Parameter Self-Tuning of SISO Compact-Form Model-Free Adaptive Controller Based on Long Short-Term Memory Neural Network
IEEE ACCESS(2020)
摘要
Model-free adaptive controller (MFAC) is a novel data-driven control methodology that relies only on input/output (I/O) measurement data instead of classic mathematical models of actual controlled plants. The single-input single-output (SISO) compact-form MFAC (SISO-CFMFAC) is a promising method for controlling SISO nonlinear time-varying systems. The parameters in SISO-CFMFAC must be carefully tuned before use, as inappropriate parameters may lead to poor control performance. However, up to now, parameter tuning has been a time-consuming and laborious task. In this paper, a new approach called SISO-CFMFAC-LSTM is proposed for parameter self-tuning of SISO-CFMFAC based on long short-term memory (LSTM) neural network. To evaluate the performance of the proposed methodology, qualitative and quantitative comparisons with other existing control algorithms are carried out. Six individual performance indices, namely, the root mean square error (RMSE), the integral absolute error (IAE), the integral time-weighted absolute error (ITAE), the integral absolute variation of the control signal (IAVU), the maximum overshoot (MO), and the imprecise control ratio (ICR), are introduced for quantitative comparison. The experimental results demonstrate that the proposed SISO-CFMFAC-LSTM achieves the best performance in all indices, indicating that it is an effective control method for SISO nonlinear time-varying systems.
更多查看译文
关键词
LSTM neural network,model-free adaptive controller,parameter self-tuning,three-tank system
AI 理解论文
溯源树
样例
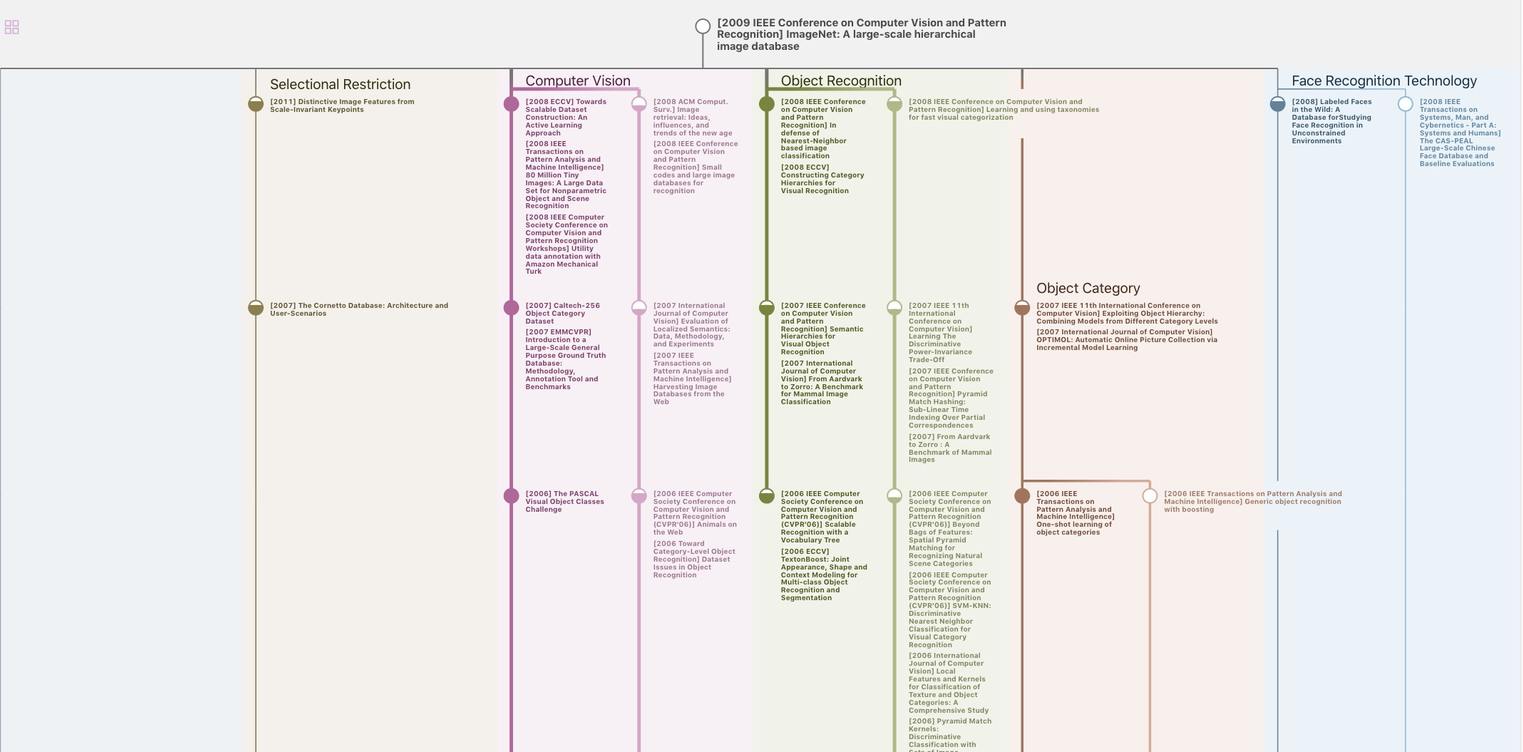
生成溯源树,研究论文发展脉络
Chat Paper
正在生成论文摘要