Workload-aware wavelet synopses for sliding window aggregates
DISTRIBUTED AND PARALLEL DATABASES(2020)
摘要
In this work, we study the problem of maintaining basic aggregate statistics over a sliding-window data stream under the constraint of limited memory. As in IoT scenarios the available memory is typically much less than the window size, queries are answered from compact synopses that are maintained in an online fashion. For the efficient construction of such synopses, we propose wavelet-based algorithms that provide deterministic guarantees and produce near exact results for a variety of data distributions. Furthermore, we show how accuracy can be further improved when workload information is known. For this purpose, we propose a workload-aware streaming system that trade-offs accuracy with synopsis’ construction throughput. The conducted experiments indicate that with only a 15% penalty in throughput, the proposed system produces fairly accurate results even for the most adversarial distributions.
更多查看译文
关键词
Stream processing, Sliding-window, Approximate query processing, Wavelets, Workload-aware synopses, Range queries
AI 理解论文
溯源树
样例
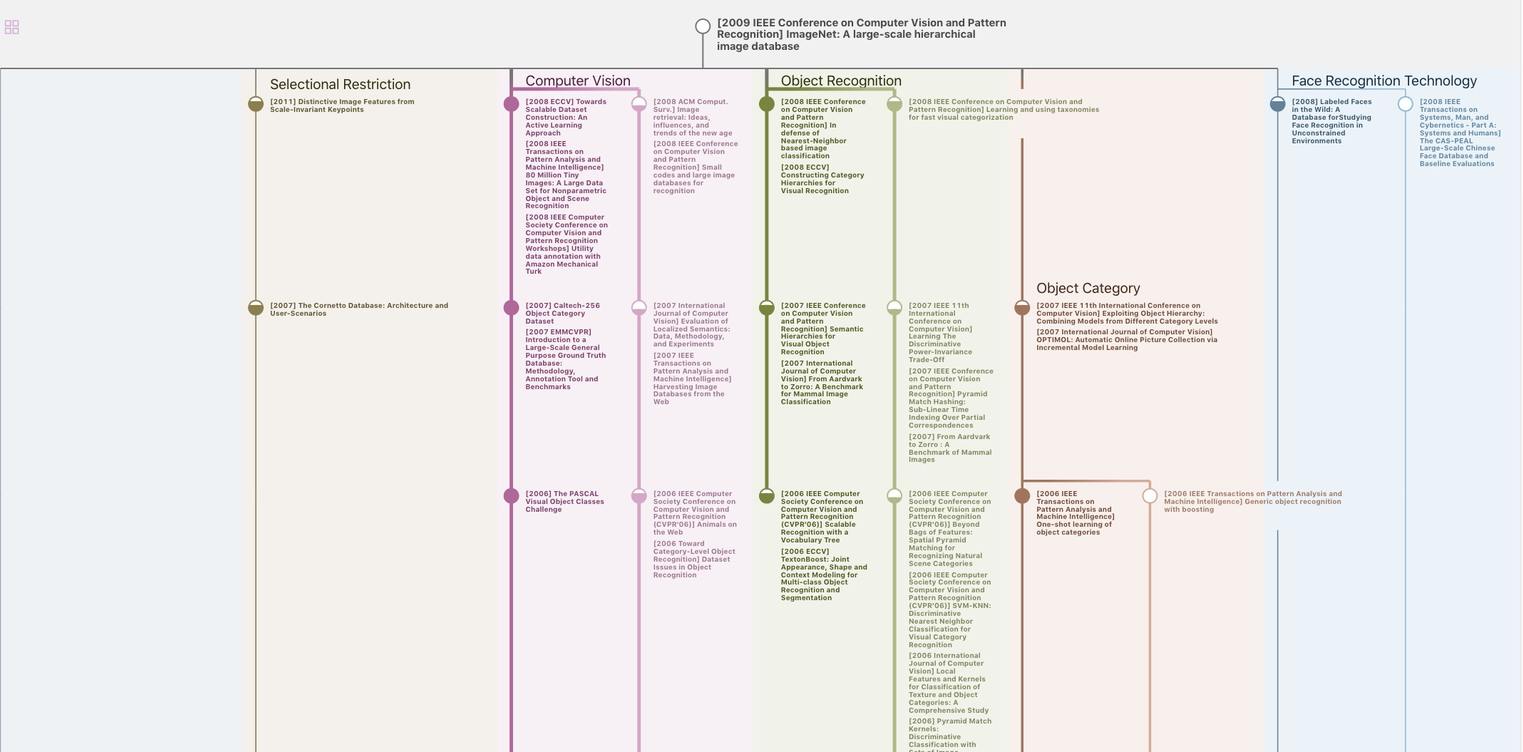
生成溯源树,研究论文发展脉络
Chat Paper
正在生成论文摘要