets. In the second phase, the temporal fuzzy utility database is scanned to decide whether the candidate itemsets are desired. Experimental results show that the proposed algorithm is superior to the existing algorithm for temporal fuzzy utility mining in terms of processing time and used memory.
更多查看译文
关键词
Fuzzy set,quantitative database,temporal fuzzy utility mining,tree structure,utility mining
AI 理解论文
溯源树
样例
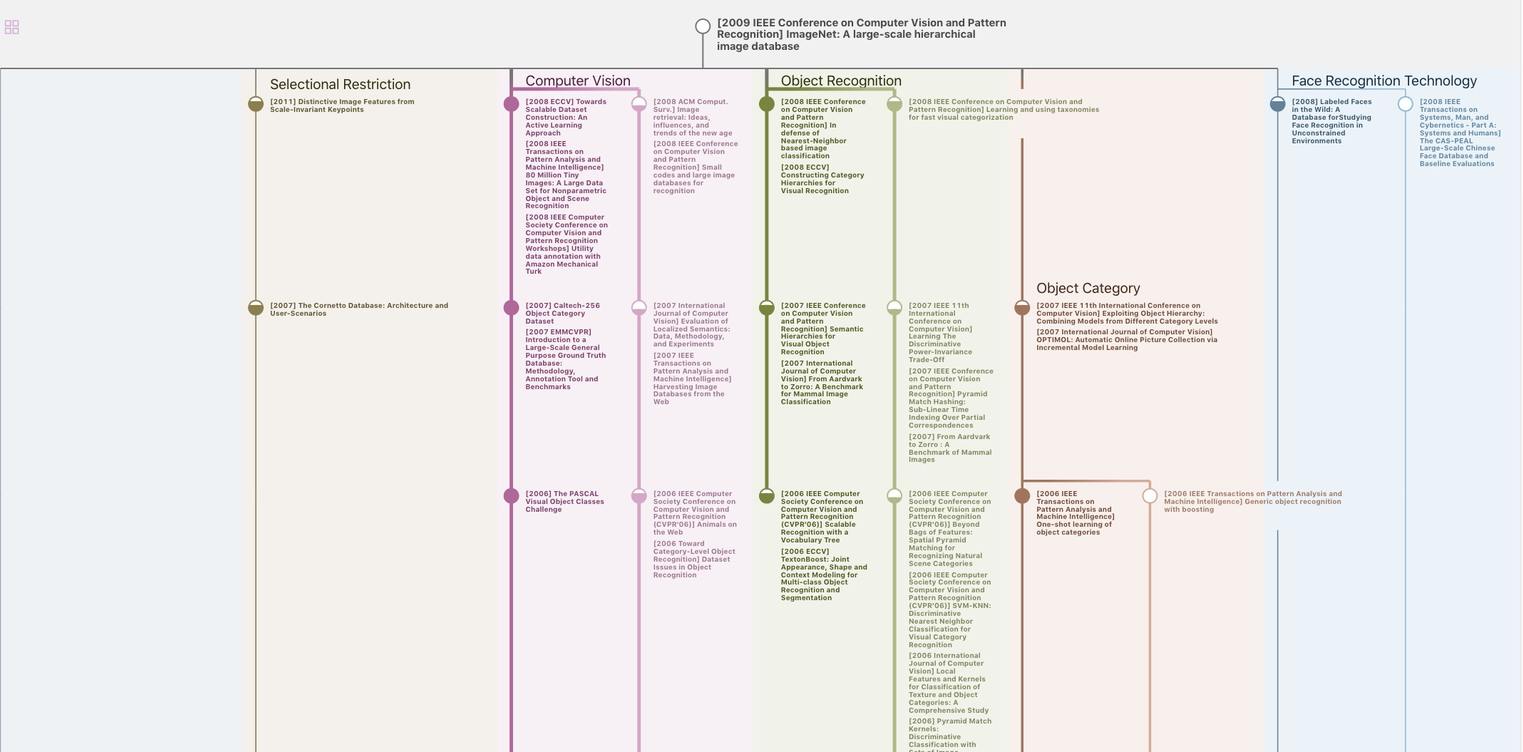
生成溯源树,研究论文发展脉络
Chat Paper
正在生成论文摘要