Indoor/Outdoor Switching Detection Using Multisensor DenseNet and LSTM
IEEE Internet of Things Journal(2021)
摘要
In recent years, with the widespread popularity of smart mobile devices, seamless location-based service (LBS) between indoor and outdoor (IO) space has become an emerging requirement. As the direct indicator of location and contextual status, accurate indoor/outdoor status guarantees stability and continuity for human localization and activity recognition. Rich-sensor smartphones have made possible various context sensing. However, it is challenging to realize fast and accurate IO switching detection in dynamic and complex environments with variable sensory signals. Toward this end, we propose a multisensor deep learning model to predict the IO state, which consists of four parts: 1) sensor vectors; 2) 1-D sensor DenseNet; 3) LSTM; and 4) MLP. Unlike the traditional feature engineering and fixed threshold strategy, we utilize 1-D sensor DenseNet to extract higher level features for different sensors, and then utilize LSTM to capture signal change pattern in time series. Finally, we leverage the MLP to classify indoor/outdoor scenes. We evaluated our proposed architecture on two data sets, one for only differentiating IO scenes and one for detecting the indoor/outdoor switching scenes. Extensive experimental results show that our proposed method outperforms the fixed threshold method, traditional machine learning-based methods, and other existing neural network-based methods in terms of switching delay and recognition accuracy.
更多查看译文
关键词
Deep learning,DenseNet,indoor/outdoor detection,LSTM,seamless indoor/outdoor positioning
AI 理解论文
溯源树
样例
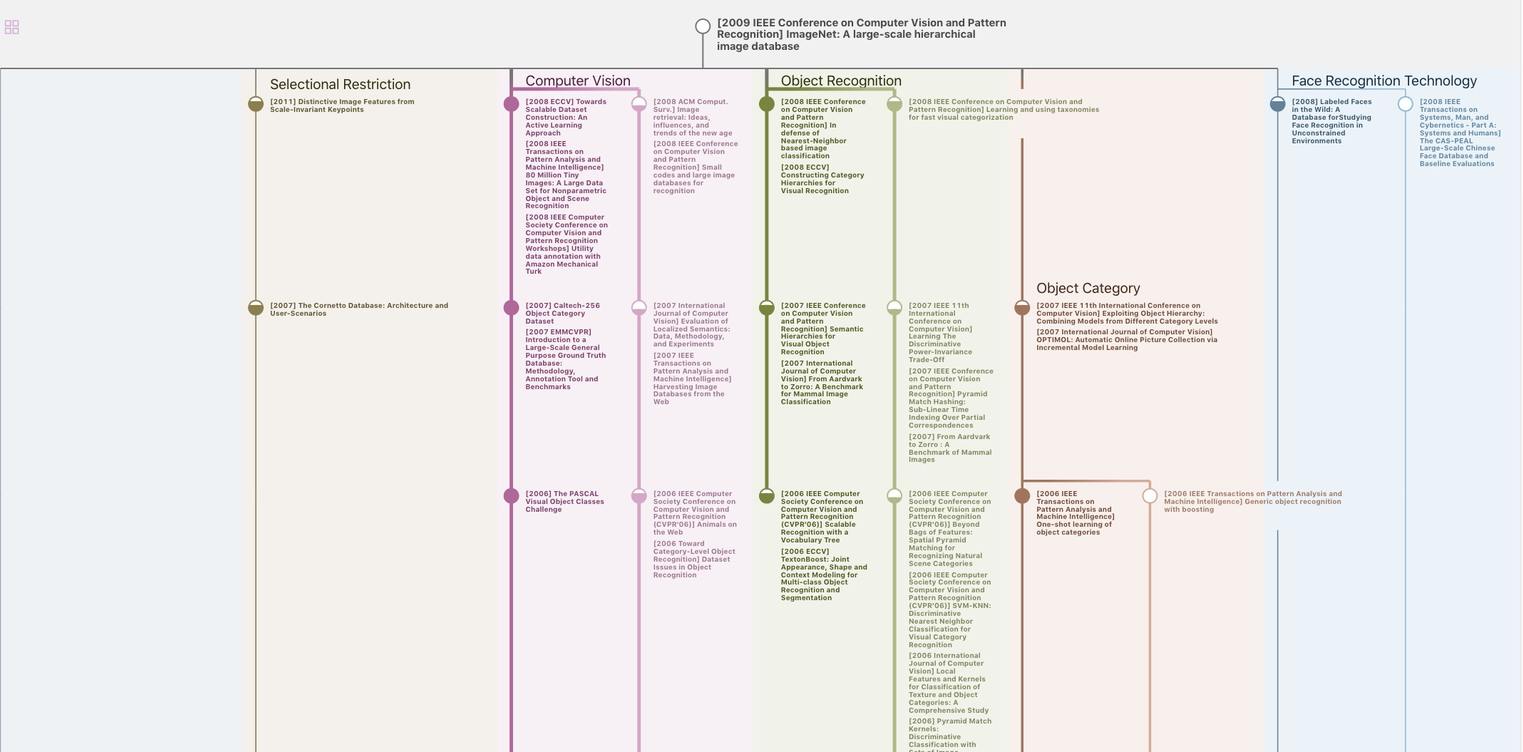
生成溯源树,研究论文发展脉络
Chat Paper
正在生成论文摘要