Knowledge Transfer for On-Device Deep Reinforcement Learning in Resource Constrained Edge Computing Systems
IEEE ACCESS(2020)
摘要
Deep reinforcement learning (DRL) is a promising approach for developing control policies by learning how to perform tasks. Edge devices are required to control their actions by exploiting DRL to solve tasks autonomously in various applications such as smart manufacturing and autonomous driving. However, the resource limitations of edge devices make it unfeasible for them to train their policies from scratch. It is also impractical for such an edge device to use the policy with a large number of layers and parameters, which is pre-trained by a centralized cloud infrastructure with high computational power. In this paper, we propose a method, on-device DRL with distillation (OD3), to efficiently transfer distilled knowledge of how to behave for on-device DRL in resource-constrained edge computing systems. Our proposed method makes it possible to simultaneously perform knowledge transfer and policy model compression in a single training process on edge devices with considering their limited resource budgets. The novelty of our method is to apply a knowledge distillation approach to DRL based edge device control in integrated edge cloud environments. We analyze the performance of the proposed method by implementing it on a commercial embedded system-on-module equipped with limited hardware resources. The experimental results show that 1) edge policy training with the proposed method achieves near-cloud-performance in terms of average rewards, although the size of the edge policy network is significantly smaller compared to that of the cloud policy network and 2) the training time elapsed for edge policy training with our method is reduced significantly compared to edge policy training from scratch.
更多查看译文
关键词
Training,Cloud computing,Task analysis,Computational modeling,Hardware,Edge computing,Artificial intelligence,Deep reinforcement learning,edge computing,edge AI,knowledge transfer,policy model compression,on-device training
AI 理解论文
溯源树
样例
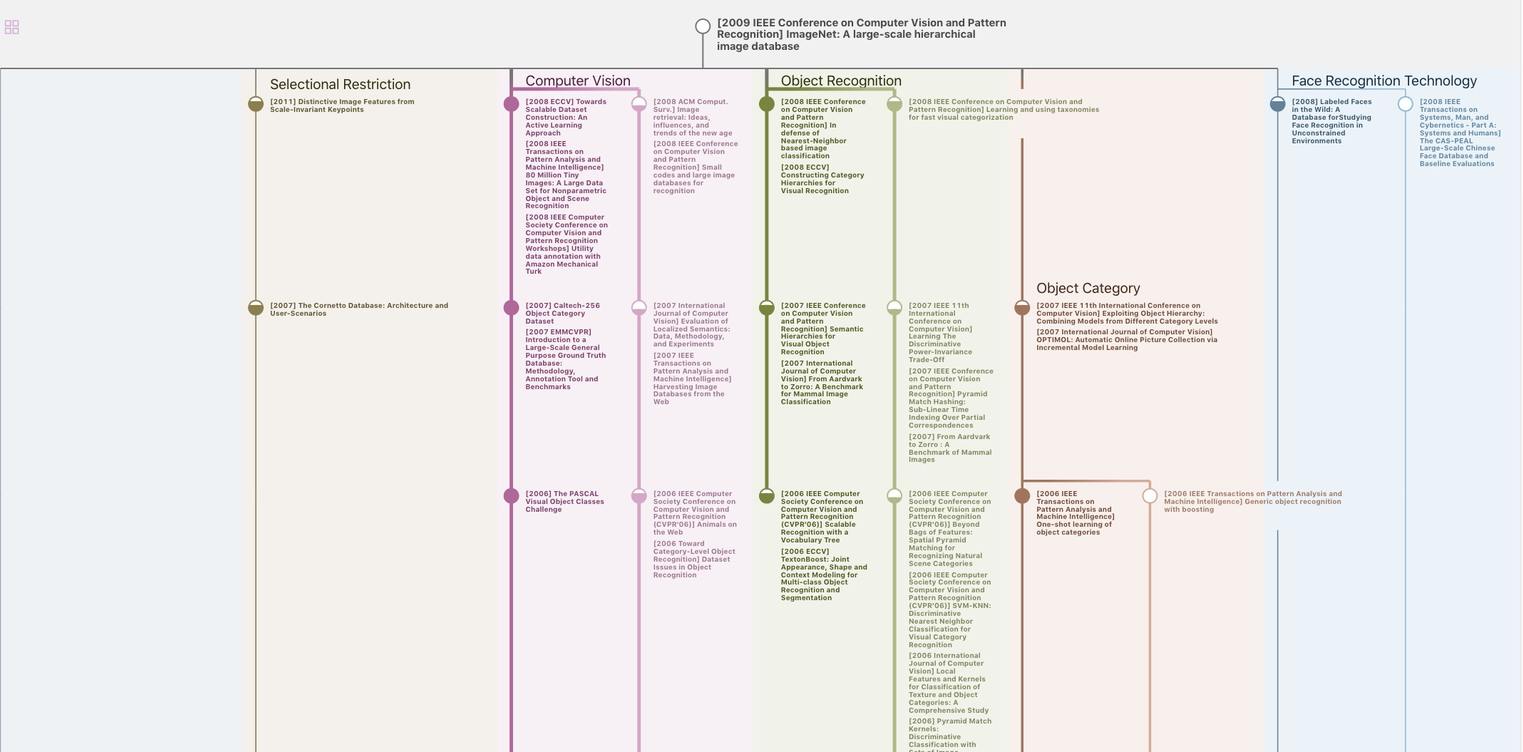
生成溯源树,研究论文发展脉络
Chat Paper
正在生成论文摘要