A Machine Learning Approach to Dataset Imputation for Software Vulnerabilities.
MCSS(2020)
摘要
This paper proposes a supervised machine learning approach for the imputation of missing categorical values in a dataset where the majority of samples are incomplete. Twelve models have been designed that can predict nine of the twelve Adversarial Tactics, Techniques, and Common Knowledge (ATT\u0026CK) tactic categories using only the Common Attack Pattern Enumeration and Classification (CAPEC). The proposed method has been evaluated on a test dataset consisting of 867 unseen samples, with the classification accuracy ranging from 99.88% to 100%. These models were employed to generate a more complete dataset with no missing ATT\u0026CK tactic features.
更多查看译文
关键词
Cyber security, Vulnerability, Mitre ATT&CK, Machine learning, Dataset, Imputation
AI 理解论文
溯源树
样例
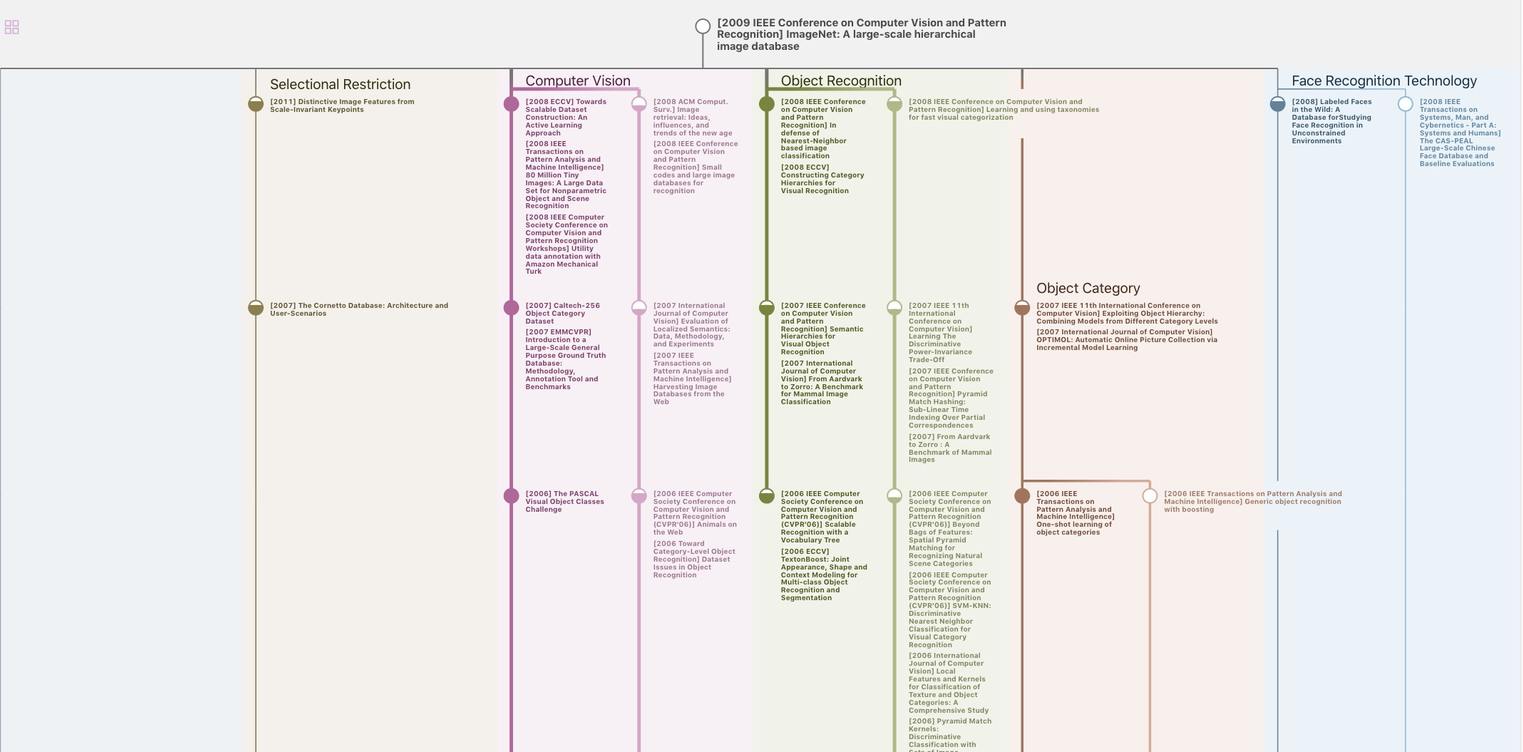
生成溯源树,研究论文发展脉络
Chat Paper
正在生成论文摘要