AutoIDL: Automated Imbalanced Data Learning via Collaborative Filtering.
KSEM (2)(2020)
摘要
AutoML aims to select an appropriate classification algorithm and corresponding hyperparameters for an individual dataset. However, existing AutoML methods usually ignore the intrinsic imbalance nature of most real-world datasets and lead to poor performance. For handling imbalanced data, sampling methods have been widely used since their independence of the used algorithms. We propose a method named AutoIDL for selecting the sampling methods as well as classification algorithms simultaneously. Particularly, AutoIDL firstly represents datasets as graphs and extracts their meta-features with a graph embedding method. In addition, meta-targets are identified as pairs of sampling methods and classification algorithms for each imbalanced dataset. Secondly, the user-based collaborative filtering method is employed to train a ranking model based on the meta repository to select appropriate sampling methods and algorithms for a new dataset. Extensive experimental results demonstrate that AutoIDL is effective for automated imbalanced data learning and it outperforms the state-of-the-art AutoML methods.
更多查看译文
关键词
automated imbalanced data learning
AI 理解论文
溯源树
样例
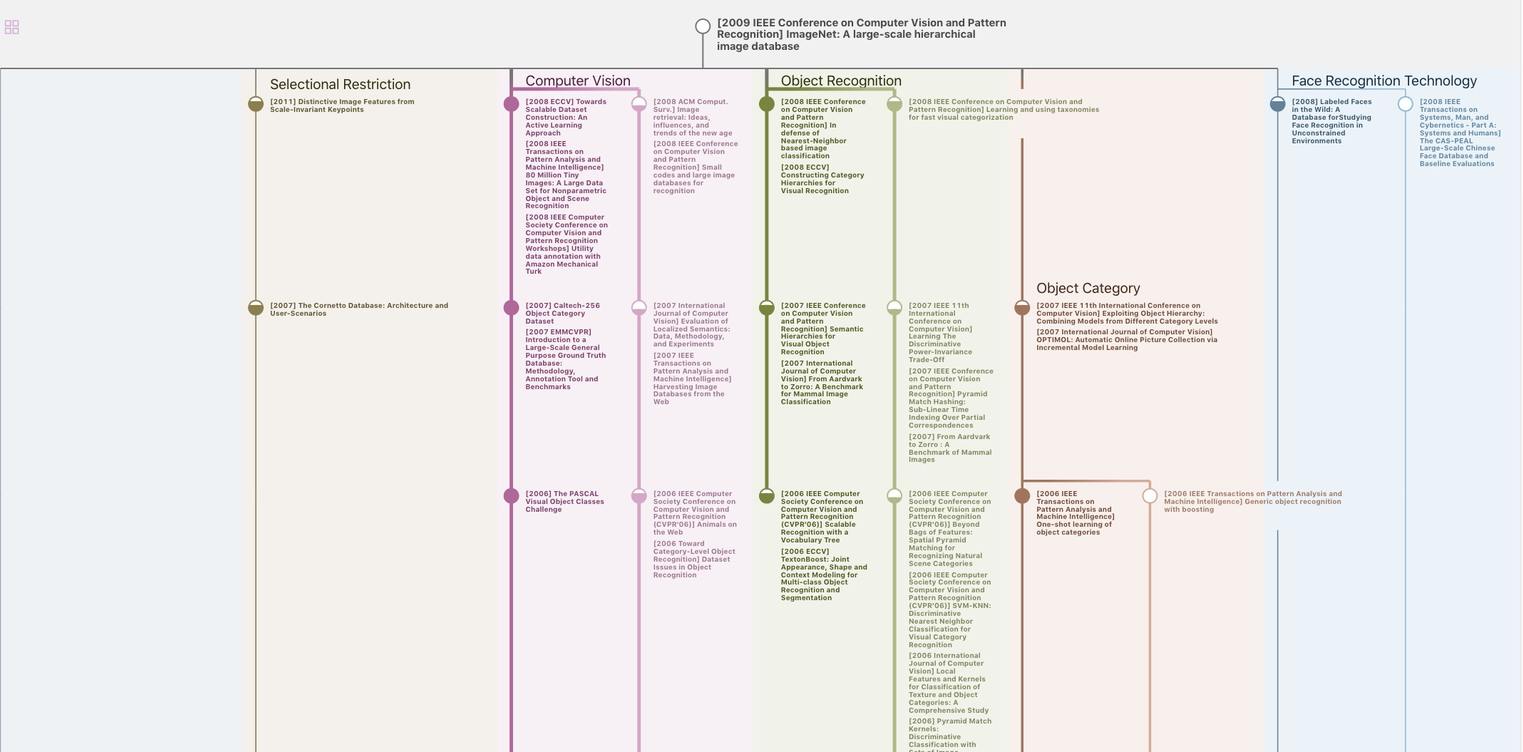
生成溯源树,研究论文发展脉络
Chat Paper
正在生成论文摘要