Updating Knowledge In Multigranulation Decision-Theoretic Rough Set Model Based On Decision Support Degree
JOURNAL OF ENGINEERING-JOE(2020)
摘要
Based on the majority rules, a multigranulation decision-theoretic rough set model based on the decision support degree is proposed, in which the thresholds can be computed by the decision risk minimisation based on the Bayesian decision-theoretic. In various practical situations, information systems may alter dynamically with time. Incremental learning is an alternative manner for maintaining knowledge by utilising previous computational results under dynamic data. Therefore, the authors investigate dynamic approaches to update the knowledge in the new model when adding or deleting granular structures. Besides, the corresponding dynamic and static algorithms are designed and their time complexities are analysed. Finally, comparative experiments by using six data sets from UCI are carried out; the results illustrate that the proposed dynamic algorithm is effective and is more efficient than the static algorithm.
更多查看译文
关键词
Bayes methods, granular computing, decision theory, rough set theory, learning (artificial intelligence), multigranulation decision-theoretic rough set model, decision support degree, decision risk minimisation, Bayesian decision-theoretic, incremental learning, static algorithm
AI 理解论文
溯源树
样例
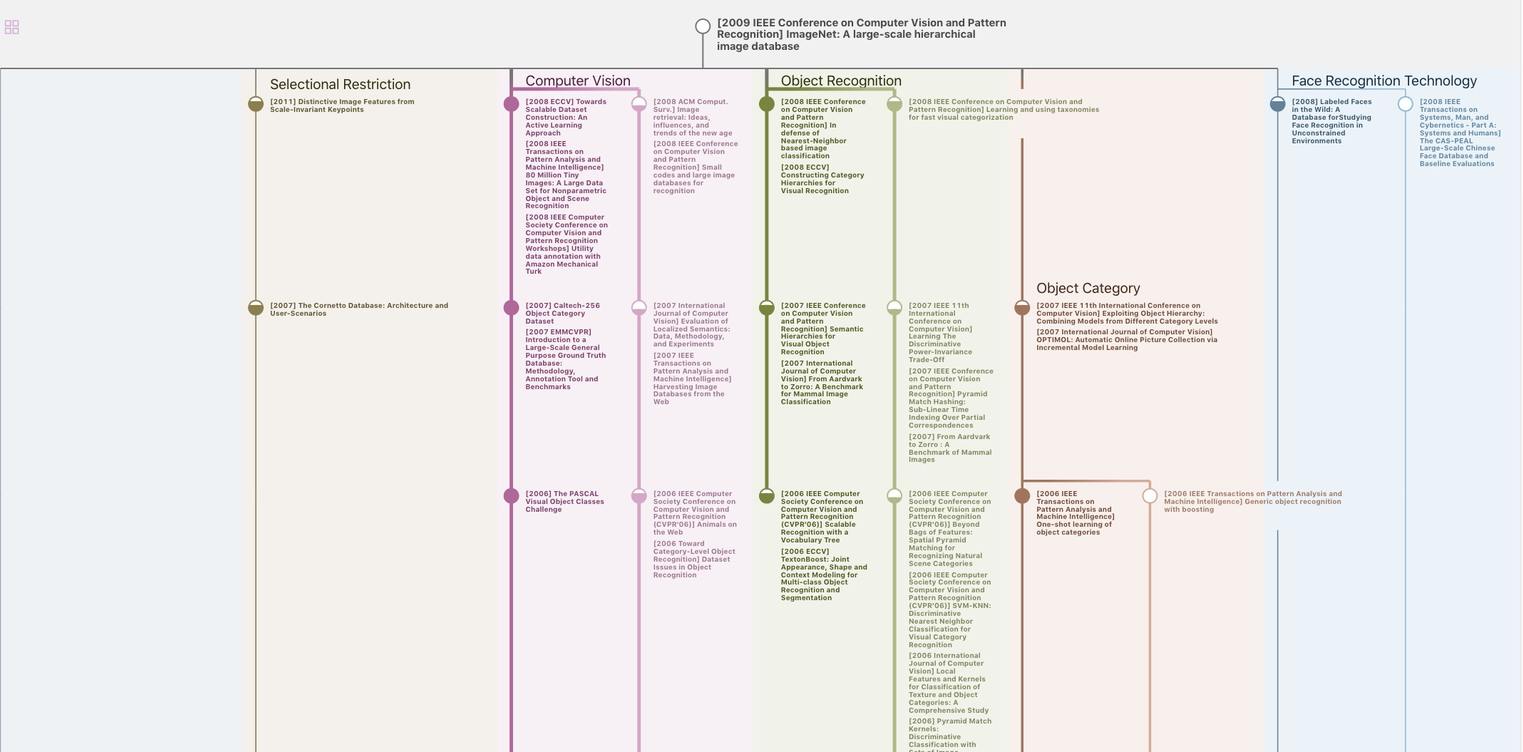
生成溯源树,研究论文发展脉络
Chat Paper
正在生成论文摘要