Optimal Long Short Term Memory Networks For Long-Term Forecasting Of Real Wind Characteristics
IFAC PAPERSONLINE(2020)
摘要
The capability of wind power to meet the energy demand inspired researchers to develop techniques for harnessing this clean and renewable energy. As a primary step, accurate forecasting of wind characteristics by modelling the stochastic nature of wind is done. Although, statistical methods provide good results in forecasting, they are inferior to Deep Learning based tools while handling extreme nonlinearities in wind characteristics. The authors in this work implemented Long Short Term Memory (LSTM) networks, a deep learning based tool, for modelling wind time series data due to its efficiency in handling long term dependencies. However, several hyper-parameters like activation function and design are chosen heuristically, making the modelling process tedious and inefficient. In this study, novel multi objective optimization formulation, driven by NSGA II, is proposed to design the LSTM networks with respect to the conflicting objectives of accuracy and parsimony. The resultant optimal LSTM models are used for long term forecasting (2 years) of wind characteristics data, with an accuracy of 97%, obtained from a real wind farm in France. To demonstrate the importance of this forecasting, a study of wind power calculations on a real wind farm is conducted. For a given layout, the effect of wind frequency scenarios, generated from the time series data of wind, on the annual power calculations, is determined. The existing and forecasted values of wind speed and direction over longer periods of time resulted in realistic values of expected power from wind farm. This study demonstrates the importance of forecasting while evaluating the power which can impact research in fields such as wind farm layout optimization and control under uncertainty. (C) 2020, IFAC (International Federation of Automatic Control) Hosting by Elsevier Ltd. All rights reserved.
更多查看译文
关键词
Wind Characteristics Forecasting, Deep Learning, LSTM, Evolutionary optimization
AI 理解论文
溯源树
样例
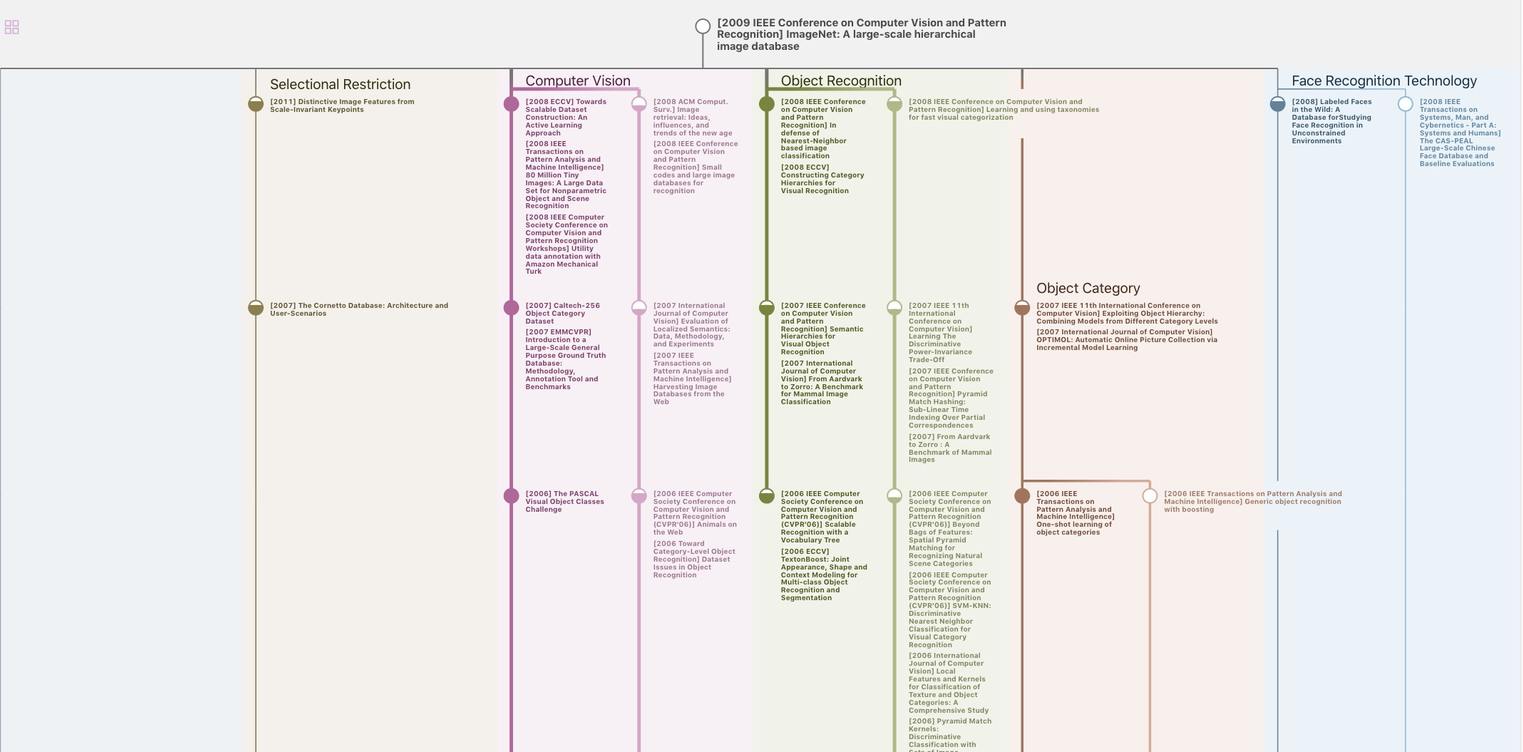
生成溯源树,研究论文发展脉络
Chat Paper
正在生成论文摘要