LSTM-based radiography for anomaly detection in softwarized infrastructures
2020 32nd International Teletraffic Congress (ITC 32)(2020)
摘要
Legacy and novel network services are expected to be migrated and designed to be deployed in fully virtualized environments. Starting with 5G, NFV becomes a formally required brick in the specifications, for services integrated within the infrastructure provider networks. This evolution leads to deployment of virtual resources Virtual-Machine (VM)-based, container-based and/or server-less platforms, all calling for a deep virtualization of infrastructure components. Such a network softwarization also unleashes further logical network virtualization, easing multi-layered, multi-actor and multi-access services, so as to be able to fulfill high availability, security, privacy and resilience requirements. However, the derived increased components heterogeneity makes the detection and the characterization of anomalies difficult, hence the relationship between anomaly detection and corresponding reconfiguration of the NFV stack to mitigate anomalies. In this article we propose an unsupervised machine-learning data-driven approach based on Long-Short- Term-Memory (LSTM) autoencoders to detect and characterize anomalies in virtualized networking services. With a radiography visualization, this approach can spot and describe deviations from nominal parameter values of any virtualized network service by means of a lightweight and iterative mean-squared reconstruction error analysis of LSTM-based autoencoders. We implement and validate the proposed methodology through experimental tests on a vIMS proof-of-concept deployed using Kubernetes.
更多查看译文
关键词
Machine Learning, Anomaly detection, SDN, NFV, Orchestration
AI 理解论文
溯源树
样例
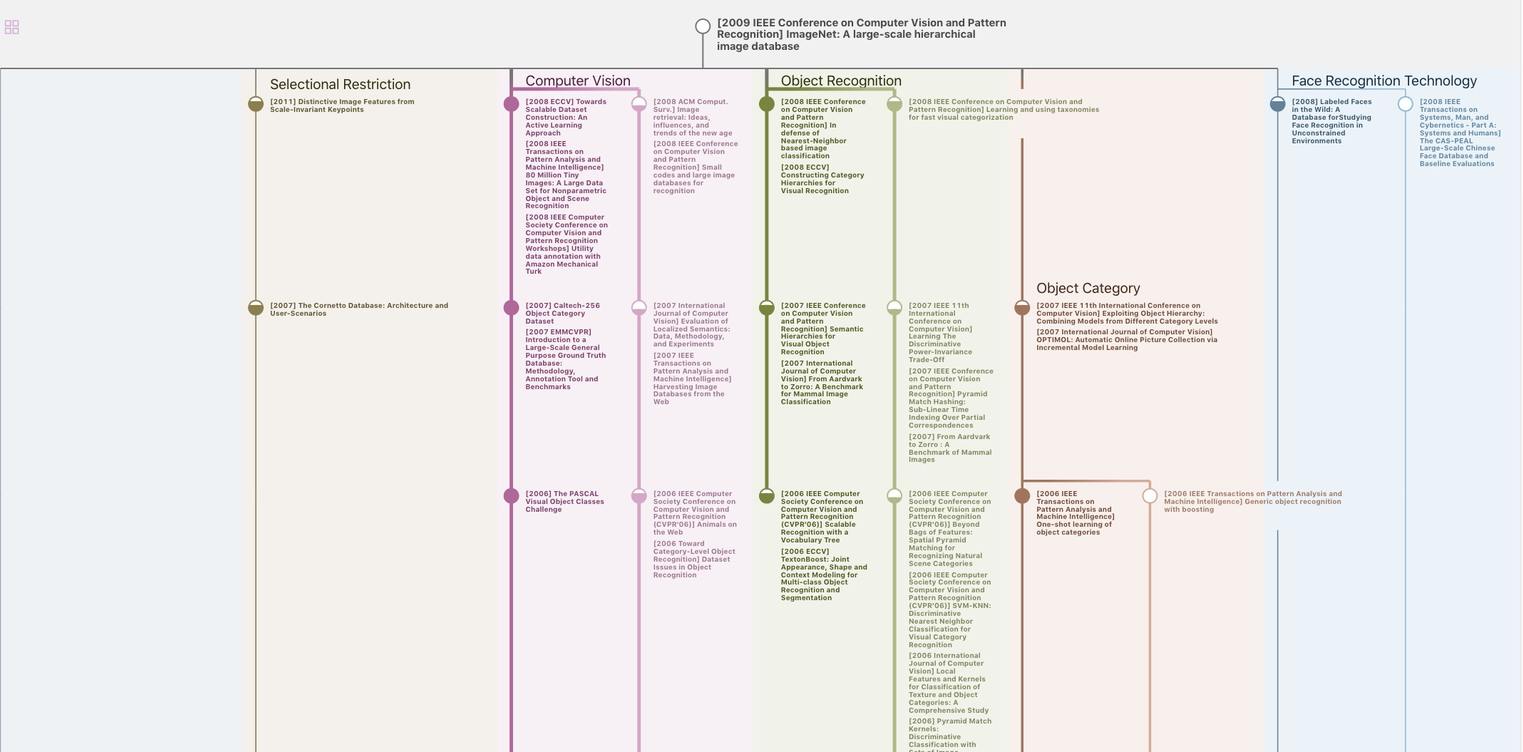
生成溯源树,研究论文发展脉络
Chat Paper
正在生成论文摘要