Application of Parameter Screening to Derive Optimal Initial State Adjustments for Streamflow Forecasting
WATER RESOURCES RESEARCH(2020)
摘要
Streamflow forecasting is essential in many applications, including the management of hydropower reservoirs and of flood risks. To increase the performance of these hydrologic forecasts, a hydrological model is typically updated with optimal initial model states. These states are usually selected a priori and adjusted to obtain the optimal initial setup. The model states to adjust are generally selected by experts with deep knowledge of the model's behavior. These relationships are rarely documented and formalized, leading to difficulties in reproducibility and transferability to other models. The method here provides a fully automatized system to find the model states based on the parameter-free sensitivity method of efficient elementary effects (EEE), which identifies the most informative variables of the hydrologic model CEQUEAU. The analysis is applied to 1,826 model setups for four Canadian basins. The identified states are formalized using clustering techniques to obtain adjustment "recipes" conditioned on given meteorological conditions. Several recipes are tested and compared to expert-based recipes. The recipes are tested according to their ability to (a) obtain optimal initial states and (b) their forecast performance. The results show that (1) the EEE-based recipes perform similarly or better than the expert-based recipes in both objectives; (2) the recipes are similar across the four basins, highlighting their potential of spatial transferability; and (3) the adjustment of hydrologic model inputs significantly decreases forecast performance. The proposed approach to use EEE-based recipes to obtain optimal initial states for forecasting is reproducible, objective, and suitable to be applied to other models and basins.
更多查看译文
关键词
data assimilation,sensitivity analysis,streamflow forecasting
AI 理解论文
溯源树
样例
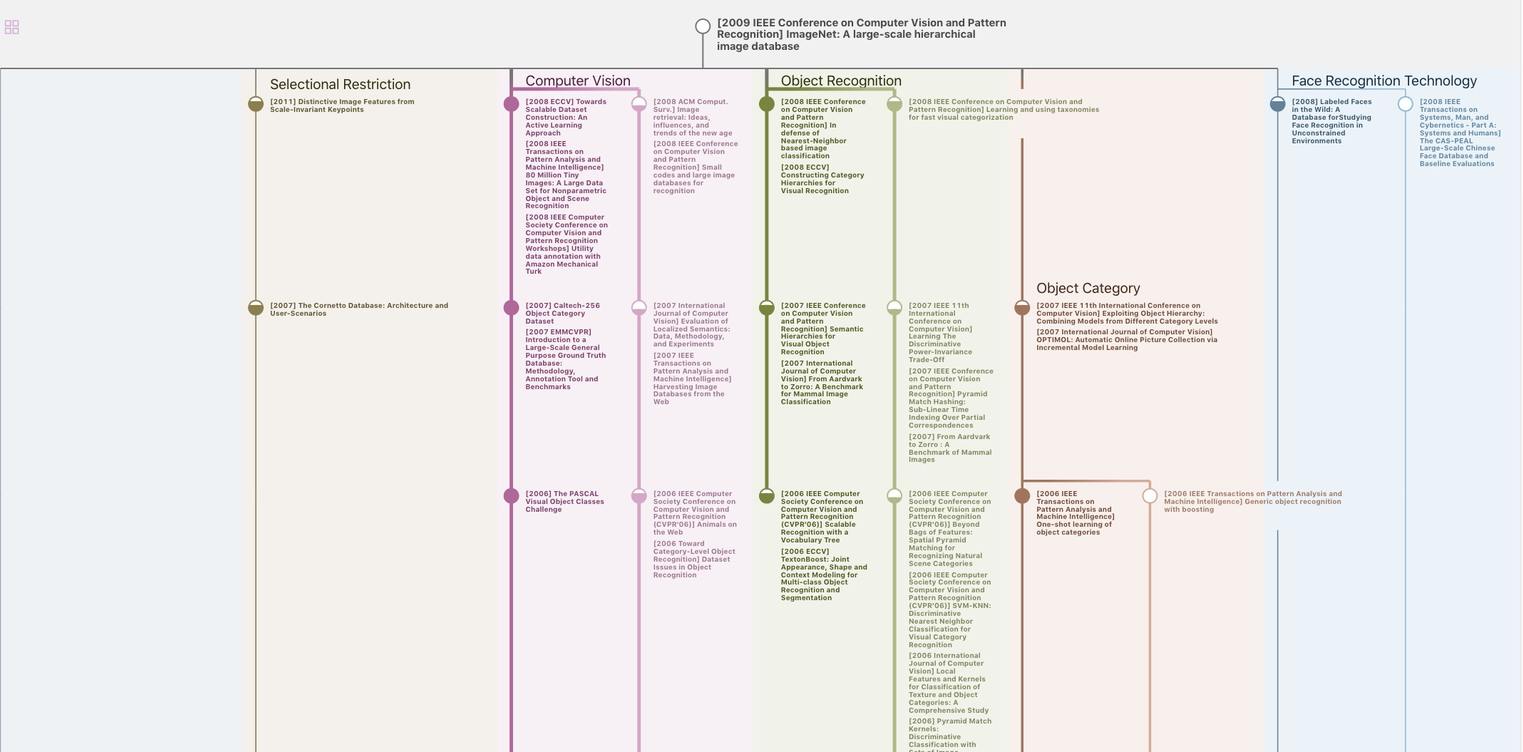
生成溯源树,研究论文发展脉络
Chat Paper
正在生成论文摘要