Privacy-Preserving Collaborative Learning for Multiarmed Bandits in IoT
IEEE Internet of Things Journal(2021)
摘要
This article studies privacy-preserving collaborative learning in decentralized Internet-of-Things (IoT) networks, where the agents exchange information constantly to improve the learnability, and meanwhile make the privacy of agents protected during communications. However, the harsh constraints in IoT make executing collaborative learning much more difficult than well-connected systems composed by servers with strong computation power, due to the weak capacity of devices, limited bandwidth for exchanging information, the asynchronous communication environment, and the necessity of privacy preserving. We show that even if with the harsh constraints in IoT, it still can devise efficient privacy-preserving collaborative learning algorithms, by proposing the first known decentralized collaborative learning algorithm for the fundamental multiarmed bandits problem under the framework of local differential privacy. Rigorous analysis shows that the proposed learning algorithm can make every agent learn the best arm with a high probability and keep the privacy preserved meanwhile. Extensive experiments illustrate that our learning algorithm performs well in real settings.
更多查看译文
关键词
Collaborative learning,local differential privacy (LDP),multiarmed bandits (MAB),privacy preserving
AI 理解论文
溯源树
样例
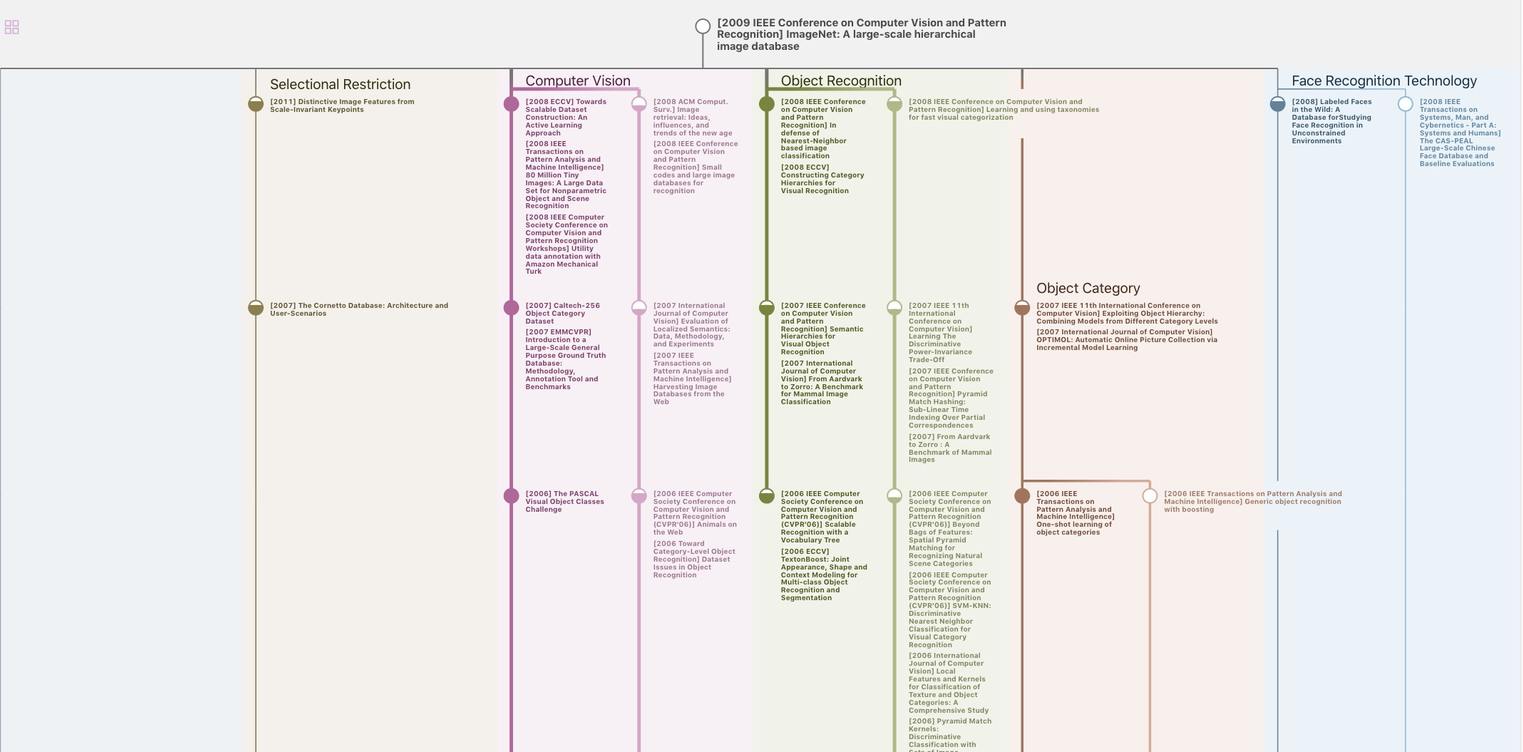
生成溯源树,研究论文发展脉络
Chat Paper
正在生成论文摘要