Dilated-DenseNet For Macromolecule Classification In Cryo-electron Tomography.
ISBRA(2020)
摘要
Cryo-electron tomography (cryo-ET) combined with subtomogram averaging (STA) is a unique technique in revealing macromolecule structures in their near-native state. However, due to the macromolecular structural heterogeneity, low signal-to-noise-ratio (SNR) and anisotropic resolution in the tomogram, macromolecule classification, a critical step of STA, remains a great challenge. In this paper, we propose a novel convolution neural network, named 3D-Dilated-DenseNet, to improve the performance of macromolecule classification in STA. The proposed 3D-Dilated-DenseNet is challenged by the synthetic dataset in the SHREC contest and the experimental dataset, and compared with the SHREC-CNN (the state-of-the-art CNN model in the SHREC contest) and the baseline 3D-DenseNet. The results showed that 3D-Dilated-DenseNet significantly outperformed 3D-DenseNet but 3D-DenseNet is well above SHREC-CNN. Moreover, in order to further demonstrate the validity of dilated convolution in the classification task, we visualized the feature map of 3D-Dilated-DenseNet and 3D-DenseNet. Dilated convolution extracts a much more representative feature map.
更多查看译文
关键词
Convolutional Neural Network,Cryo-electron Tomography,Object Classification,Subtomogram Averaging
AI 理解论文
溯源树
样例
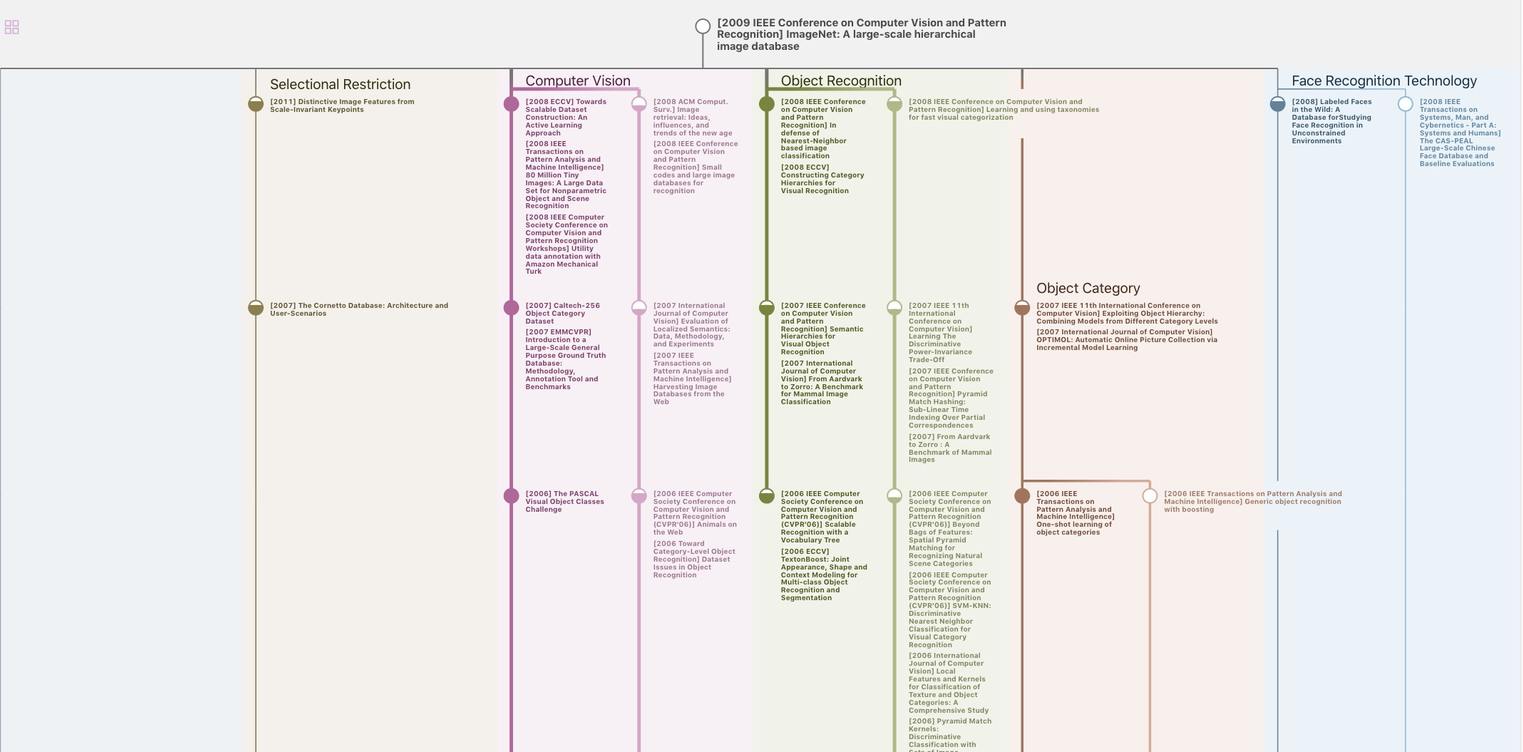
生成溯源树,研究论文发展脉络
Chat Paper
正在生成论文摘要