Proactive Quality Control: Observing System Experiments Using The Ncep Global Forecast System
MONTHLY WEATHER REVIEW(2020)
摘要
Proactive quality control (PQC) is a fully flow dependent QC based on ensemble forecast sensitivity to observations (EFSO). Past studies showed in several independent cases that GFS forecasts can be improved by rejecting observations identified as detrimental by EFSO. However, the impact of cycling PQC in sequential data assimilation has, so far, only been examined using the simple Lorenz '96 model. Using a low-resolution spectral GFS model that assimilates PrepBUFR (no radiance) observations with the local ensemble transform Kalman filter (LETKF), this study aims to become a bridge between a simple model and the implementation into complex operational systems. We demonstrate the major benefit of cycling PQC in a sequential data assimilation framework through the accumulation of improvements from previous PQC updates. Such accumulated PQC improvement is much larger than the "current'' PQC improvement that would be obtained at each analysis cycle using "future'' observations. As a result, it is unnecessary to use future information, and hence this allows the operational implementation of cycling PQC. The results show that the analyses and forecasts are improved the most by rejecting all the observations identified as detrimental by EFSO, but that major improvements also come from rejecting just the most detrimental 10% observations. The forecast improvements brought by PQC are observed throughout the 10 days of integration and provide more than a 12-h forecast lead-time gain. An important finding is that PQC not only reduces substantially the root-mean-squared forecast errors but also the forecast biases. We also show a case of "skill dropout,'' where the control forecast misses a developing baroclinic instability, whereas the accumulated PQC corrections result in a good prediction.
更多查看译文
AI 理解论文
溯源树
样例
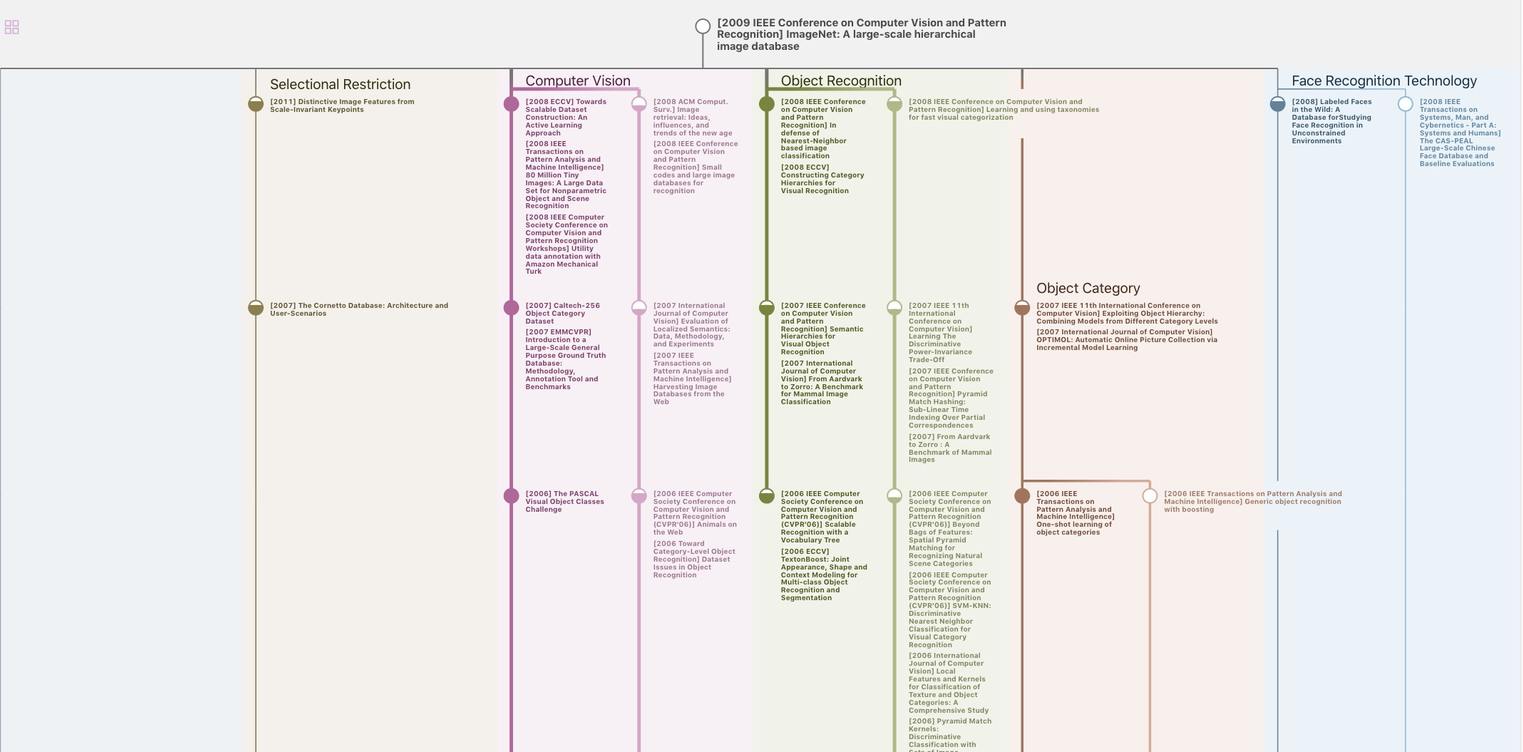
生成溯源树,研究论文发展脉络
Chat Paper
正在生成论文摘要