A Dynamic Two-Layers Mi And Clustering-Based Ensemble Feature Selection For Multi-Labels Text Classification
INTERNATIONAL JOURNAL OF ADVANCED COMPUTER SCIENCE AND APPLICATIONS(2020)
摘要
Multi-label text classification deals with the issue that arises from each sample being related to multiple labels. The text data suffers from high dimensionality. In order to resolve this issue, a feature selection (FS) method can be implemented for efficiently removing the noisy, irrelevant, and redundant features. Multi-label FS is a powerful tool for solving the high-dimension problem. With regards to handling correlation and high dimensionality problems in multi-label text classification, this paper investigates the various heterogeneous FS ensemble schemes. In addition, this paper proposes an enhanced FS method called dynamic multi-label two-layers MI and clustering-based ensemble feature selection algorithm (DMMC-EFS). The proposed method considers the: 1) dynamic global weight of feature, 2) heterogeneous ensemble, and 3) maximum dependency and relevancy and minimum redundancy of features. This method aims to overcome the high dimensionality of multi-label datasets and acquire improved multi-label text classification. We have conducted experiments based on three benchmark datasets: Reuters-21578, Bibtex, and Enron. The experimental results show that DMMC-EFS has significantly outperformed other state-of-the-art conventional and ensemble multi-label FS methods.
更多查看译文
关键词
Multi-label text classification, high dimensionality, filtering method, ensemble clustering, ensemble MI feature selection
AI 理解论文
溯源树
样例
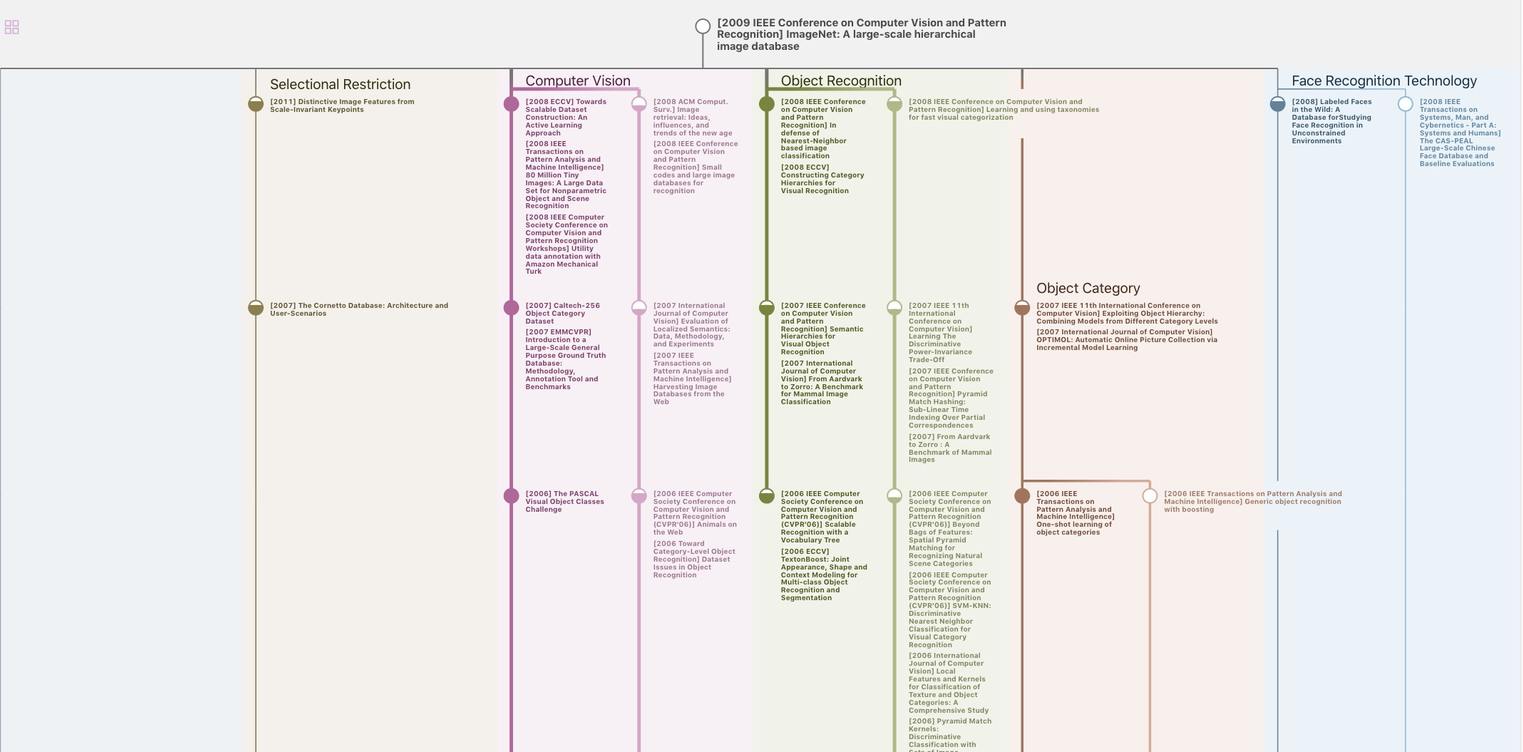
生成溯源树,研究论文发展脉络
Chat Paper
正在生成论文摘要