Machine Learning Application in Reservoir Water Level Forecasting for Sustainable Hydropower Generation Strategy
SUSTAINABILITY(2020)
摘要
The aim of this study is to accurately forecast the changes in water level of a reservoir located in Malaysia with two different scenarios; Scenario 1 (SC1) includes rainfall and water level as input and Scenario 2 (SC2) includes rainfall, water level, and sent out. Different time horizons (one day ahead to seven days) will be investigated to check the accuracy of the proposed models. In this study, four supervised machine learning algorithms for both scenarios were proposed such as Boosted Decision Tree Regression (BDTR), Decision Forest Regression (DFR), Bayesian Linear Regression (BLR) and Neural Network Regression (NNR). Eighty percent of the total data were used for training the datasets while 20% for the dataset used for testing. The models' performance is evaluated using five statistical indexes; the Correlation Coefficient (R-2), Mean Absolute Error (MAE), Root Mean Square Error (RMSE), Relative Absolute Error (RAE), and Relative Squared Error (RSE). The findings showed that among the four proposed models, the BLR model outperformed other models with R(2)0.998952 (1-day ahead) for SC1 and BDTR for SC2 with R(2)0.99992 (1-day ahead). With regards to the uncertainty analysis, 95PPU and d-factors were adopted to measure the uncertainties of the best models (BLR and BDTR). The results showed the value of 95PPU for both models in both scenarios (SC1 and SC2) fall into the range between 80% to 100%. As for the d-factor, all values in SC1 and SC2 fall below one.
更多查看译文
关键词
forecasting water level,machine learning algorithms,boosted decision tree regression,decision forest regression,neural network regression,Bayesian linear regression
AI 理解论文
溯源树
样例
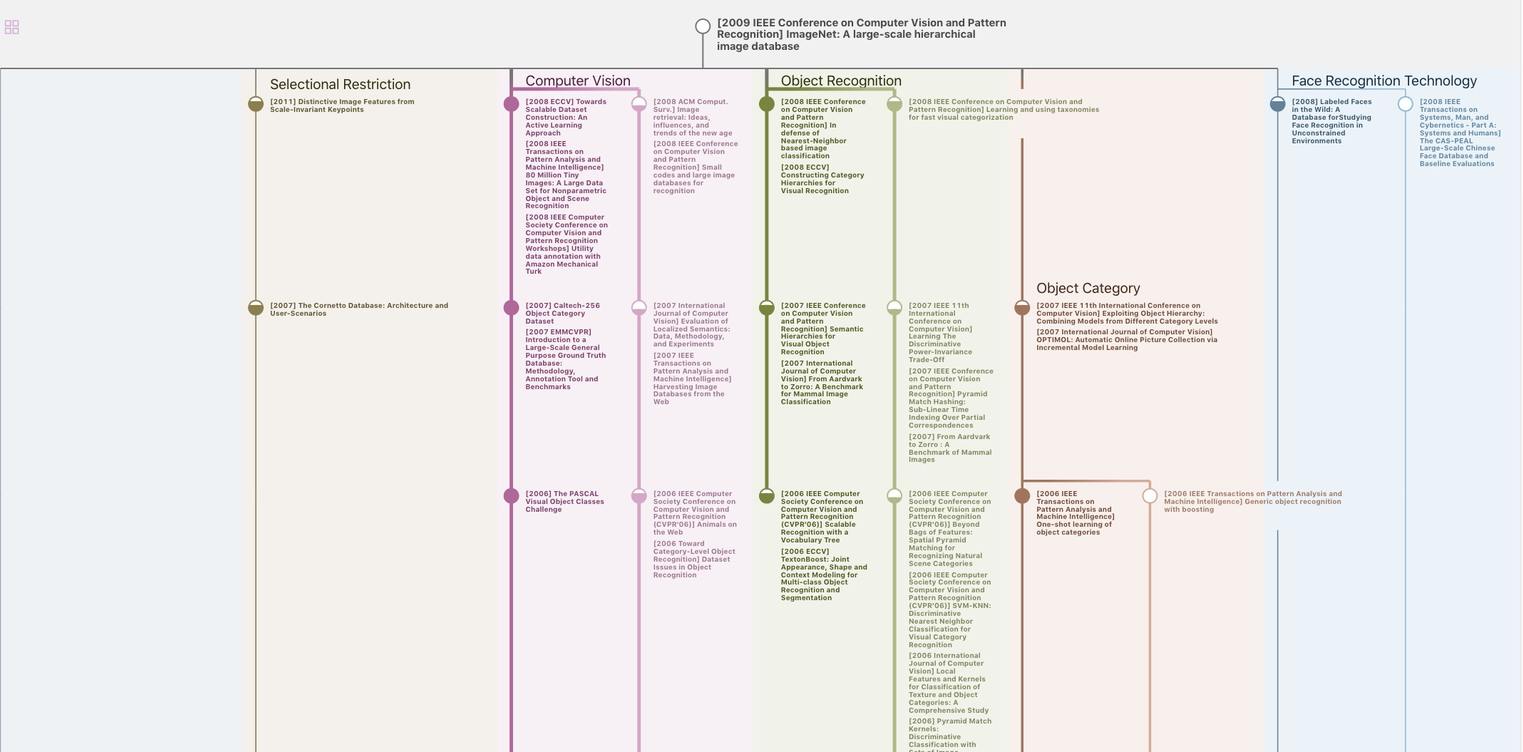
生成溯源树,研究论文发展脉络
Chat Paper
正在生成论文摘要