Bayesian Optimization of Expected Quadratic Loss for Multiresponse Computer Experiments with Internal Noise
SIAM-ASA JOURNAL ON UNCERTAINTY QUANTIFICATION(2020)
摘要
Design of systems based on computer simulations is prevalent. An important idea to improve design quality, called robust parameter design (RPD), is to optimize control factors based on the expectation of a loss function so that the design is robust to noise factor variations. When computer simulations are time consuming, optimizing the simulator based on a Gaussian process (GP) emulator for the response is a computationally efficient approach. For this purpose, acquisition functions (AFs) are used to sequentially determine the next design point so that the GP emulator can more accurately locate the optimal setting. Despite this, few articles consider AFs for positive definite quadratic forms such as the expected quadratic loss (EQL) function, which is the standard expected loss function for RPD with nominally-the-best responses. This paper proposes new AFs for optimizing the EQL, analyzes their convergence, and develops quick and accurate methods based on the characteristic function of the EQL to compute them. We apply the AFs to RPD problems with internal noise factors based on a GP model and an initial design tailored for such problems. Numerical results indicate that all four AFs considered have similar performance, and they outperform an optimization approach based on modeling the quadratic loss as a GP and maximin Latin hypercube designs. MATLAB codes for reproducing reported results are given in the online supplement.
更多查看译文
关键词
Bayesian optimization,expected quadratic loss,expected improvement,characteristic function
AI 理解论文
溯源树
样例
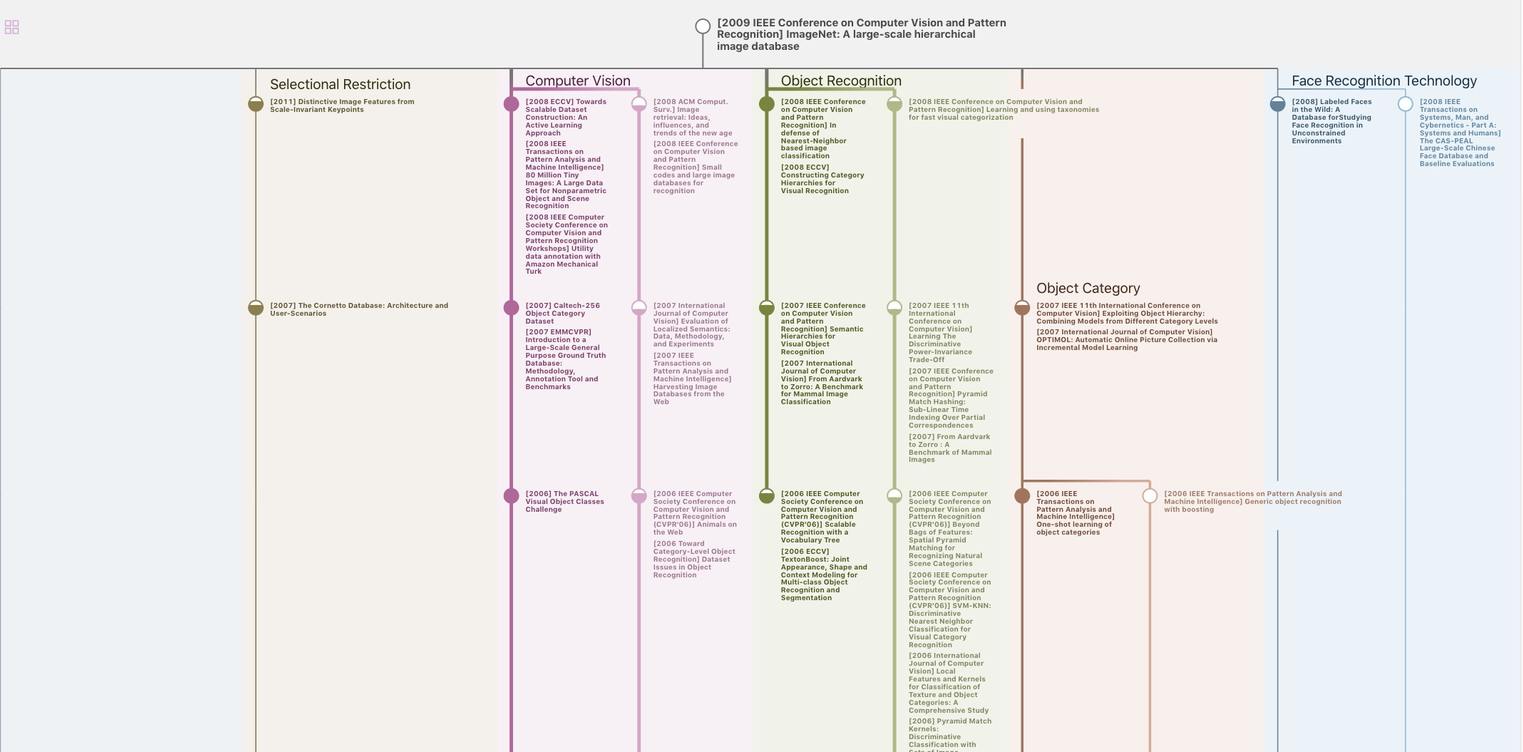
生成溯源树,研究论文发展脉络
Chat Paper
正在生成论文摘要