Shallow water bathymetry with multi-spectral satellite ocean color sensors: Leveraging temporal variation in image data
REMOTE SENSING OF ENVIRONMENT(2020)
摘要
Polar-orbiting ocean color satellites such as Landsat-8, Suomi National Polar-orbiting Partnership (SNPP), and Sentinel-3 offer valuable image data for the derivation of water bathymetry in optically shallow environments. Because of the multi-spectral limitation, however, it is challenging to derive bathymetry over global shallow waters without reliable mechanistic algorithms. In this contribution, we present and test a physics-based algo-rithm for improved retrieval of bathymetry with multi-spectral sensors. The algorithm leverages the temporal variation of water-column optical properties in two satellite measurements. By incorporating two remote sensing reflectance spectra in an optimization procedure, it enhances the spectral constraining condition for the optimization, thus leading to improved retrieval accuracy. This scheme is evaluated using synthetic multi-spectral data. It is shown that the new approach can provide accurate estimation of water depths over 0-30 m range with three types of benthic substrates (corals, seagrass, and sand) and for a wide range of water column optical properties. Based on the degree of improvement, Landsat-8 appears to be benefited the most, followed by SNPP, and then Sentinel-3. The application of the new approach is demonstrated with satellite images over shallow waters (0-30 m) dominated with coral reefs, seagrass, and sand, respectively. This proof-of-concept study confirms the promise of multi-spectral satellite sensors for accurate water depth retrieval by accounting for the temporal characteristics in multiple measurements, suggesting a path forward for the derivation of bathymetry from the existing satellites over global shallow waters.
更多查看译文
关键词
Shallow water,Bathymetry,Spectral optimization,Remote sensing reflectance,Temporal variation,Landsat-8,SNPP,Sentinel-3
AI 理解论文
溯源树
样例
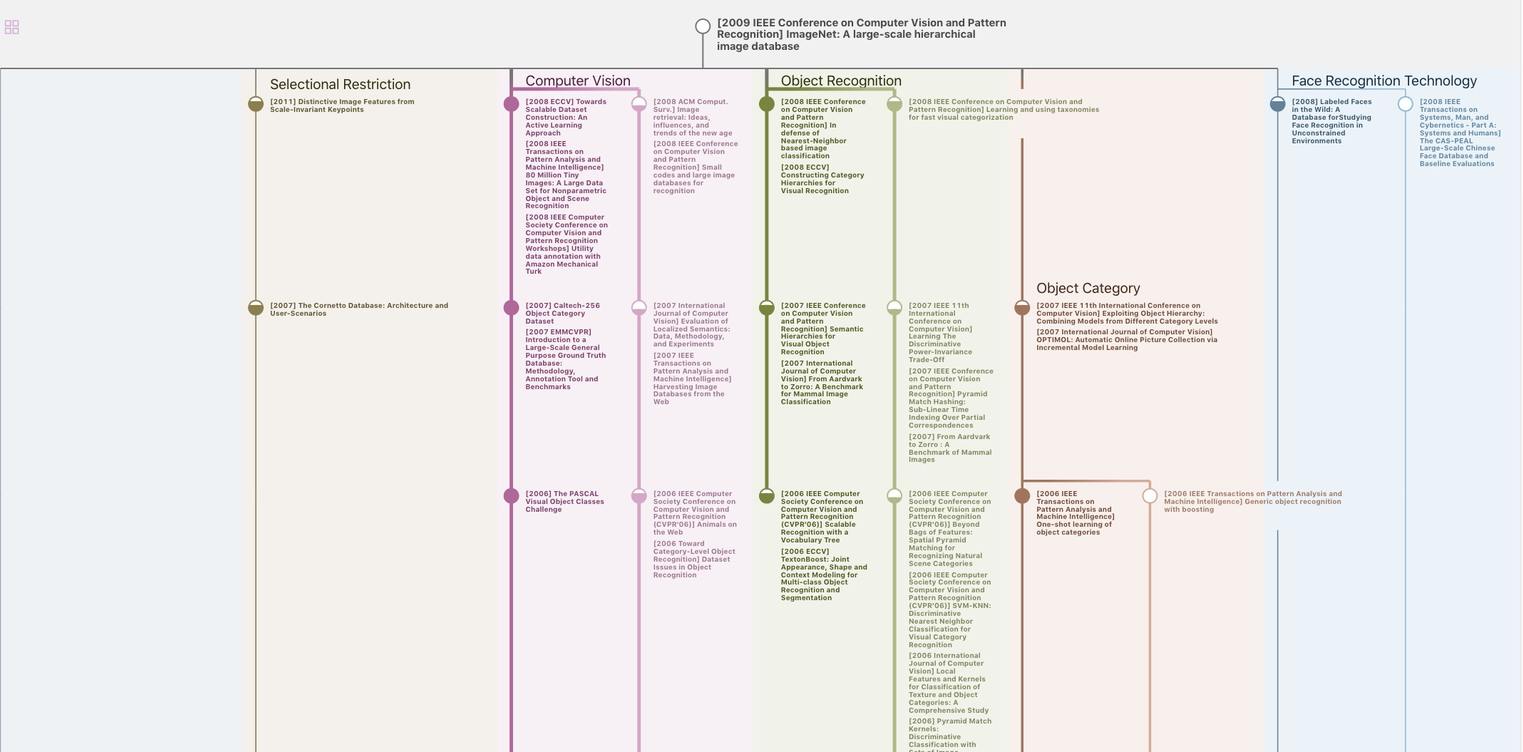
生成溯源树,研究论文发展脉络
Chat Paper
正在生成论文摘要