Unsupervised Optical Flow Estimation Based on Improved Feature Pyramid
NEURAL PROCESSING LETTERS(2020)
摘要
Deep learning methods for optical flow estimation usually increase the receptive field of convolution through reducing image resolution, which results in loss of spatial detail information during feature extraction. In this paper, we introduce dilated convolution into feature pyramid network, which can extract multi-scale features containing more motion details and can further improve the accuracy of optical flow estimation. The unsupervised loss function is based on forward–backward consistency check and robust census transform that has a good constraint performance in the case of illumination changes, which can train an unsupervised learning optical flow model with higher accuracy. Our network is trained on FlyingChairs and KITTI raw datasets with an unsupervised manner and tested on MPI-Sintel, KITTI 2012 and KITTI 2015 benchmarks. The experimental results show the advantages of our method in unsupervised learning approaches.
更多查看译文
关键词
Optical flow estimation,Deep learning,Feature pyramid,Dilated convolution
AI 理解论文
溯源树
样例
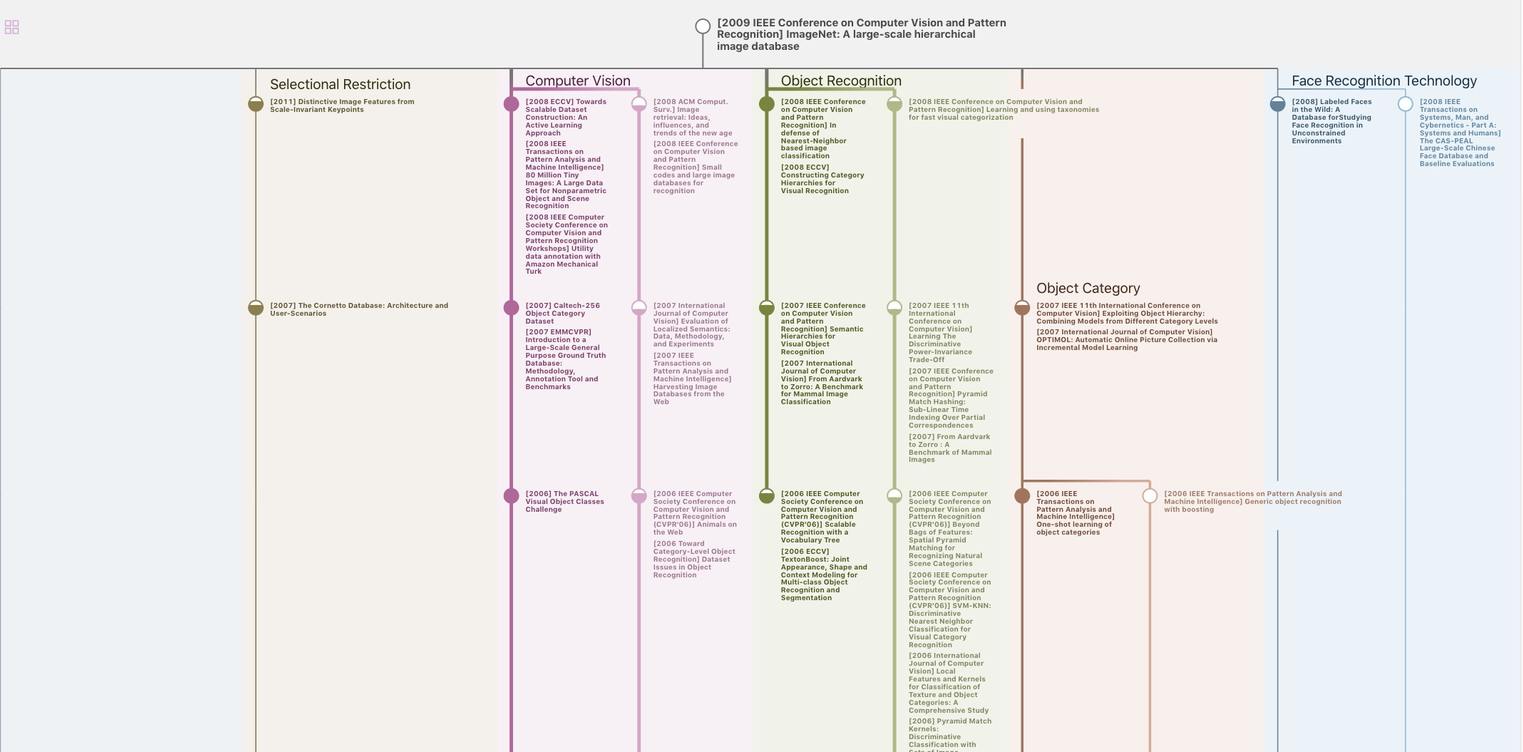
生成溯源树,研究论文发展脉络
Chat Paper
正在生成论文摘要