VSA-CGAN: An Intelligent Generation Model for Deep Learning Sample Database Construction
IEEE ACCESS(2020)
摘要
In order to solve the problem of model accuracy reduction caused by the difficulty of obtaining specific training samples or the insufficient number of samples in the application of existing object detection and recognition model based on deep learning, this article proposes a conditional generative adversarial network model (VSA-CGAN), which integrates the self-attention mechanism of visual perception to optimize the inference of object attention feature maps, so as to learn the global information of the image and the detailed features of the object. It is designed to add conditional features in the generator and the discriminator, associate the specific dimensions of the data with the semantic features, and explicitly indicate the model to generate the corresponding object signature category information, so as to generate the feature representation of the image which is more suitable for the distribution of the original data. The model in this article has completed numerical experiments on several general standard data sets, and compared with several mainstream generative adversarial network models in image data augmentation performance. The experimental results show that the generation model in this article has excellent object simulation ability and strong application prospects.
更多查看译文
关键词
Generative adversarial networks,Data models,Generators,Gallium nitride,Feature extraction,Numerical models,Machine learning,Generative adversarial network,attention mechanism,visual salience,object simulation,deep learning,data augmentation
AI 理解论文
溯源树
样例
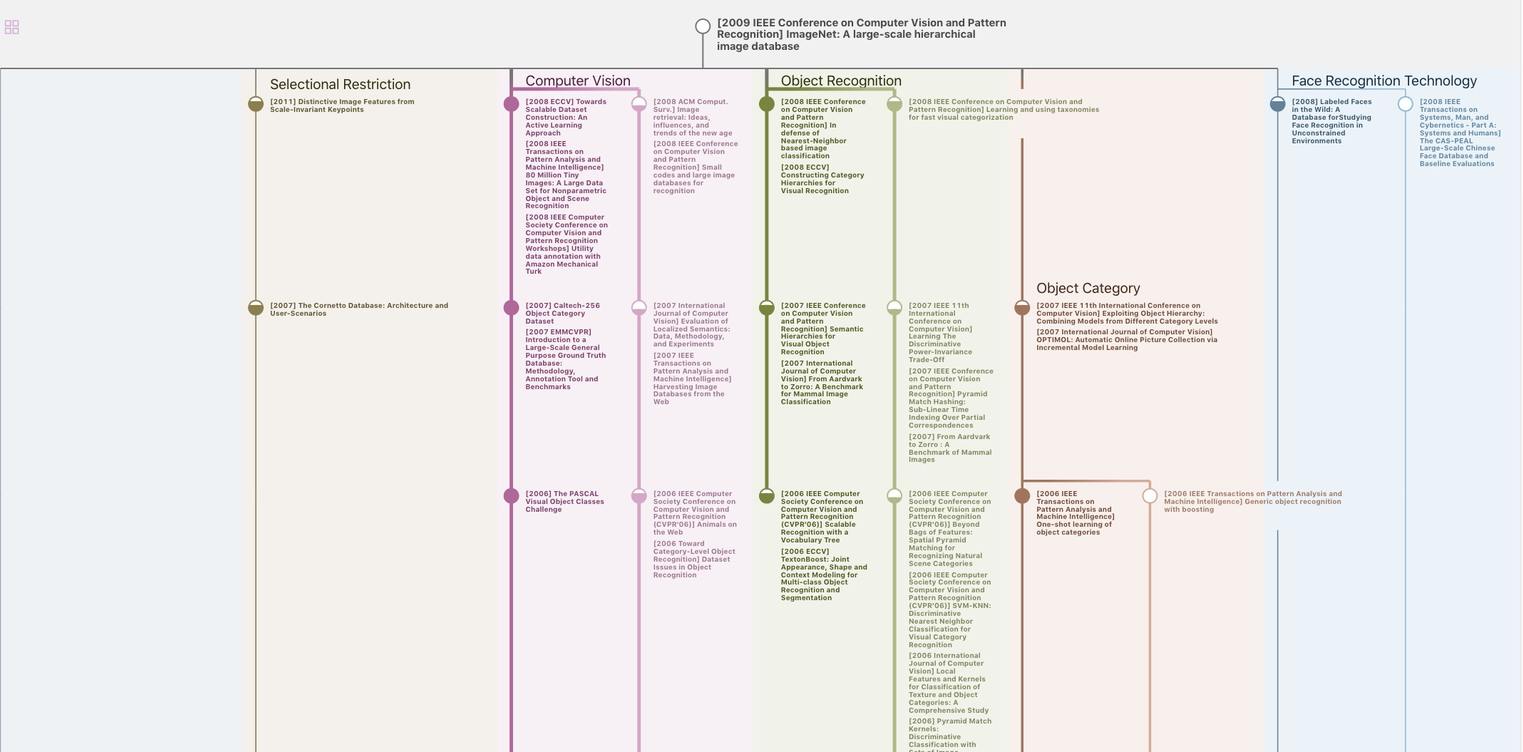
生成溯源树,研究论文发展脉络
Chat Paper
正在生成论文摘要