Potential of Ensemble Learning to Improve Tree-Based Classifiers for Landslide Susceptibility Mapping
IEEE JOURNAL OF SELECTED TOPICS IN APPLIED EARTH OBSERVATIONS AND REMOTE SENSING(2020)
摘要
Ensemble learning methods have been widely used due to their remarkable generalized performance, but their potential in landslide spatial prediction application is not fully studied. To take full advantage of ensemble learning techniques, the classification and regression tree classifier and four tree-based ensemble classifiers of random forest, extremely randomized tree, gradient boosting decision trees, and extreme gradient boosting decision trees are used in this study for landslide susceptibility assessment. Specifically, a stacking ensemble learning framework coupled with embedded feature selection is presented, consisting of multiple tree-based classifiers mentioned previously as base learners and logistic regression as a metalearner in a two-layer structure. In the study area of Yongxin, China, 364 historical landslide locations were first randomly partitioned into a ratio of 7/3 for training and testing the model. Then, a spatial database of 16 landslide causative factors was constructed for landslide prediction. Meanwhile, the relative importance of these factors were quantified by using the total number of feature splits and the average Gini index during the training process, and a novel embedded feature selection method was used in the base learner of the proposed framework to further improve the computational efficiency and predictive performance by allowing each base learner to obtain its own optimal subfeature space. Finally, different methods were assessed by using several evaluation criteria. Experimental results demonstrated that the proposed ensemble learning framework had the highest area under the curve value of 0.864, and it is more effective than the conventional tree-based classifiers and other ensemble learning methods.
更多查看译文
关键词
Terrain factors,Stacking,Geology,Indexes,Boosting,Feature extraction,Embedded feature selection,ensemble learning,landslides susceptibility mapping,tree-based classifiers
AI 理解论文
溯源树
样例
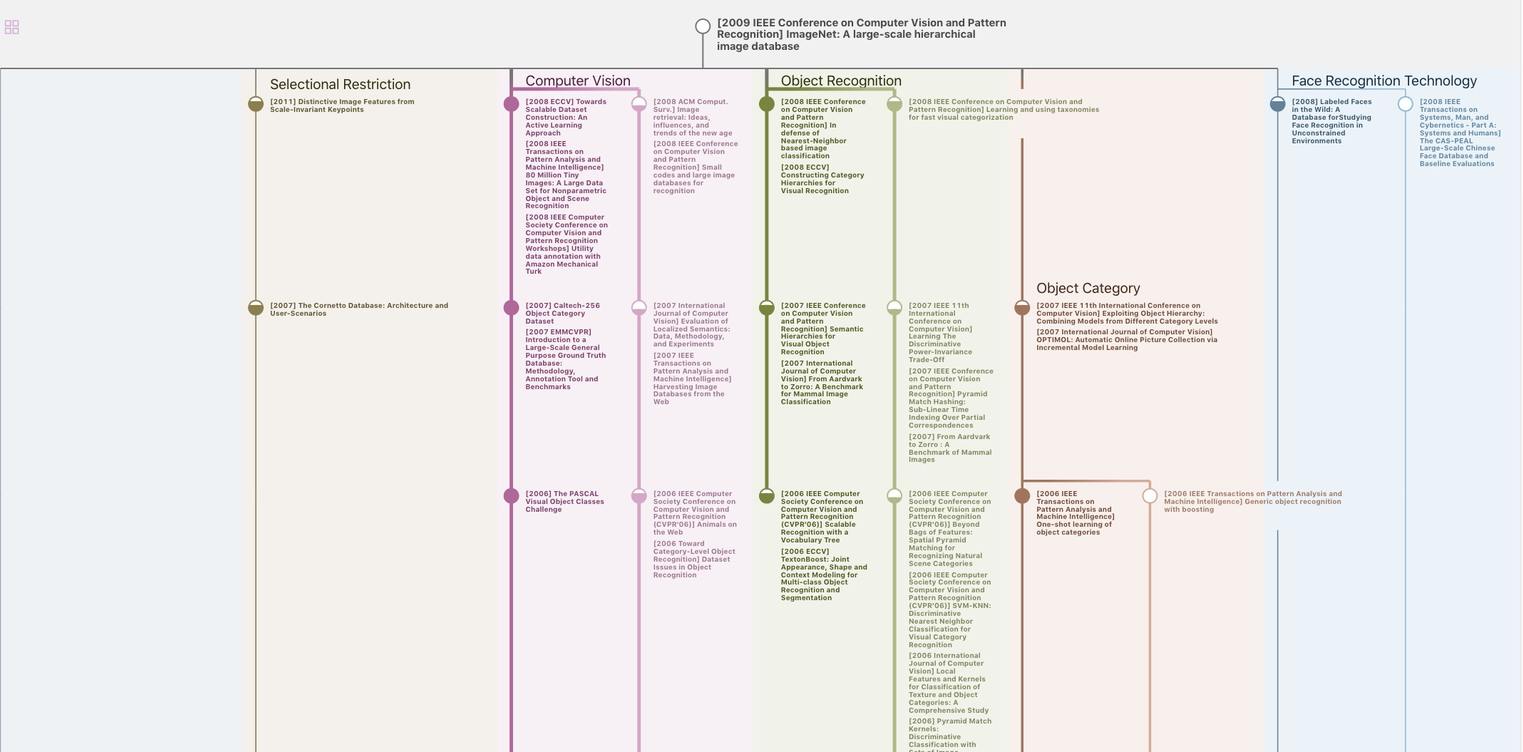
生成溯源树,研究论文发展脉络
Chat Paper
正在生成论文摘要