MSANet: A Multi-Scale Attention Module
ISKE(2019)
摘要
Multi-scale representation ability is one of key criteria for measuring convolutional neural networks (CNNs) effectiveness. Recent studies have shown that multi-scale features can represent different semantic information of original images, and a combination of them would have positive influence on vision tasks. Many researchers are investigated in extract the multi-scale features in a layerwise manner and equipped with relatively inflexible receptive field. In this paper, we propose a multi-scale attention (MSA) module for CNNs, namely MSANet, where the residual block comprises hierarchical attention connections and skip connections. The MSANet improves the multi-scale representation power of the network by adaptively enriching the receptive fields of each convolutional branch. We insert the proposed MSANet block into several backbone CNN models and achieve consistent improvement over backbone models on CIFAR-100 dataset. To better verify the effectiveness of MSANet, the experimental results on major network details, i.e., scale, depth, further demonstrate the superiority of the MSANet over the Res2Net methods.
更多查看译文
关键词
multi-scale,attention
AI 理解论文
溯源树
样例
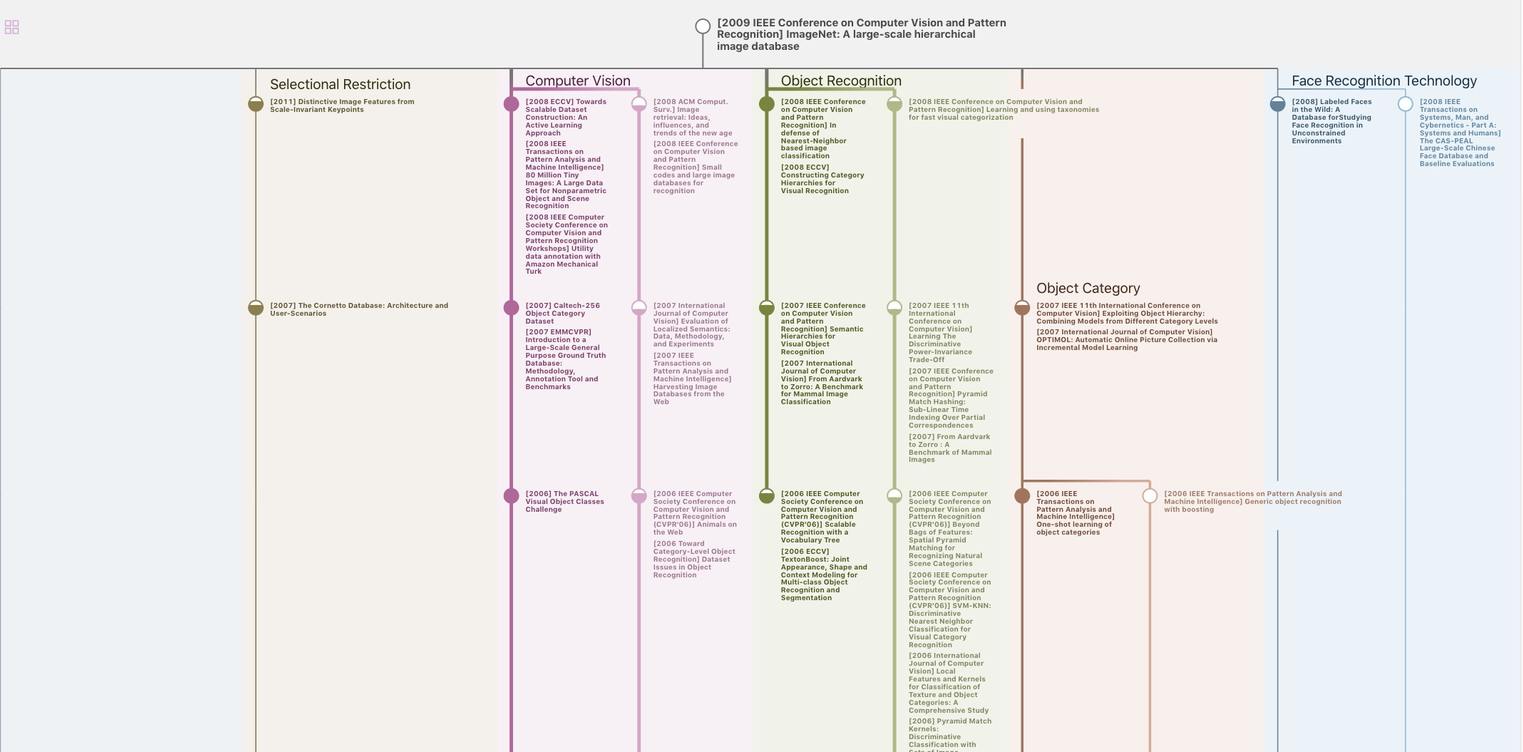
生成溯源树,研究论文发展脉络
Chat Paper
正在生成论文摘要