On the Variety and Veracity of Cyber Intrusion Alerts Synthesized by Generative Adversarial Networks
ACM Transactions on Management Information Systems(2020)
摘要
AbstractMany cyber attack actions can be observed, but the observables often exhibit intricate feature dependencies, non-homogeneity, and potentially rare yet critical samples. This work tests the ability to learn, model, and synthesize cyber intrusion alerts through Generative Adversarial Networks (GANs), which explore the feature space by reconciling between randomly generated samples and data that reflect a mixture of diverse attack behaviors without a priori knowledge. Through a comprehensive analysis using Jensen-Shannon Divergence, Conditional and Joint Entropy, and mode drops and additions, we show that the Wasserstein-GAN with Gradient Penalty and Mutual Information is more effective in learning to generate realistic alerts than models without Mutual Information constraints. We further show that the added Mutual Information constraint pushes the model to explore the feature space more thoroughly and increases the generation of low probability, yet critical, alert features. This research demonstrates the novel and promising application of unsupervised GANs to learn from limited yet diverse intrusion alerts to generate synthetic alerts that emulate critical dependencies, opening the door to proactive, data-driven cyber threat analyses.
更多查看译文
关键词
GAN, intrusion alert analysis, cyberattack characterization
AI 理解论文
溯源树
样例
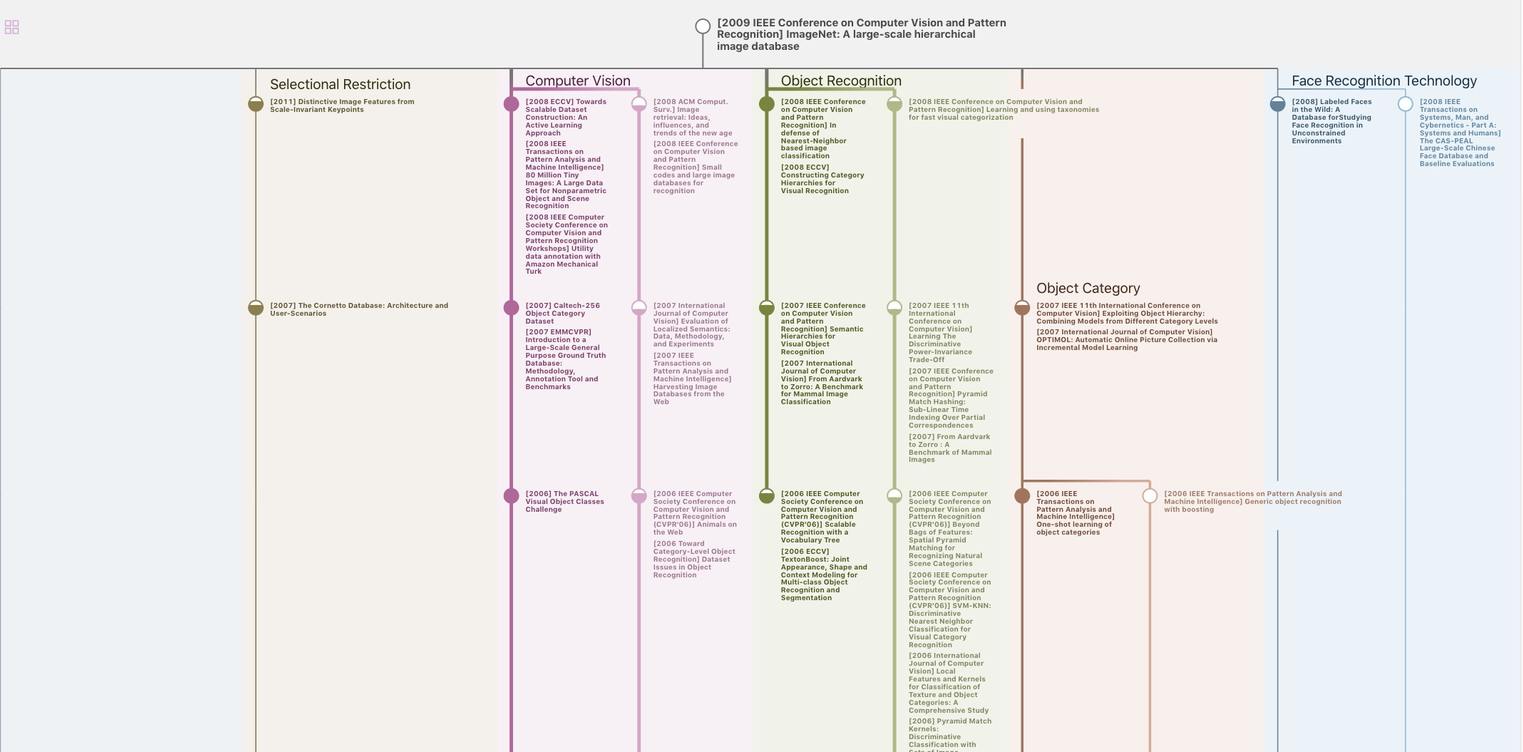
生成溯源树,研究论文发展脉络
Chat Paper
正在生成论文摘要