A Novel Inherent Distinguishing Feature Selector for Highly Skewed Text Document Classification
ARABIAN JOURNAL FOR SCIENCE AND ENGINEERING(2020)
摘要
major problem text classification faces is the high dimensional feature space of the text data. Feature selection (FS) algorithms are used for eliminating the irrelevant and redundant terms, thus increasing accuracy and speed of a text classifier. For text classification, FS algorithms have to be designed keeping the highly imbalanced classes of the text data in view. To this end, more recently ensemble algorithms (e.g., improved global feature selection scheme (IGFSS) and variable global feature selection scheme (VGFSS)) were proposed. These algorithms, which combine local and global FS metrics, have shown promising results with VGFSS having better capability of addressing the class imbalance issue. However, both these schemes are highly dependent on the underlying local and global FS metrics. Existing FS metrics get confused while selecting relevant terms of a data with highly imbalanced classes. In this paper, we propose a new FS metric named inherent distinguished feature selector (IDFS), which selects terms having greater relevance to classes and is highly effective for imbalanced data sets. We compare performance of IDFS against five well-known FS metrics as a stand-alone FS algorithm and as a part of the IGFSS and VGFSS frameworks on five benchmark data sets using two classifiers, namely support vector machines and random forests. Our results show that IDFS in both scenarios selects smaller subsets, and achieves higher micro and macro F_1 values, thus outperforming the existing FS metrics.
更多查看译文
关键词
Text document classification, Feature selection, Feature ranking metrics
AI 理解论文
溯源树
样例
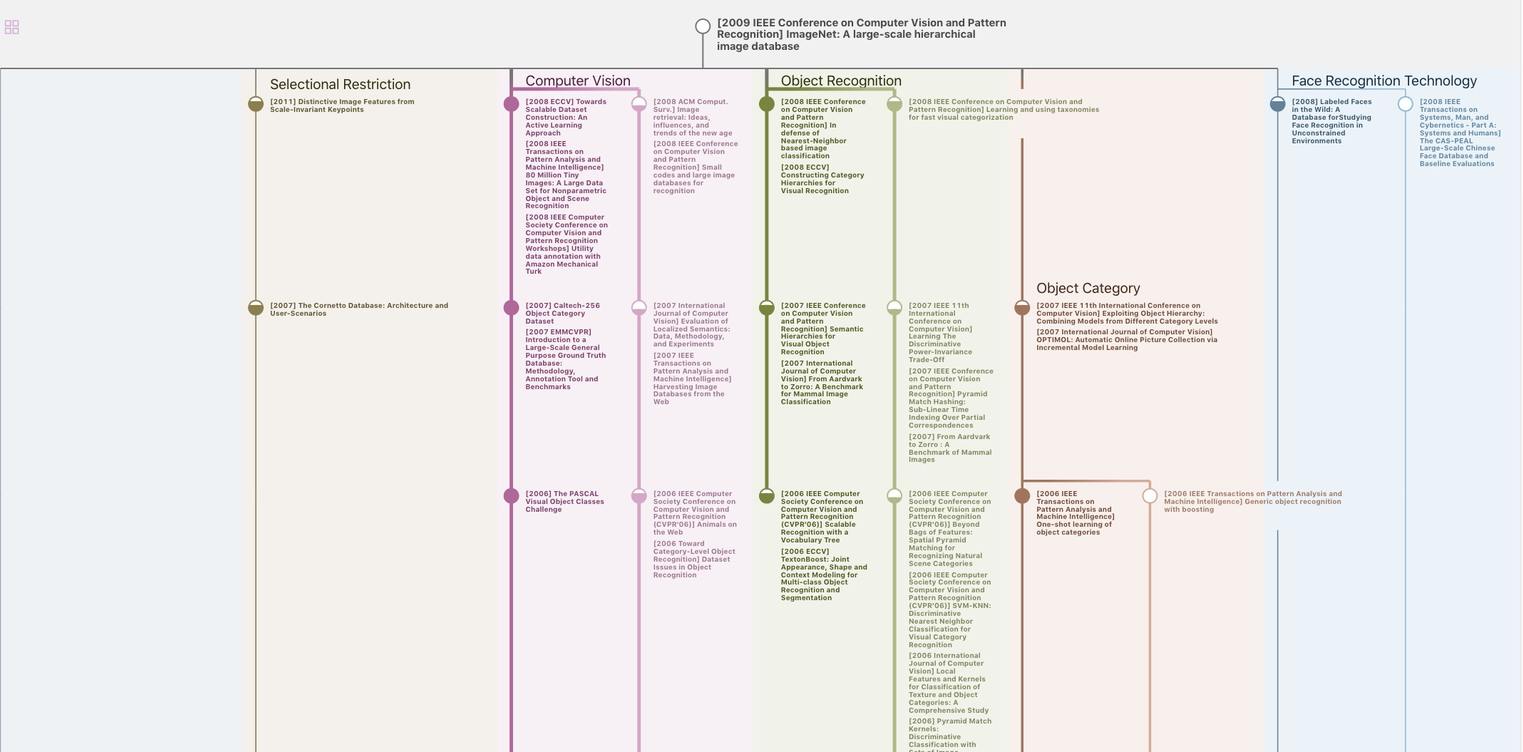
生成溯源树,研究论文发展脉络
Chat Paper
正在生成论文摘要