Emotion Recognition from Multidimensional Electroencephalographic Signals on the Manifold of Symmetric Positive Definite Matrices
2020 IEEE Conference on Multimedia Information Processing and Retrieval (MIPR)(2020)
摘要
The aim of this study is to classify human emotions using Electroencephalographic (EEG) signals. The main contribution of our approach is classifying four classes of emotions using a simple distance metric Log-Euclidean Riemannian Metric (LERM) on a symmetric positive definite manifold (SPD). In this work, four classes of emotions were recognized (HVHA, LVHA, LVLA, and HVLA) using four different channel combinations (2-channels, 7-channels, 10-channels, and 18-channels) over four frequency bands (theta, alpha, beta, and gamma). Our approach shows comparable results to existing studies applied on the DEAP dataset. The best emotion classification accuracy for HVHA is 88:3%, LVHA is 84:38%, LVLA is 79:3%, and HVLA is 78:4%. The average recognition accuracy for valence is 74:6% ± 3,9, and 72:6% ± 6,7 for arousal.
更多查看译文
关键词
DEAP dataset,metric Log-Euclidean Riemannian Metrics,multidimensional electroencephalographic signals,emotion classification,human emotions,emotion recognition
AI 理解论文
溯源树
样例
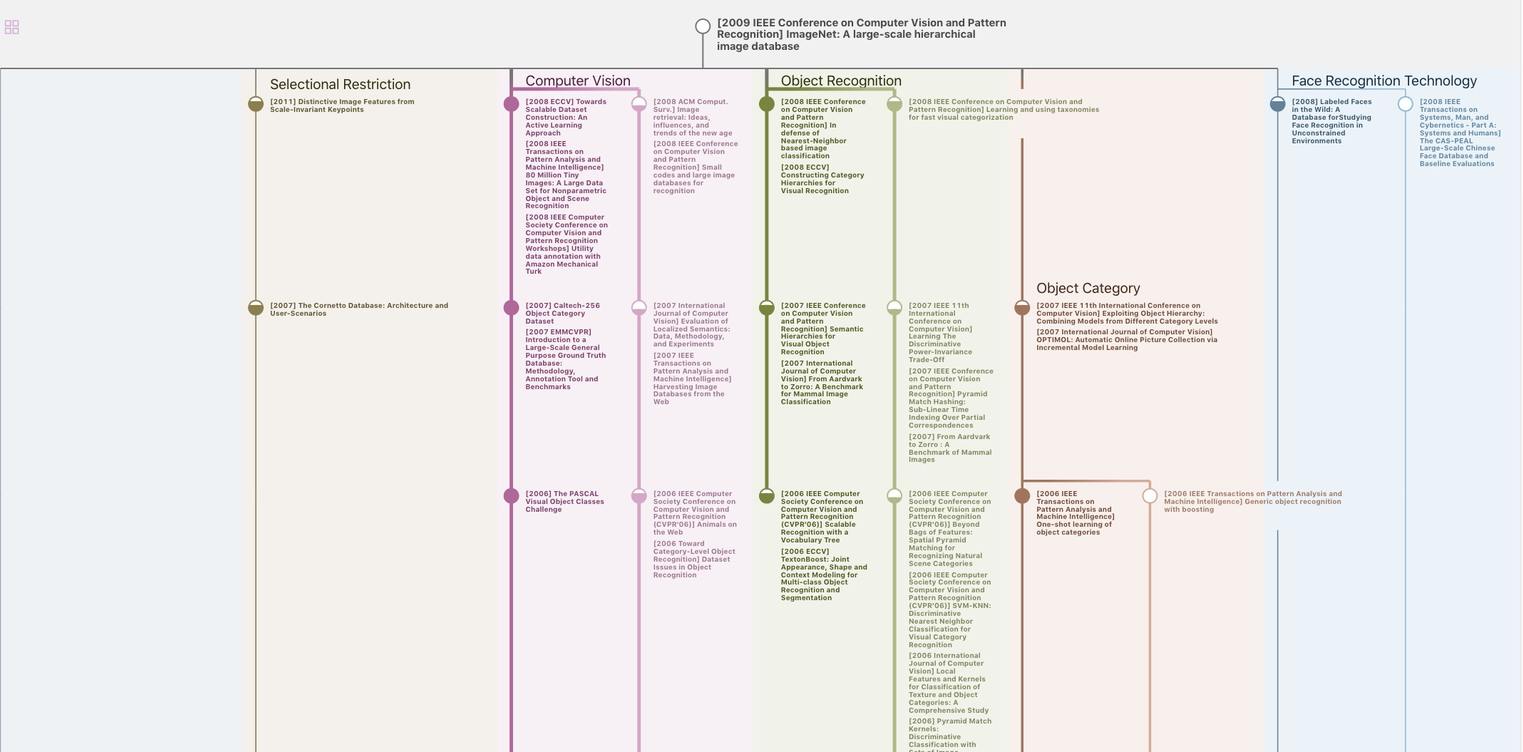
生成溯源树,研究论文发展脉络
Chat Paper
正在生成论文摘要