Detecting and Tracking Sinkholes Using Multi-Level Convolutional Neural Networks and Data Association
IEEE ACCESS(2020)
摘要
Sinkholes can cause severe property damage and threaten public safety. Therefore, the early prediction and detection of sinkholes are important measures for protecting both citizenry and infrastructure. Although many studies have made significant progress on sinkhole detection, challenges remain, including long-term data collection and the discovery of lightweight machine learning models that can be deployed to analyze sinkhole images. In this paper, we propose a method that takes advantage of the recent success of deep learning models to detect and track sinkholes via video streaming. Our system consists of two main stages: sinkhole detection with a cascaded convolutional neural network and sinkhole tracking with a data association algorithm. The experimental results show that a sinkhole can be tracked in real time using the dataset [1]. Furthermore, we implement the system on a Jetson TX2 embedded board (weighing 85 grams), which can operate at 13.2 FPS (frames per second). With an average IoU (intersection over union) score of 88% for sinkhole tracking and an accuracy of 97,6% for sinkhole detection on a 45-minute dataset, this study demonstrates the feasibility of sinkhole detection and tracking using IR images and their suitability for practical applications.
更多查看译文
关键词
Cameras,Monitoring,Convolutional neural networks,Machine learning,Data models,Laser radar,Temperature measurement,Sinkhole detection,convolutional neural network,imagenet,embedded system,data association
AI 理解论文
溯源树
样例
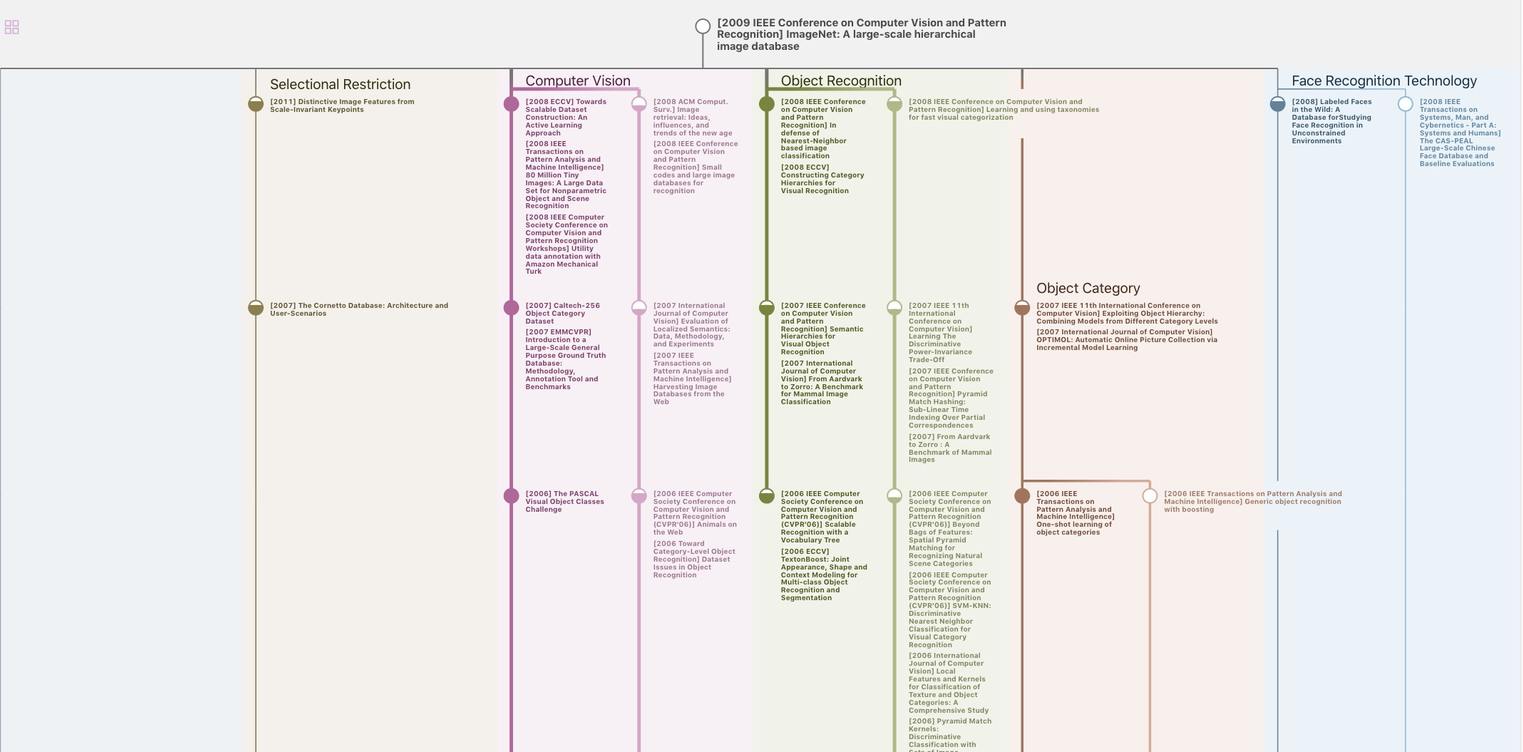
生成溯源树,研究论文发展脉络
Chat Paper
正在生成论文摘要