Mining arguments in scientific abstracts with discourse-level embeddings
data and knowledge engineering(2020)
摘要
Abstract Argument mining consists in the automatic identification of argumentative structures in texts. In this work we leverage existing discourse-level annotations to facilitate the identification of argumentative components and relations in scientific texts, which has been recognized as a particularly challenging task. We propose a new annotation schema and use it to augment a corpus of computational linguistics abstracts that had previously been annotated with discourse units and relations. Our initial experiments with the enriched corpus confirm the potential value of incorporating discourse information in argument mining tasks. In order to tackle the limitations posed by the lack of corpora containing both discourse and argumentative annotations we explore two transfer learning approaches in which discourse parsing is used as an auxiliary task when training argument mining models. In this case, as no discourse information is used as input, the resulting models could be used to predict the argumentative structure of unannotated texts.
更多查看译文
AI 理解论文
溯源树
样例
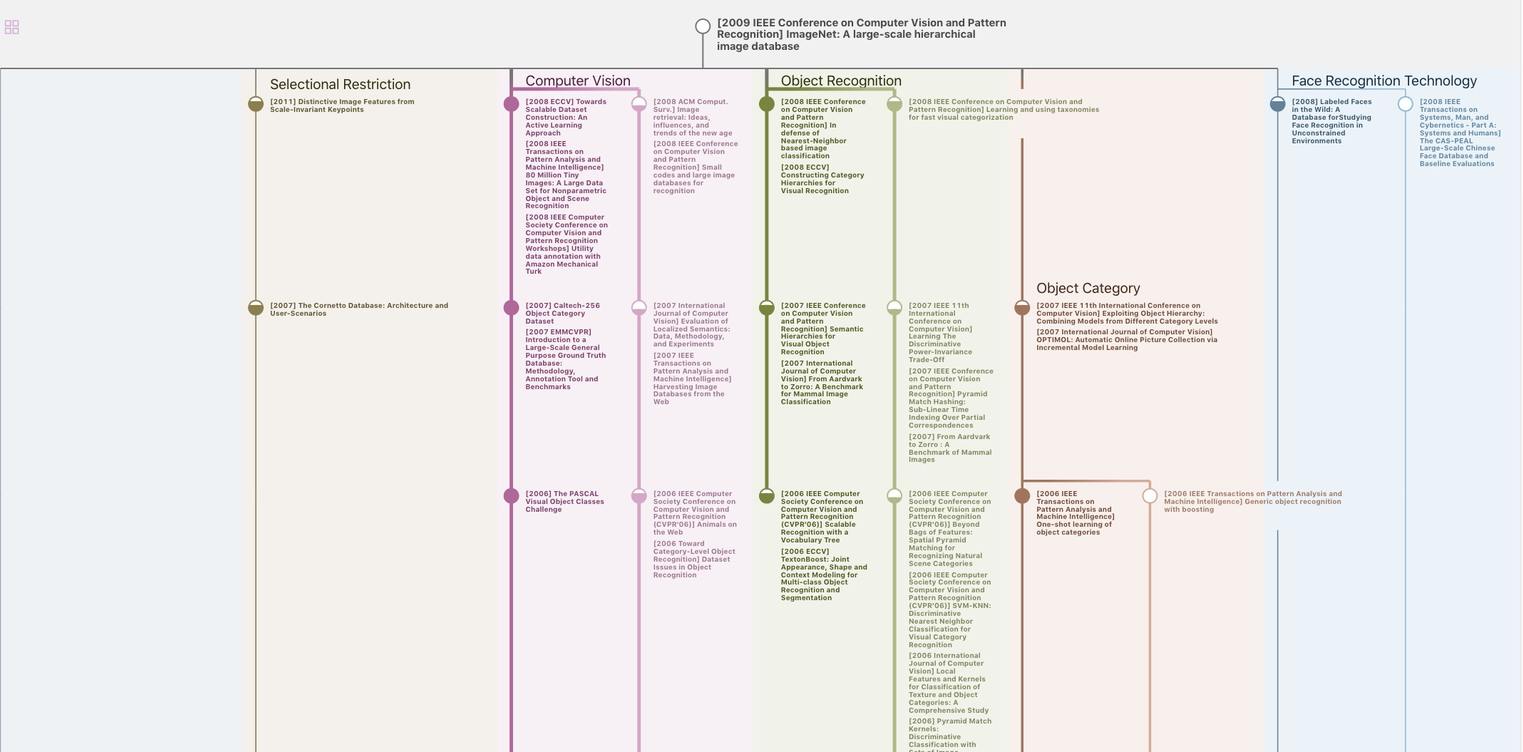
生成溯源树,研究论文发展脉络
Chat Paper
正在生成论文摘要