Construction of an indoor radio environment map using gradient boosting decision tree
WIRELESS NETWORKS(2020)
摘要
Radio environment maps represent a signal strength map or a coverage area of radio networks. Constructing such maps involves gathering signal coverage information in sparse locations, which can be conventionally performed by measurement methods such as the manual drive test. Nevertheless, as this process is large-scale, time-consuming, and costly, several methods for minimization of drive tests have been introduced. Machine learning is commonly used in solving regression or classification problems; in several studies, its performance even surpassed human abilities. In this study, we applied the gradient boosting algorithm to construct radio environment maps from sparse data gathered by user equipments. XGBoost and light gradient boosting machine were experimentally evaluated in constructing base station coverage, reference signal received power, reference signal received quality, and signal-to-noise ratio heatmaps, under various configuration settings. Results validated the superior performance of the two approaches against existing baseline methods k-nearest neighbor and support vector machine. Furthermore, we also assessed our model’s ability to construct radio environment maps based on unseen configuration settings, which confirmed reliable results even if they were trained using completely different sets of configuration settings.
更多查看译文
关键词
Radio environment map,Machine learning,Gradient boosting,Coverage predictions,Constructions methods,RSRP,RSRQ,SNR
AI 理解论文
溯源树
样例
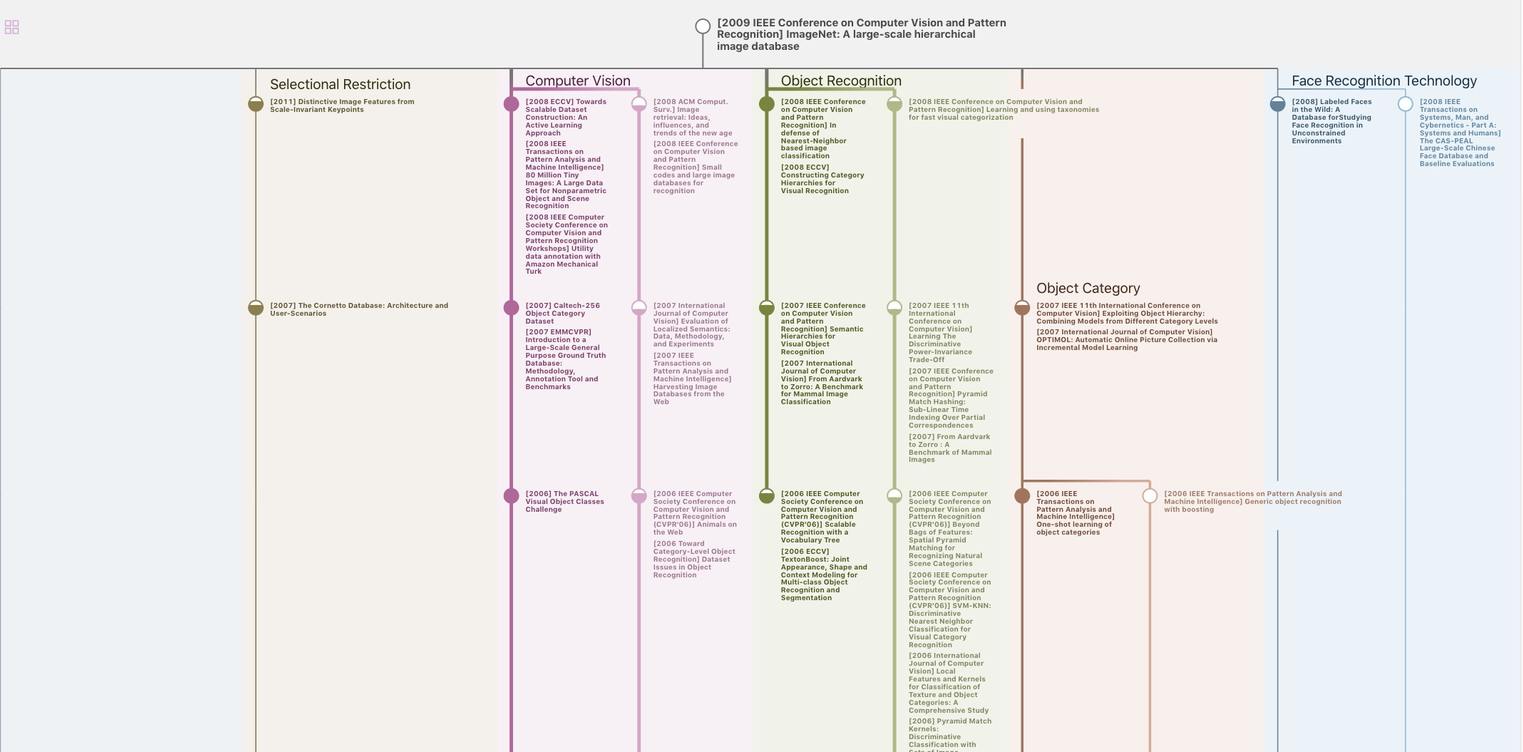
生成溯源树,研究论文发展脉络
Chat Paper
正在生成论文摘要