Image Translation With Attention Mechanism Based On Generative Adversarial Networks
IEEE INFOCOM 2020 - IEEE CONFERENCE ON COMPUTER COMMUNICATIONS WORKSHOPS (INFOCOM WKSHPS)(2020)
摘要
In this paper, we present a novel approach for image translation based on Generative Adversarial Networks (GANs). We apply the self-attention mechanism to improve the quality of the generated images as well as focus on not only local feature representation but also global structural correlation. Meanwhile, we adopt the idea of cyclic image translation in CycleGAN. Moreover, in order to stabilize the training process and reduce the probability of appearing unusual gradients, two techniques spectral normalization and TTUR applied during training process. In the experimental section, we compare our method with the original CycleGAN in terms of subjective evaluation, objective scoring, classification verification and computational cost. Both subjective and objective results show that our model can generate high quality and abundant diversity images that uses unpaired unlabeled samples. Two techniques used during the training have prompt the convergence speed of the network and help the model use less time to get the optimal point. Lots of comparisons against CycleGAN demonstrate that our proposed method is superior to the original one.
更多查看译文
关键词
generative adversarial network, image translation, self attention
AI 理解论文
溯源树
样例
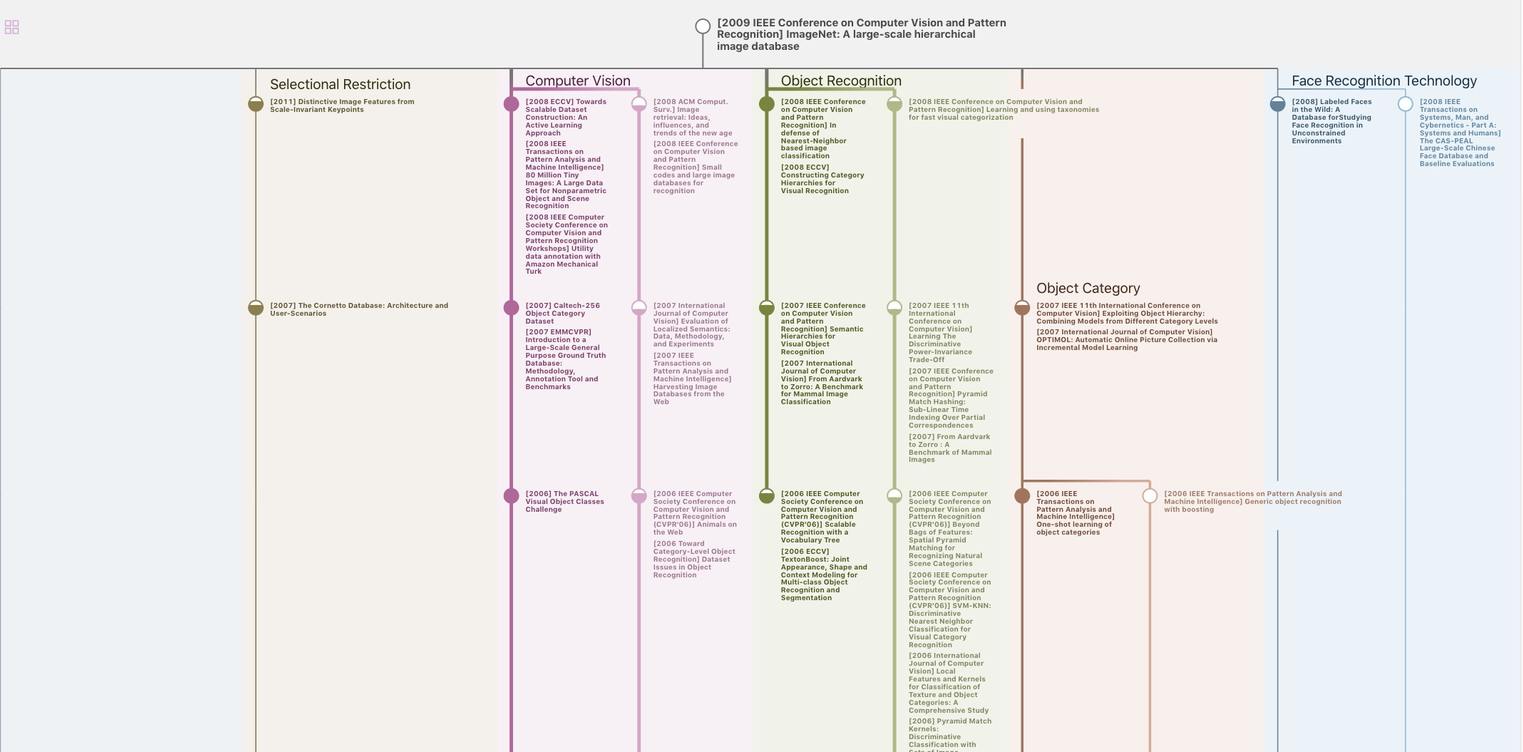
生成溯源树,研究论文发展脉络
Chat Paper
正在生成论文摘要