Double-Talk Robust Multichannel Acoustic Echo Cancellation Using Least-Squares Mimo Adaptive Filtering: Transversal, Array, And Lattice Forms
IEEE TRANSACTIONS ON SIGNAL PROCESSING(2020)
摘要
In this paper, we address the problem of noise-robust multiple-input multiple-output (MIMO) adaptive filtering that is optimal in least-squares sense with application to multichannel acoustic echo cancellation. We formulate the problem as minimization of a multichannel least-squares cost function that incorporates near-end speech and noise statistics resulting in a novel noise-robust framework for MIMO adaptive filtering. Although the issue of numerical stability has been widely explored in the context of recursive least-squares (RLS) filtering, a rigorous mathematical treatment of the MIMO case in the context of numerically stable noise-robust multichannel echo cancellation remains absent. Guided by quantization-error modeling, we resolve the issue of numerical instability in our noise-robust scheme by utilizing transversal RLS filtering of Type 2. Thereafter, an explicit derivation of its inverse QR-decomposition (IQRD) counterpart based on Givens rotations is presented. We also derive computationally efficient lattice forms for our noise-robust RLS Type-2 and IQRD algorithms. It is highlighted that propagation of angle-normalized errors occurs naturally within the numerically stable least-squares lattice (LSL). Thus, our approach combines the four sought after attributes in a multichannel echo cancellation scheme, i.e., computational efficiency, numerical stability, fast convergence and tracking, and robustness against noise. We analyze our formulations using simulations in terms of convergence, re-convergence, robustness in the presence of double-talk, and numerical stability.
更多查看译文
关键词
Noise robustness, Robustness, Echo cancellers, MIMO communication, Lattices, Microphones, Cost function, Recursive least-squares, noise-robust, least-squares lattice, inverse QRD, double-talk
AI 理解论文
溯源树
样例
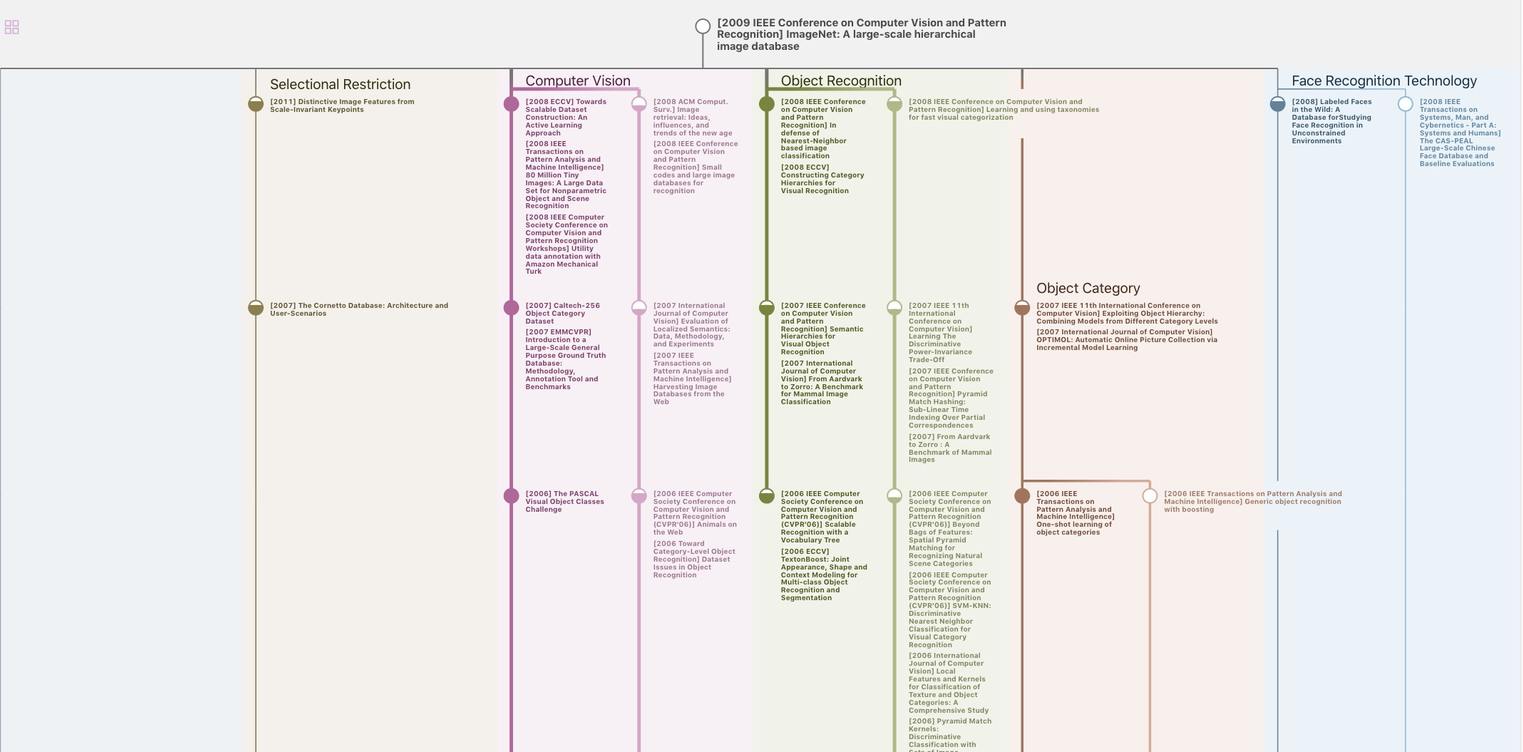
生成溯源树,研究论文发展脉络
Chat Paper
正在生成论文摘要