Counterfactual Analysis and Inference With Nonstationary Data
JOURNAL OF BUSINESS & ECONOMIC STATISTICS(2022)
摘要
Recently, there has been growing interest in developing econometric tools to conduct counterfactual analysis with aggregate data when a single "treated" unit suffers an intervention, such as a policy change, and there is no obvious control group. Usually, the proposed methods are based on the construction of an artificial/synthetic counterfactual from a pool of "untreated" peers, organized in a panel data structure. In this article, we investigate the consequences of applying such methodologies when the data comprise integrated processes of order 1, I(1), or are trend-stationary. We find that for I(1) processes without a cointegrating relationship (spurious case) the estimator of the effects of the intervention diverges, regardless of its existence. Although spurious regression is a well-known concept in time-series econometrics, they have been ignored in most of the literature on counterfactual estimation based on artificial/synthetic controls. For the case when at least one cointegration relationship exists, we have consistent estimators for the intervention effect albeit with a nonstandard distribution. Finally, we discuss a test based on resampling which can be applied when there is at least one cointegration relationship or when the data are trend-stationary.
更多查看译文
关键词
ArCo, Cointegration, Counterfactual analysis, Nonstationarity, Policy evaluation, Synthetic control
AI 理解论文
溯源树
样例
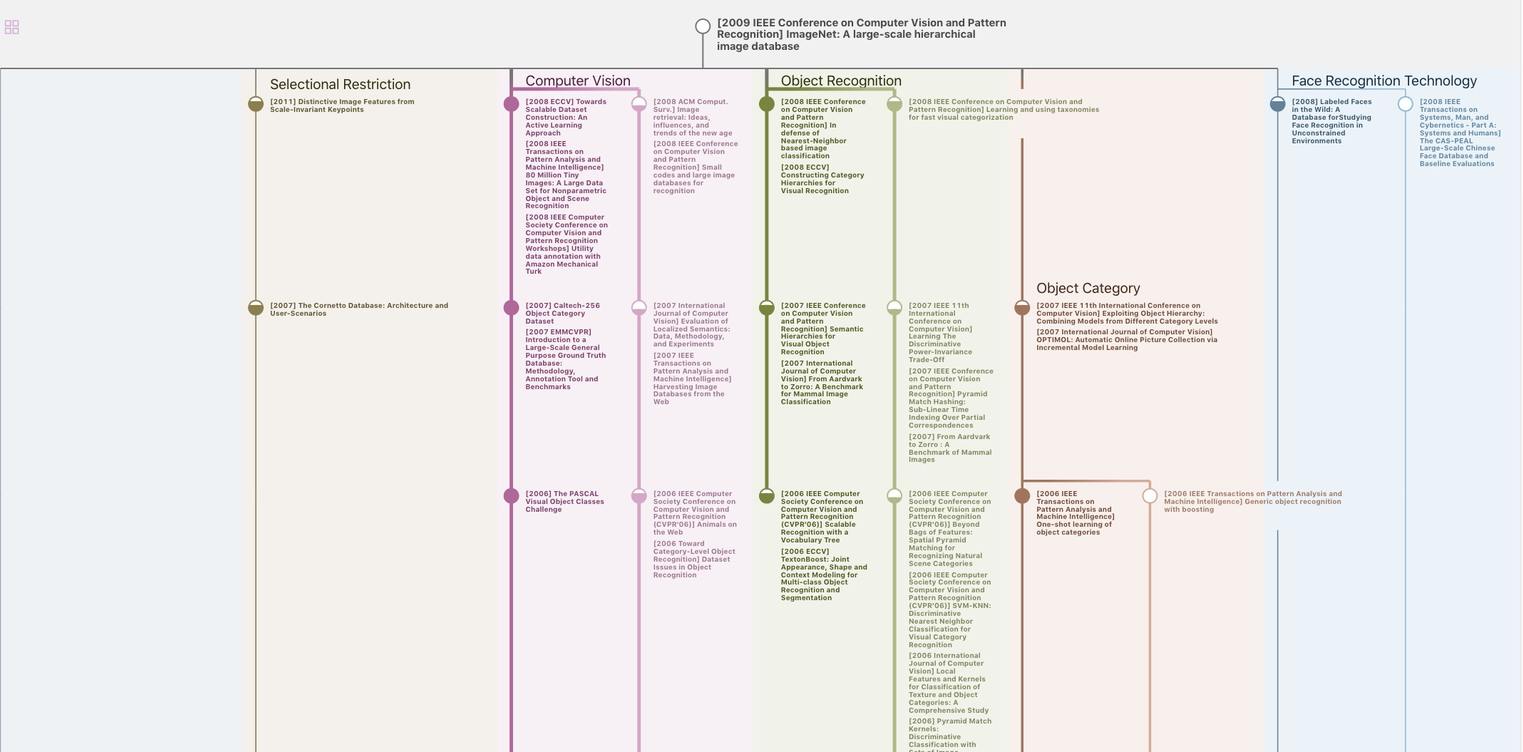
生成溯源树,研究论文发展脉络
Chat Paper
正在生成论文摘要