Maximum likelihood estimation based on the Laplace approximation for p2 network regression models
Statistica Neerlandica(2020)
摘要
The class ofp(2)models is suitable for modeling binary relation data in social network analysis. Ap(2)model is essentially a regression model for bivariate binary responses, featuring within-dyad dependence and correlated crossed random effects to represent heterogeneity of actors. Despite some desirable properties, these models are used less frequently in empirical applications than other models for network data. A possible reason for this is due to the limited possibilities for this model for accounting for (and explicitly modeling) structural dependence beyond the dyad as can be done in exponential random graph models. Another motive, however, may lie in the computational difficulties existing to estimate such models by means of the methods proposed in the literature, such as joint maximization methods and Bayesian methods. The aim of this article is to investigate maximum likelihood estimation based on the Laplace approximation approach, that can be refined by importance sampling. Practical implementation of such methods can be performed in an efficient manner, and the article provides details on a software implementation usingR. Numerical examples and simulation studies illustrate the methodology.
更多查看译文
关键词
automatic differentiation, importance sampling, numerical integration, random effects, social network analysis
AI 理解论文
溯源树
样例
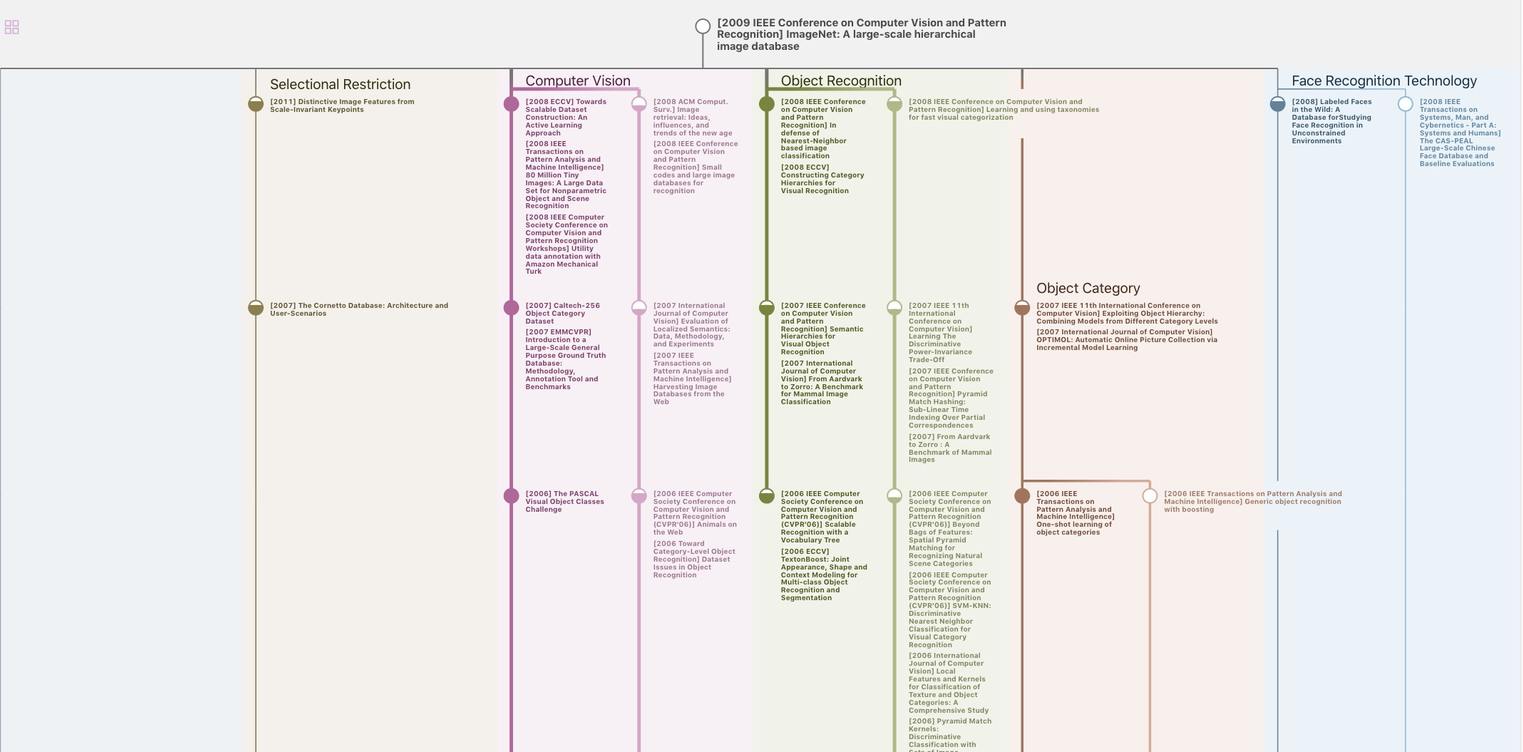
生成溯源树,研究论文发展脉络
Chat Paper
正在生成论文摘要