A Heterogeneous Ensemble Credit Scoring Model Based On Adaptive Classifier Selection: An Application On Imbalanced Data
INTERNATIONAL JOURNAL OF FINANCE & ECONOMICS(2021)
摘要
In the domain of credit scoring, the number of bad clients is far less than that of good ones. So imbalanced data classification is a realisitc and critical issue in the credit scoring process. In this study, a novel heterogeneous ensemble credit scoring model is proposed for the problem of imbalanced data classification. This proposed model is on basis of five standard classifiers, namely LSVM, KNN, MDA, DT, LR, and adaptively selects the base classifiers with highest AUC according to the data distribution, then integrates all base classifiers to obtain a prediction. Finally, by using five comprehensive performance measures and four classical credit datasets, we find that the proposed model is better than other baseline models. This novel model can be applied to actual credit scoring and assist financial institutions in credit risk management.
更多查看译文
关键词
binary classification, credit scoring, ensemble model, heterogeneous ensemble, imbalanced data
AI 理解论文
溯源树
样例
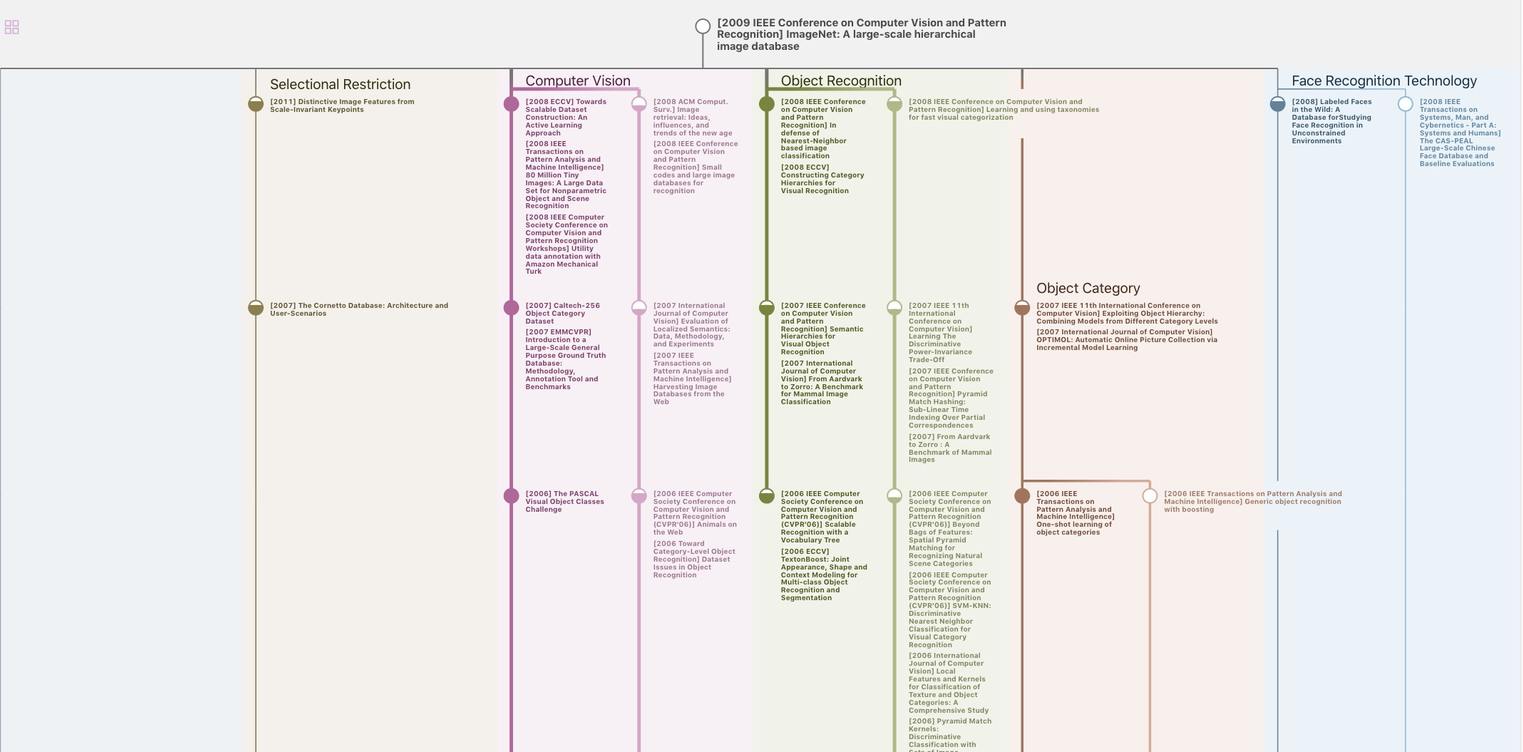
生成溯源树,研究论文发展脉络
Chat Paper
正在生成论文摘要