Multinoise-Type Blind Denoising Using A Single Uniform Deep Convolutional Neural Network
JOURNAL OF ELECTRONIC IMAGING(2020)
摘要
Deep convolutional neural networks (CNNs) have achieved considerable success with image denoising. However, they still lack consistent performance across different noise types and levels. We extend noise scenarios to four categories: Gaussian, random-impulse, salt-and-pepper, and Poisson. We also propose a multinoise-type blind denoising network (MBDNet) that solves the blind denoising task using a uniform deep CNN architecture. The network can be divided into two stages where a concise CNN is first used to estimate auxiliary noise-type and noise-level information. Estimation results are then integrated as additional channels of the noisy image and are fed to the subsequent denoising stage. A unique two-branch structure is further adopted in the residual denoising CNN, wherein a shallow branch predicts the filter-flow mask and adaptively adjusts the feature extraction of the parallel deep branch. Extensive experiments on synthetic noisy images validate the effectiveness of the noise-estimation and denoising subnetworks and show that MBDNet is highly competitive as compared to state-of-the-art methods in both denoising performance and model runtime. (C) 2020 SPIE and IS&T
更多查看译文
关键词
image denoising, convolutional neural networks, blind denoising, noise estimation
AI 理解论文
溯源树
样例
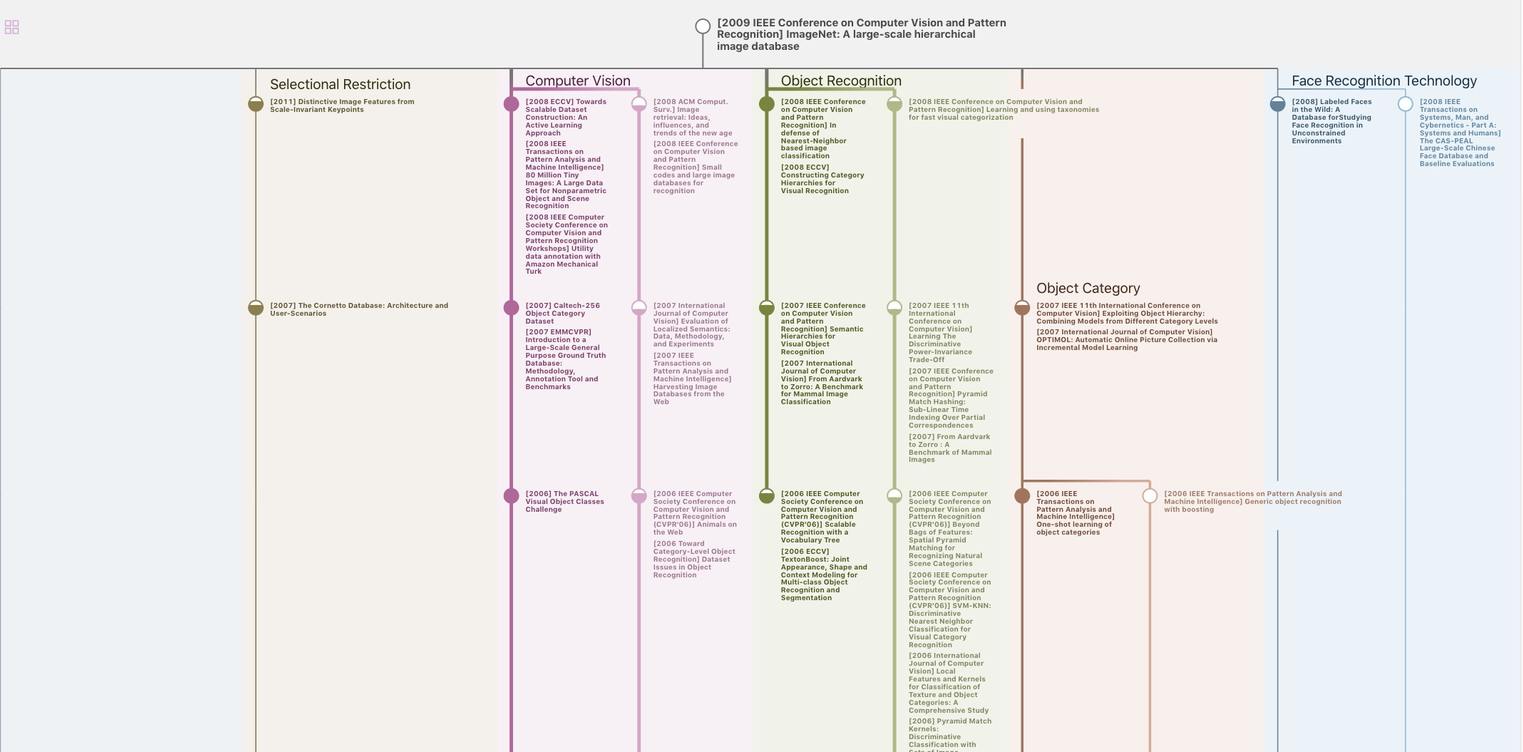
生成溯源树,研究论文发展脉络
Chat Paper
正在生成论文摘要