Scalable Architecture, Storage and Visualization Approaches for Time Series Analysis Systems
international conference on data technologies and applications(2019)
摘要
In order to adapt to the recent phenomenon of exponential growth of time series data sets in both academic and commercial environments, and with the goal of deriving valuable knowledge from this data, a multitude of analysis software tools have been developed to allow groups of collaborating researchers to find and annotate meaningful behavioral patterns. However, these tools commonly lack appropriate mechanisms to handle massive time series data sets of high cardinality, as well as suitable visual encodings for annotated data. In this paper we conduct a comparative study of architectural, persistence and visualization methods that can enable these analysis tools to scale with a continuously-growing data set and handle intense workloads of concurrent traffic. We implement these approaches within a web platform, integrated with authentication, versioning, and locking mechanisms that prevent overlapping contributions or unsanctioned changes. Additionally, we measure the performance of a set of databases when writing and reading varying amounts of series data points, as well as the performance of different architectural models at scale.
更多查看译文
关键词
Time series, Annotations, Annotation systems, Collaborative software, Data analysis, Information science, Data modeling, Knowledge management, Database management systems, Time series databases distributed systems, Software architecture, Information visualization
AI 理解论文
溯源树
样例
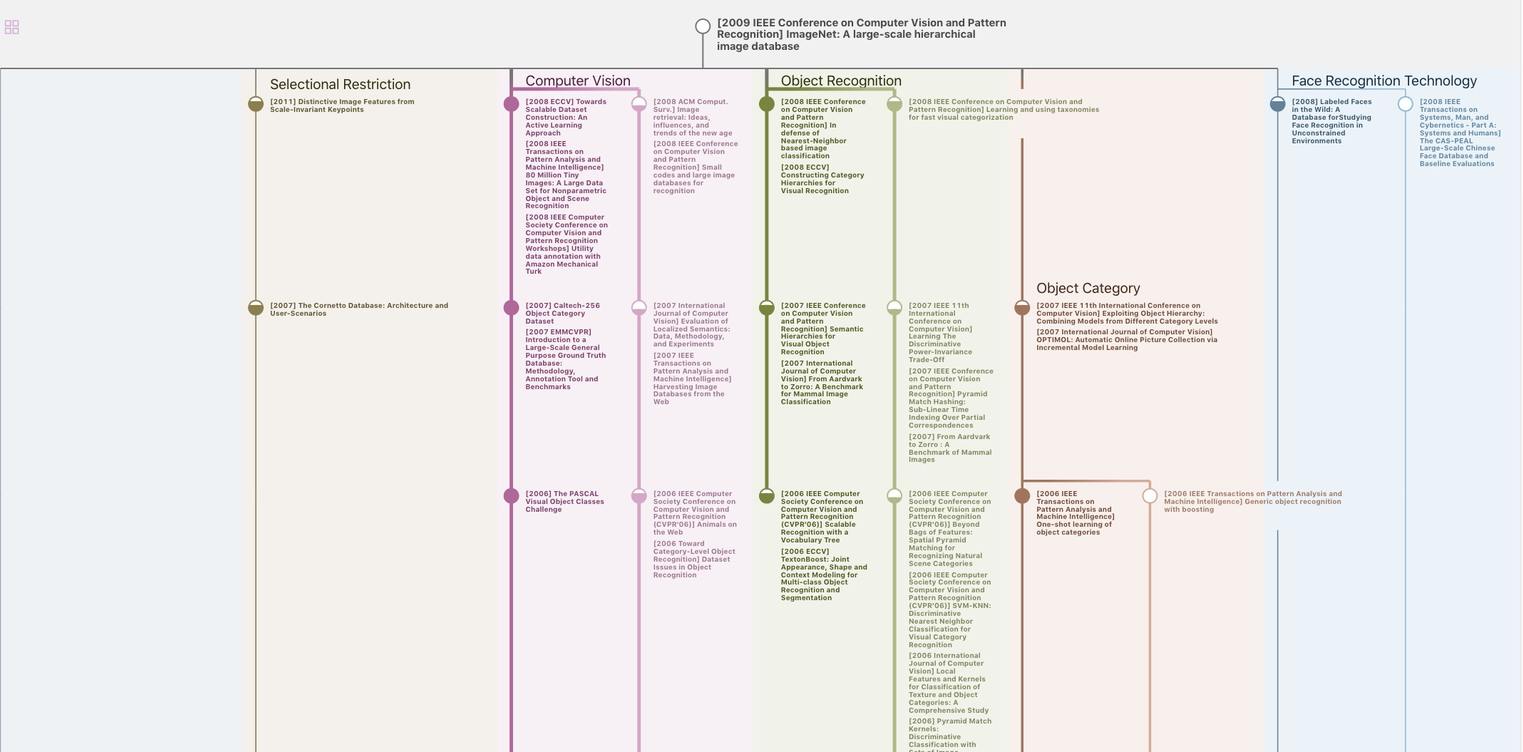
生成溯源树,研究论文发展脉络
Chat Paper
正在生成论文摘要