An Improved Deep Fusion CNN for Image Recognition
CMC-COMPUTERS MATERIALS & CONTINUA(2020)
摘要
With the development of Deep Convolutional Neural Networks (DCNNs), the extracted features for image recognition tasks have shifted from low-level features to the high-level semantic features of DCNNs. Previous studies have shown that the deeper the network is, the more abstract the features are. However, the recognition ability of deep features would be limited by insufficient training samples. To address this problem, this paper derives an improved Deep Fusion Convolutional Neural Network (DF-Net) which can make full use of the differences and complementarities during network learning and enhance feature expression under the condition of limited datasets. Specifically, DF-Net organizes two identical subnets to extract features from the input image in parallel, and then a well-designed fusion module is introduced to the deep layer of DF-Net to fuse the subnet's features in multi-scale. Thus, the more complex mappings are created and the more abundant and accurate fusion features can be extracted to improve recognition accuracy. Furthermore, a corresponding training strategy is also proposed to speed up the convergence and reduce the computation overhead of network training. Finally, DF-Nets based on the well-known ResNet, DenseNet and MobileNetV2 are evaluated on CIFAR100, Stanford Dogs, and UECFOOD-100. Theoretical analysis and experimental results strongly demonstrate that DF-Net enhances the performance of DCNNs and increases the accuracy of image recognition.
更多查看译文
关键词
Deep convolutional neural networks,deep features,image recognition,deep fusion,feature fusion
AI 理解论文
溯源树
样例
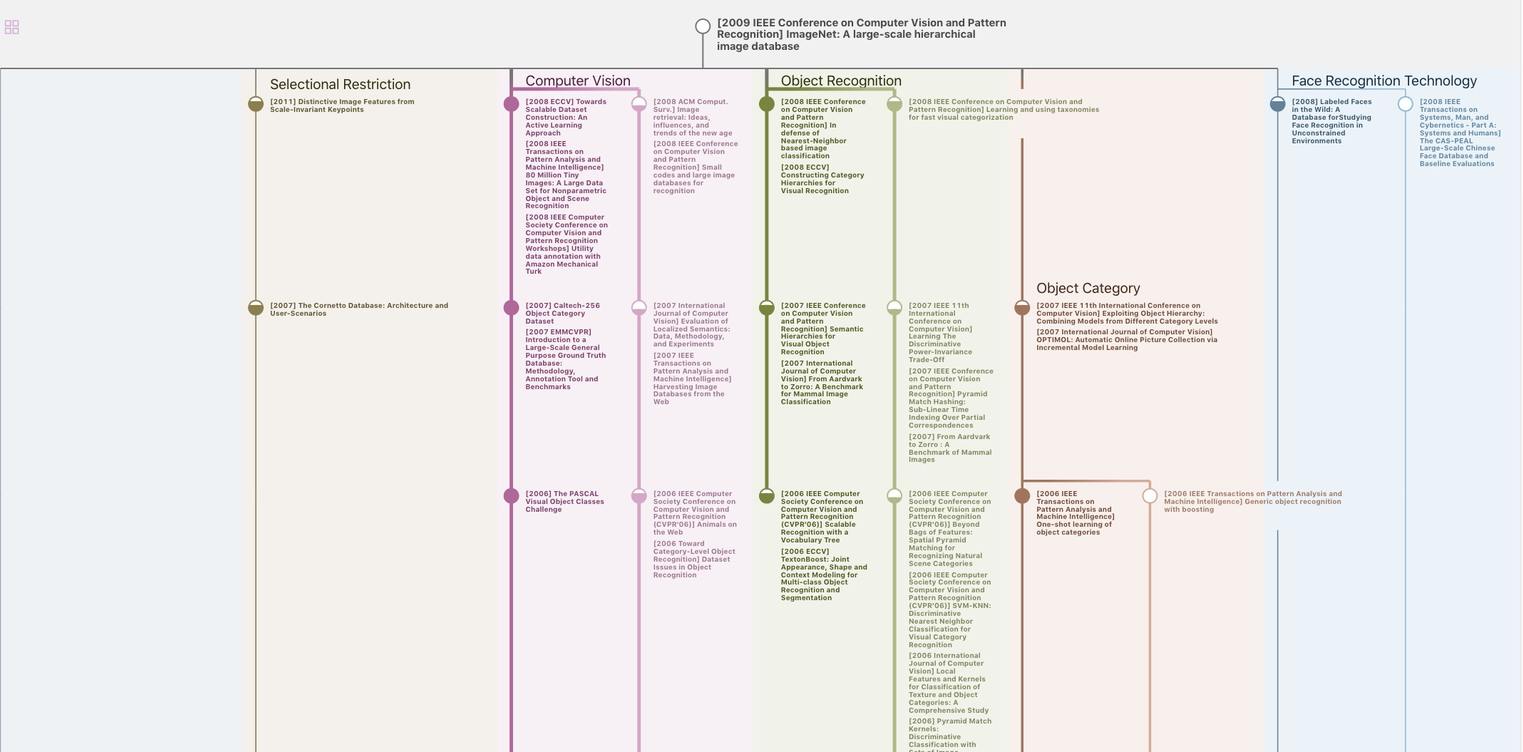
生成溯源树,研究论文发展脉络
Chat Paper
正在生成论文摘要