Verify and measure the quality of rule based machine leaning
Knowledge-Based Systems(2020)
摘要
In recent years, explainable AI has been gaining great attention, and there is a surge of interest in studying how prediction models work and how to provide formal guarantees for the models. Rule based machine learning (RBML), which aims to automatically identify and learn a set of relational rules that collectively represent the knowledge captured by the system, are a popular class of techniques in machine learning and data mining. Since inconsistencies in the rule base learnt can have a significant, negative impact on how the system will perform and on the conclusions that it will reach, the present work addresses the issues of verification and evaluation of consistency of rule base resulted from machine learning or domain expert using the logic based automated reasoning method. The main contribution consists of two parts. The first one focused on the consistency of rule base in the classical logic sense, which can be transformed into conjunctive normal form, so the consistency of rule base learnt can be verified via a resolution based automated reasoning method. Due to the uncertainty inevitably included in the rule-base during the learning process, the more detailed work has been presented in the second part, i.e., focused on providing a formal foundation of RBML under uncertainty in order to support logical analysis, verify and measure the consistency degree of the rule-base under uncertainty based on many-valued logic automated reasoning framework and algorithms. Some examples are also provided in both parts to illustrate the feasibility and effectiveness of the present work.
更多查看译文
关键词
Rule-based machine learning,Consistency,Uncertainty,Many-valued logic,Automated reasoning
AI 理解论文
溯源树
样例
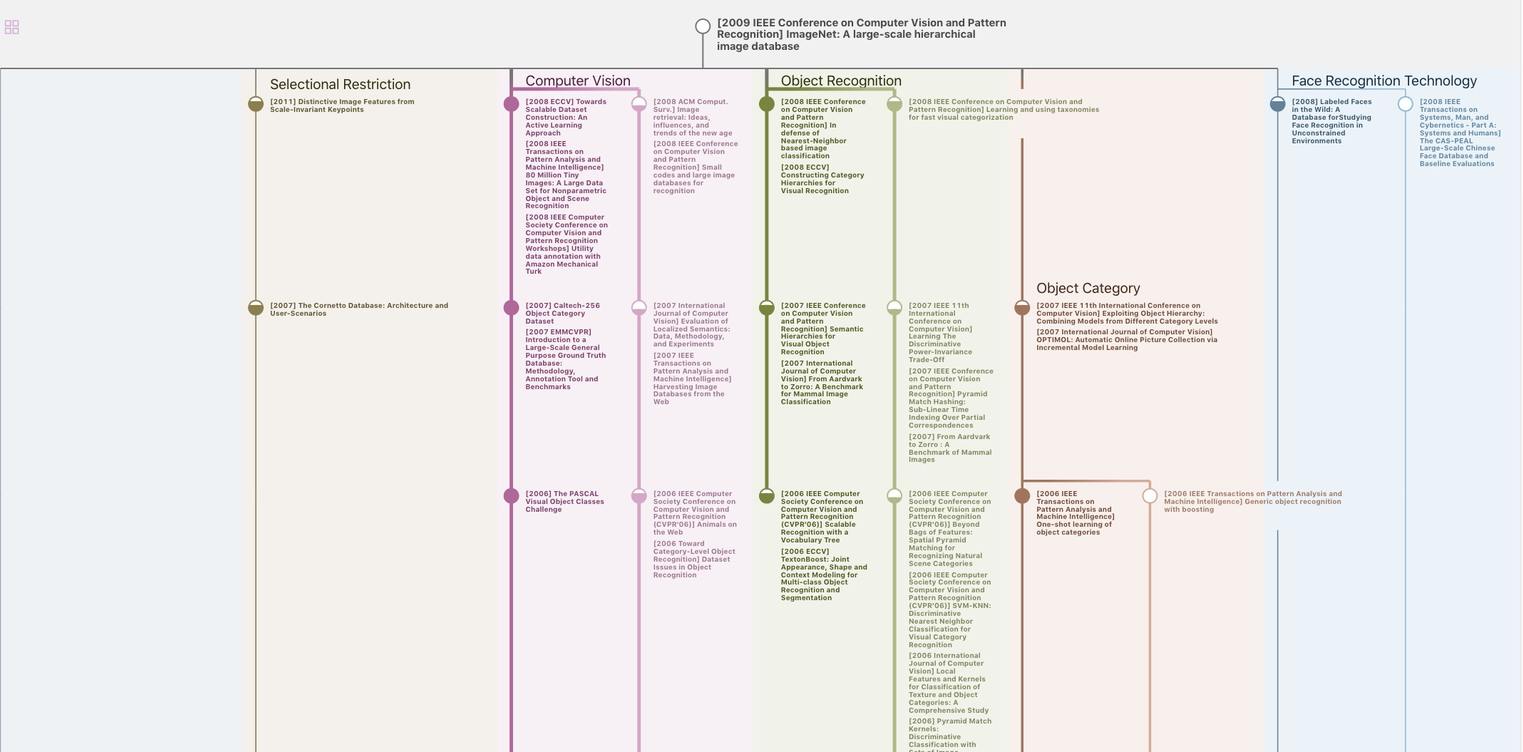
生成溯源树,研究论文发展脉络
Chat Paper
正在生成论文摘要