Probabilistic vs deep learning based approaches for narrow domain NER in Spanish.
JOURNAL OF INTELLIGENT & FUZZY SYSTEMS(2020)
摘要
This work presents an experimental study on the task of Named Entity Recognition (NER) for a narrow domain in Spanish language. This study considers two approaches commonly used in this kind of problem, namely, a Conditional Random Fields (CRF) model and Recurrent Neural Network (RNN). For the latter, we employed a bidirectional Long Short-Term Memory with ELMO's pre-trained word embeddings for Spanish. The comparison between the probabilistic model and the deep learning model was carried out in two collections, the Spanish dataset from CoNLL-2002 considering four classes under the IOB tagging schema, and aMexican Spanish news dataset with seventeen classes under IOBES schema. The paper presents an analysis about the scalability, robustness, and common errors of both models. This analysis indicates in general that the BiLSTM-ELMo model is more suitable than the CRF model when there is "enough" training data, and also that it is more scalable, as its performance was not significantly affected in the incremental experiments (by adding one class at a time). On the other hand, results indicate that the CRF model is more adequate for scenarios having small training datasets and many classes.
更多查看译文
关键词
Named entity recognition,CRF,Bi-LSTM,Spanish,news reports
AI 理解论文
溯源树
样例
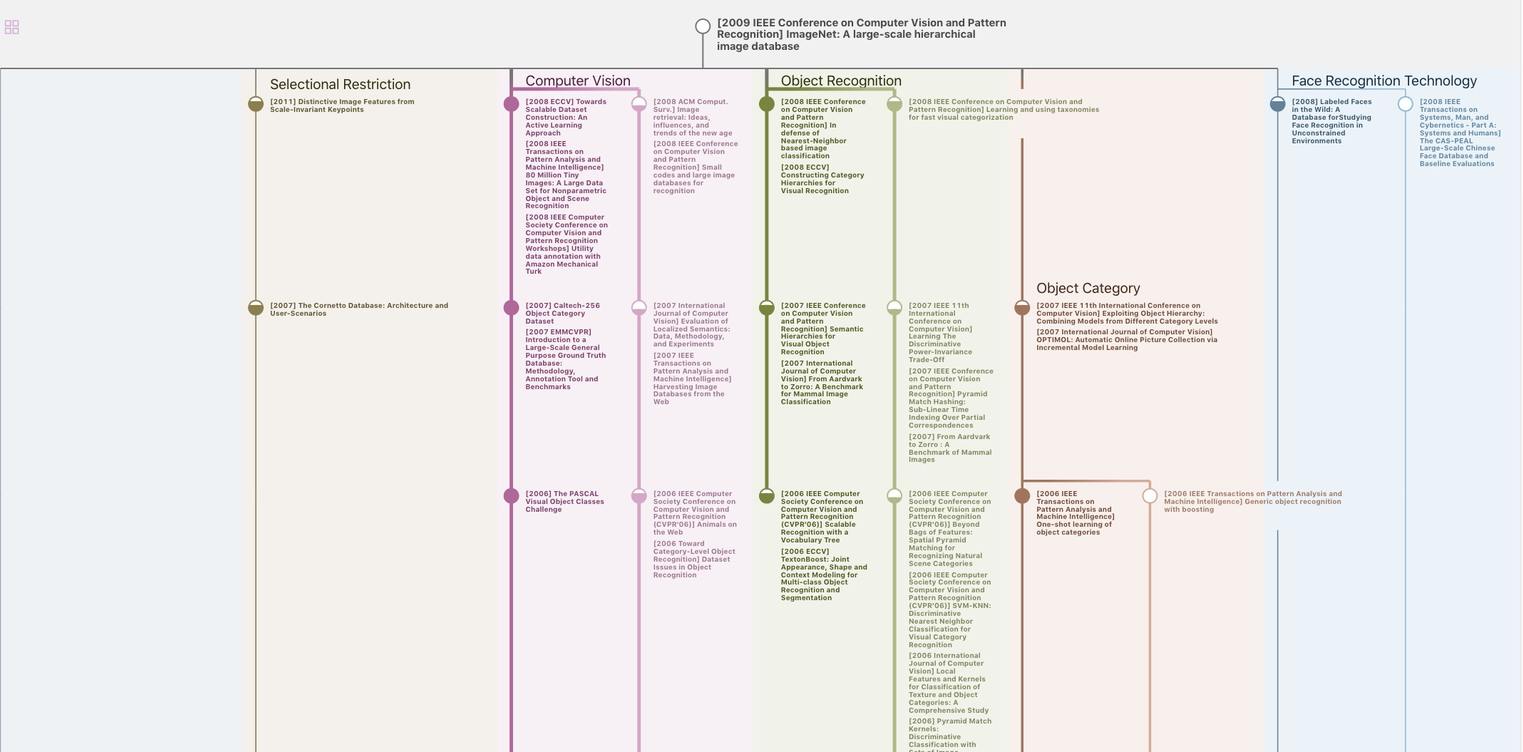
生成溯源树,研究论文发展脉络
Chat Paper
正在生成论文摘要