DeepSplit - Segmentation of Microscopy Images Using Multi-task Convolutional Networks.
MIUA(2020)
摘要
Accurate segmentation of cellular structures is critical for automating the analysis of microscopy data. Advances in deep learning have facilitated extensive improvements in semantic image segmentation. In particular, U-Net, a model specifically developed for biomedical image data, performs multi-instance segmentation through pixel-based classification. However, approaches based on U-Net tend to merge touching cells in dense cell cultures, resulting in under-segmentation. To address this issue, we propose DeepSplit; a multi-task convolutional neural network architecture where one encoding path splits into two decoding branches. DeepSplit first learns segmentation masks, then explicitly learns the more challenging cell-cell contact regions. We test our approach on a challenging dataset of cells that are highly variable in terms of shape and intensity. DeepSplit achieves 90% cell detection coefficient and 90% Dice Similarity Coefficient (DSC) which is a significant improvement on the state-of-the-art U-Net that scored 70% and 84% respectively.
更多查看译文
关键词
microscopy images,segmentation,networks,multi-task
AI 理解论文
溯源树
样例
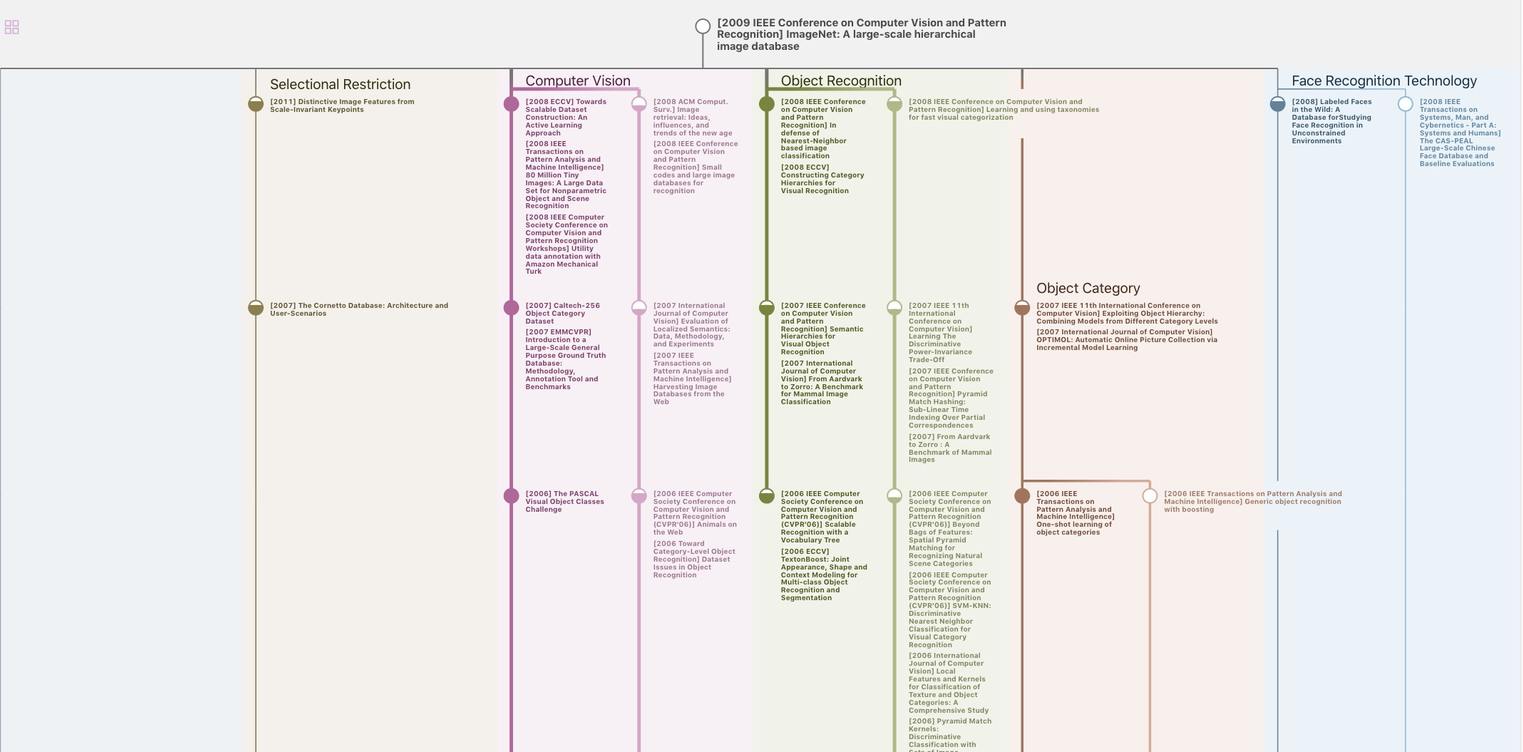
生成溯源树,研究论文发展脉络
Chat Paper
正在生成论文摘要