Modelling Stock Markets by Multi-agent Reinforcement Learning
COMPUTATIONAL ECONOMICS(2020)
摘要
Quantitative finance has had a long tradition of a bottom-up approach to complex systems inference via multi-agent systems (MAS). These statistical tools are based on modelling agents trading via a centralised order book, in order to emulate complex and diverse market phenomena. These past financial models have all relied on so-called zero-intelligence agents, so that the crucial issues of agent information and learning, central to price formation and hence to all market activity, could not be properly assessed. In order to address this, we designed a next-generation MAS stock market simulator, in which each agent learns to trade autonomously via reinforcement learning. We calibrate the model to real market data from the London Stock Exchange over the years 2007 to 2018, and show that it can faithfully reproduce key market microstructure metrics, such as various price autocorrelation scalars over multiple time intervals. Agent learning thus enables accurate emulation of the market microstructure as an emergent property of the MAS.
更多查看译文
关键词
Agent-based,Reinforcement learning,Multi-agent system,Stock markets
AI 理解论文
溯源树
样例
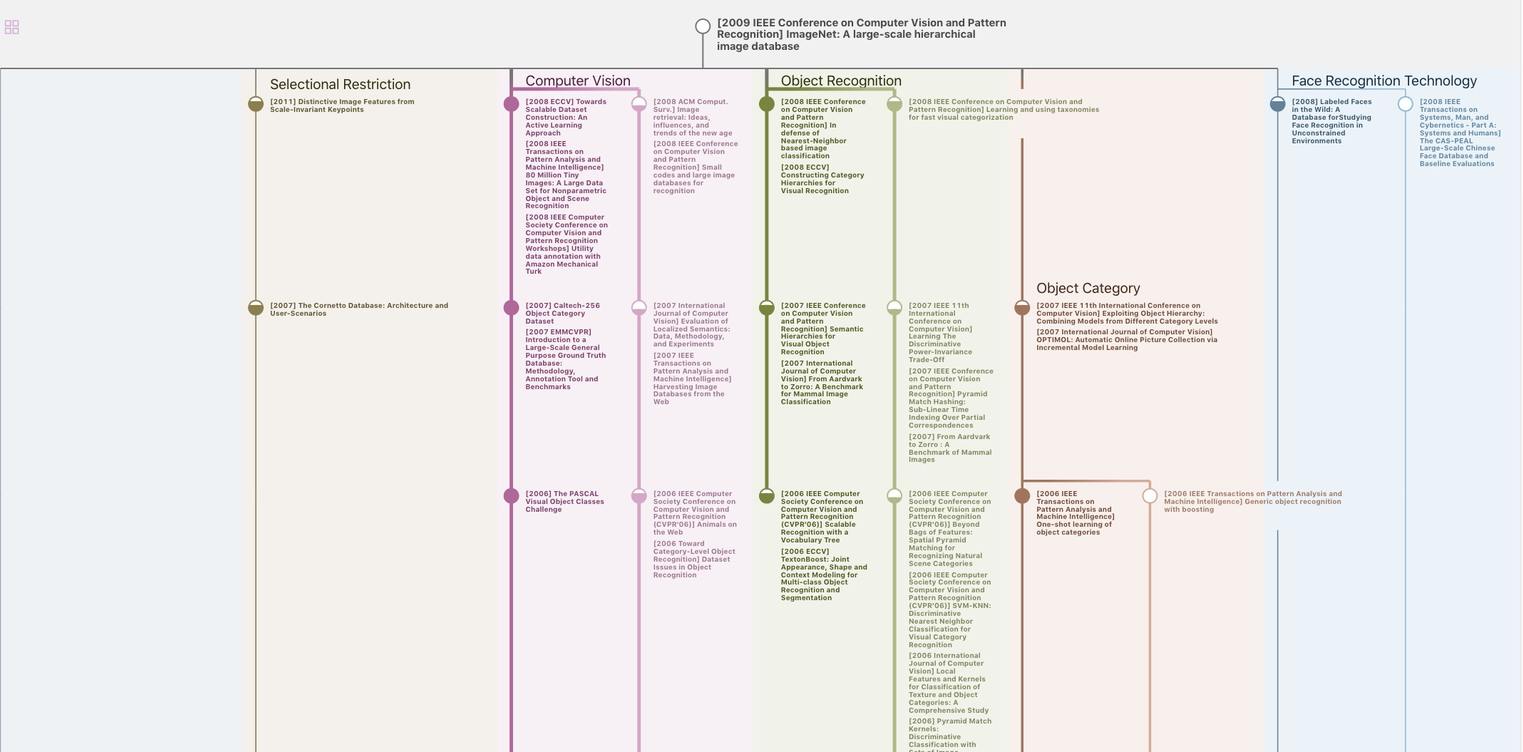
生成溯源树,研究论文发展脉络
Chat Paper
正在生成论文摘要