A Coarse-to-Fine Approach for Intelligent Logging Lithology Identification with Extremely Randomized Trees
MATHEMATICAL GEOSCIENCES(2020)
摘要
Lithology identification is vital for reservoir exploration and petroleum engineering. Recently, there has been growing interest in using an intelligent logging approach for lithology classification. Machine learning has emerged as a powerful tool in inferring lithology types with the logging curves. However, well logs are susceptible to logging parameter manual entry, borehole conditions and tool calibrations. Most studies in the field of lithology classification with machine learning approaches have focused only on improving the prediction accuracy of classifiers. Also, a model trained in one location is not reusable in a new location due to different data distributions. In this paper, a unified framework is provided for training a multi-class lithology classification model for a data set with outlier data. In this paper, a coarse-to-fine framework that combines outlier detection, multi-class classification with an extremely randomized tree-based classifier is proposed to solve these issues. An unsupervised learning approach is used to detect the outliers in the data set. Then a coarse-to-fine inference procedure is used to infer the lithology class with an extremely randomized tree classifier. Two real-world data sets of well-logging are used to demonstrate the effectiveness of the proposed framework. Comparisons are conducted with some baseline machine learning classifiers, namely random forest, gradient tree boosting, and xgboosting. Results show that the proposed framework has higher prediction accuracy in sandstones compared with other approaches.
更多查看译文
关键词
Lithology classification, Ensemble methods, Outlier detection
AI 理解论文
溯源树
样例
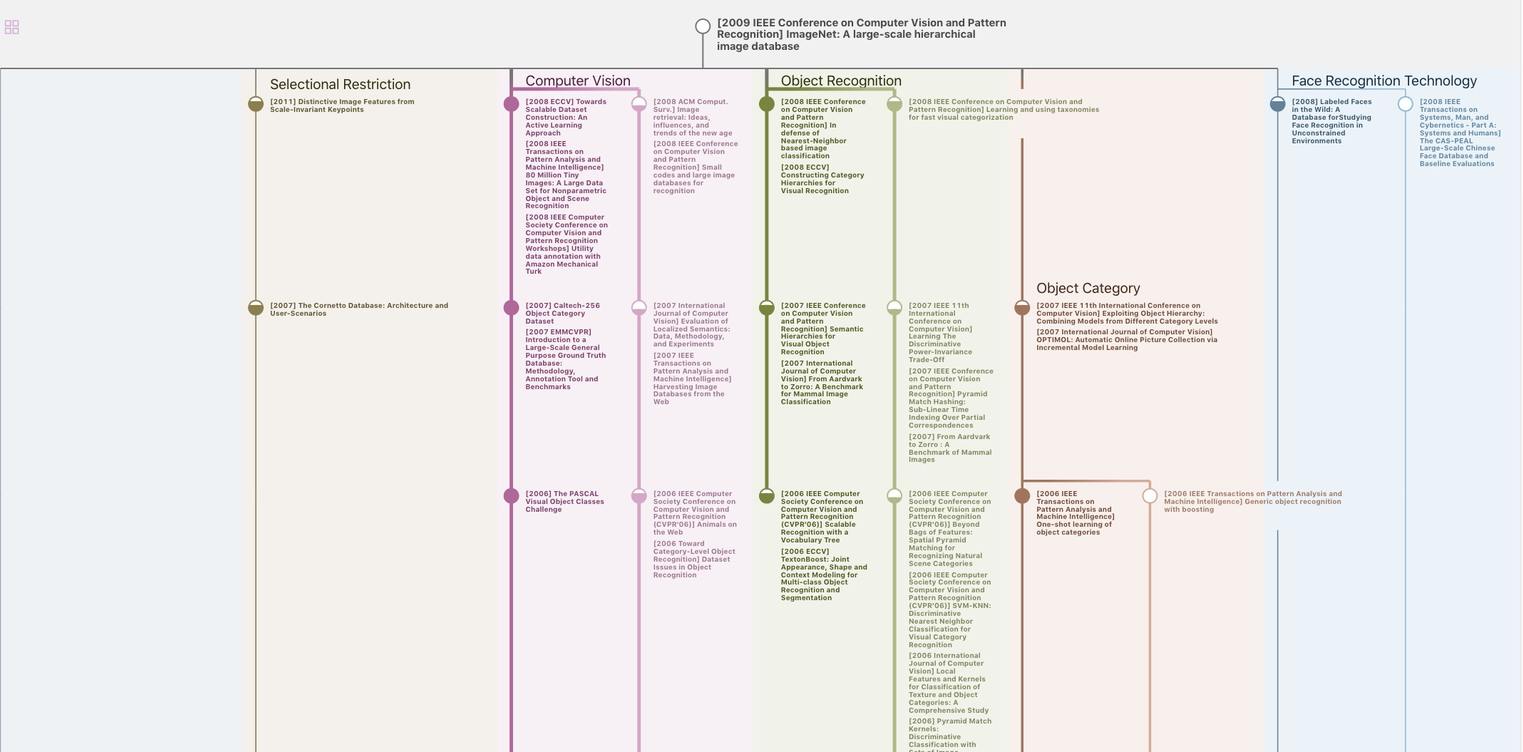
生成溯源树,研究论文发展脉络
Chat Paper
正在生成论文摘要