Interval Feature Transformation for Time Series Classification Using Perceptually Important Points
APPLIED SCIENCES-BASEL(2020)
摘要
A novel feature reconstruction method, referred to as interval feature transformation (IFT), is proposed for time series classification. The IFT uses perceptually important points to segment the series dynamically into subsequences of unequal length, and then extract interval features from each time series subsequence as a feature vector. The IFT distinguishes the best top-k discriminative feature vectors from a data set by information gain. Utilizing these discriminative feature vectors, transformation is applied to generate new k-dimensional data which are lower-dimensional representations of the original data. In order to verify the effectiveness of this method, we use the transformed data in conjunction with some traditional classifiers to solve time series classification problems and make comparative experiments to several state-of-the-art algorithms. Experiment results verify the effectiveness, noise robustness and interpretability of the IFT.
更多查看译文
关键词
interval feature,transformation,time series classification,perceptually important points
AI 理解论文
溯源树
样例
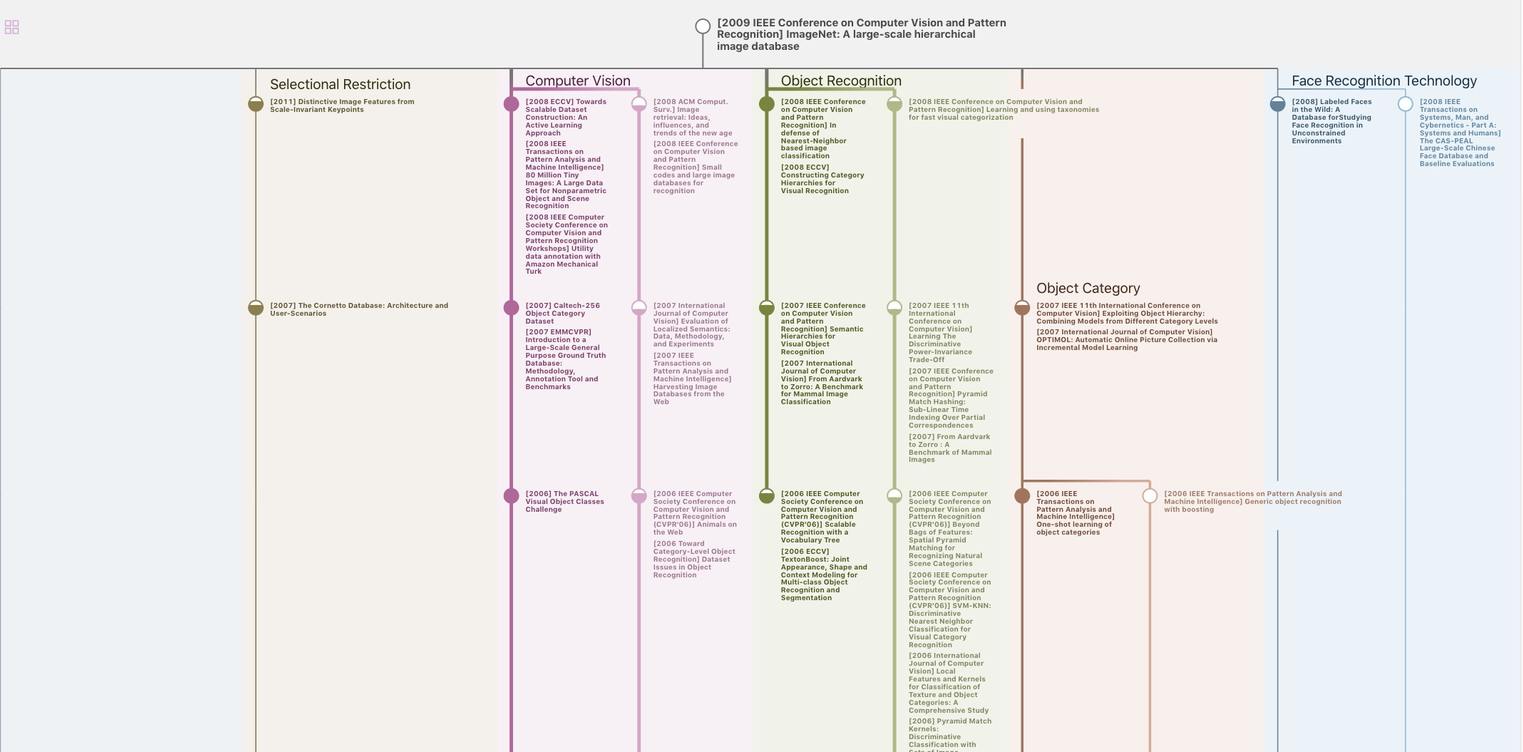
生成溯源树,研究论文发展脉络
Chat Paper
正在生成论文摘要