Machine-learning models for detection of cellular states of human body using bio-impedance spectroscopy
Sensing and Bio-Sensing Research(2024)
摘要
Assessment of cellular states of body is essential to ascertain physical fitness of a human. This paper investigates the cellular states of university students by analyzing body composition features such as body cell mass (BCM), total body water (TBW), skeletal muscle mass (SMM), basal metabolic rate (BMR) and bio-impedance measurements including bioelectrical impedance (BI), phase angle (PA) of human body. Utilizing bioelectrical impedance spectroscopy (BIS), data were collected and categorized into healthy cellular states (HCS) and stiffened cellular states (SCS) based on body cell mass (BCM) and total body water (TBW). Bioelectrical impedance vector analysis (BIVA) and Nyquist plot were employed for further differentiation. Support-vector machines (SVM), decision trees (DT), and random forest (RF) machine-learning models were applied for classification, with RF exhibiting the highest accuracy. Results reveal that students in HCS exhibit elevated BCM (35.22–55.68 kg), TBW (39.25–56.61 l), SMM (26.83–34.93 kg), BMR (25.67—28.67 kg), and PA (7.21–9.29 degree), but lower BI (443.39–569.45 Ω) compared to SCS students. Therefore, RF classifier was selected for its superior performance in detecting cellular states.
更多查看译文
关键词
Cellular states,Machine-learning models,Bioelectrical impedance spectroscopy,BIVA,Nyquist plot
AI 理解论文
溯源树
样例
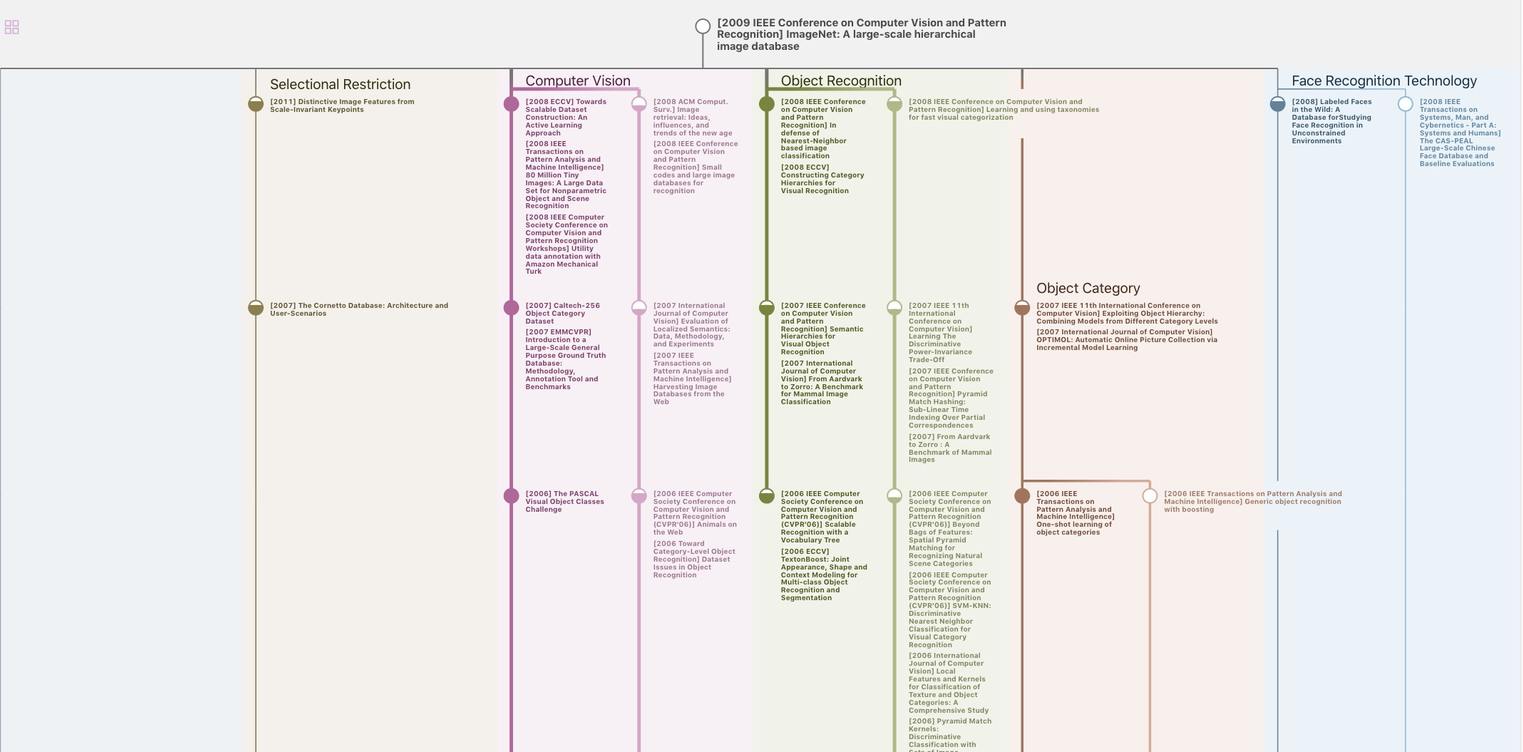
生成溯源树,研究论文发展脉络
Chat Paper
正在生成论文摘要