ID Preserving Face Super-Resolution Generative Adversarial Networks
IEEE ACCESS(2020)
摘要
We propose an ID Preserving Face Super-Resolution Generative Adversarial Networks (IP-FSRGAN) to reconstruct realistic super-resolution face images from low-resolution ones. Inspired by the success of generative adversarial networks (GAN), we introduce a novel ID preserving module to help the generator learn to infer the facial details and synthesize more realistic super-resolution faces. Our method produces satisfactory visual results and also quantitatively outperforms state-of-the-art super-resolution methods on the face datasets including CASIA-Webface, CelebA, and LFW datasets under the metrics of PSNR, SSIM, and cosine similarity. In addition, we propose a framework to apply IP-FSRGAN model to address the face verification task on low-resolution face images. The synthesized 4x super-resolution faces achieve a verification accuracy of 97.6%, improved from 92.8% of low resolution faces. We also prove by experiments that the proposed IP-FSRGAN model demonstrates excellent robustness under different downsample scaling factors and extensibility to various face verification models.
更多查看译文
关键词
ID preserving,face super-resolution,generative adversarial networks,face verification
AI 理解论文
溯源树
样例
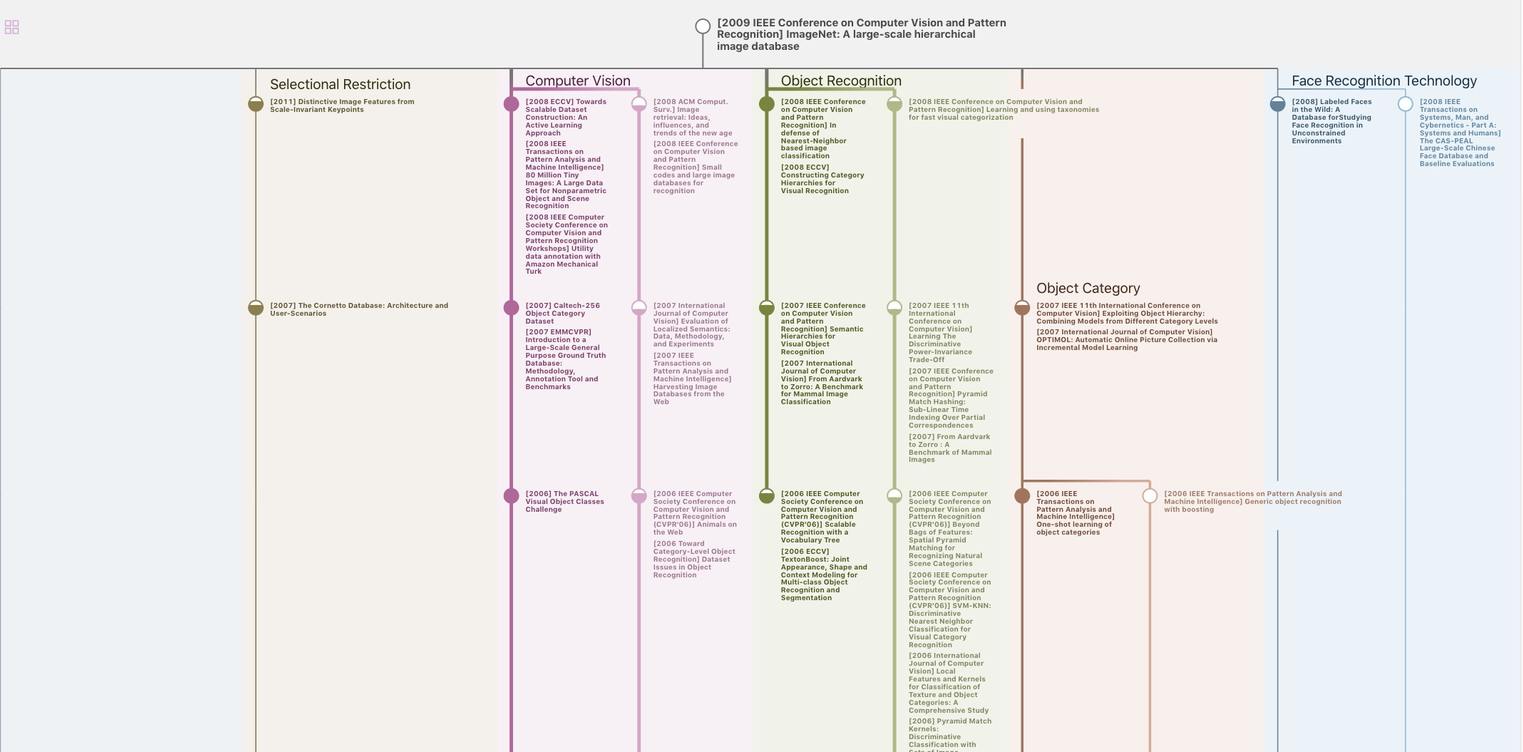
生成溯源树,研究论文发展脉络
Chat Paper
正在生成论文摘要