A data-driven lane-changing behavior detection system based on sequence learning
TRANSPORTMETRICA B-TRANSPORT DYNAMICS(2022)
摘要
Lane-changing detection is one of the most challenging tasks in advanced driver assistance system (ADAS). However, modeling driver's lane-changing process is challenging due to the complexity and uncertainty of driving behaviors. To address this issue, a novel sequential model, data-driven lane change detection (DLCD) system is proposed using deep learning techniques. Firstly, DLCD system explores to modeling driving context in spatial domain instead of traditional temporal domain. Secondly, DLCD has an ability of extracting innovative features, i.e. vehicle dynamics feature, lane boundary based distance feature and visual scene-centric feature from multi-modal input data efficiently. Finally, an improved focal loss-based deep long short-term memory (FL-LSTM) network is introduced to learn co-occurrence features and capture the dependencies within lane change events simultaneously. The experimental results on a real-world driving data set show that the DLCD system can learn the latent features of lane change behaviors and significantly outperform other advanced models.
更多查看译文
关键词
Lane change detection, sequence learning, ADAS, deep LSTM
AI 理解论文
溯源树
样例
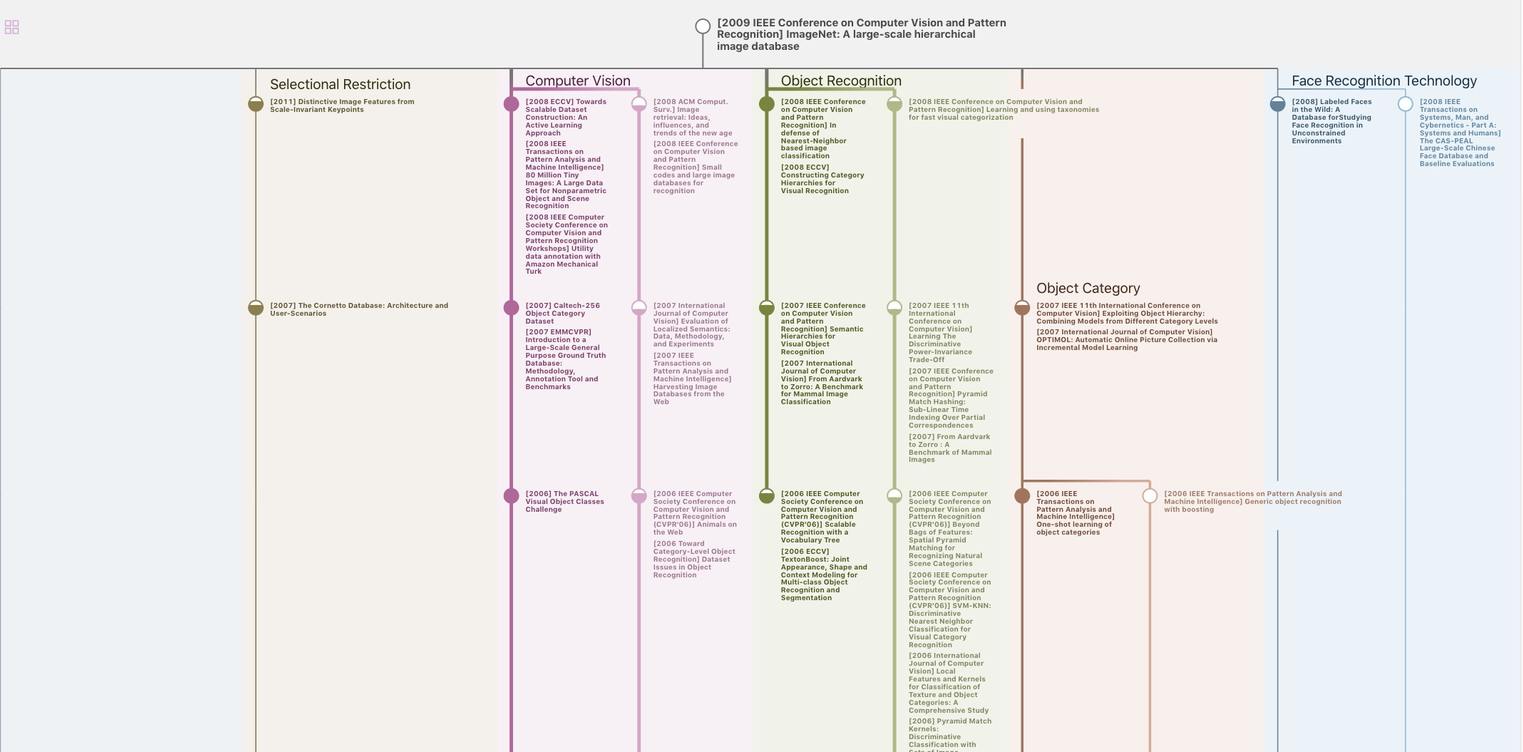
生成溯源树,研究论文发展脉络
Chat Paper
正在生成论文摘要