A bias-variance analysis of state-of-the-art random forest text classifiers
ADVANCES IN DATA ANALYSIS AND CLASSIFICATION(2020)
摘要
Random forest (RF) classifiers do excel in a variety of automatic classification tasks, such as topic categorization and sentiment analysis. Despite such advantages, RF models have been shown to perform poorly when facing noisy data, commonly found in textual data, for instance. Some RF variants have been proposed to provide better generalization capabilities under such challenging scenario, including lazy, boosted and randomized forests, all which exhibit significant reductions on error rate when compared to the traditional RFs. In this work, we analyze the behavior of such variants under the bias-variance decomposition of error rate. Such an analysis is of utmost importance to uncover the main causes of the observed improvements enjoyed by those variants in classification effectiveness. As we shall see, significant reductions in variance along with stability in bias explain a large portion of the improvements for the lazy and boosted RF variants. Such an analysis also sheds light on new promising directions for further enhancements in RF-based learners, such as the introduction of new randomization sources on both, lazy and boosted variants.
更多查看译文
关键词
Random forests, Text classification, Bias variance analysis
AI 理解论文
溯源树
样例
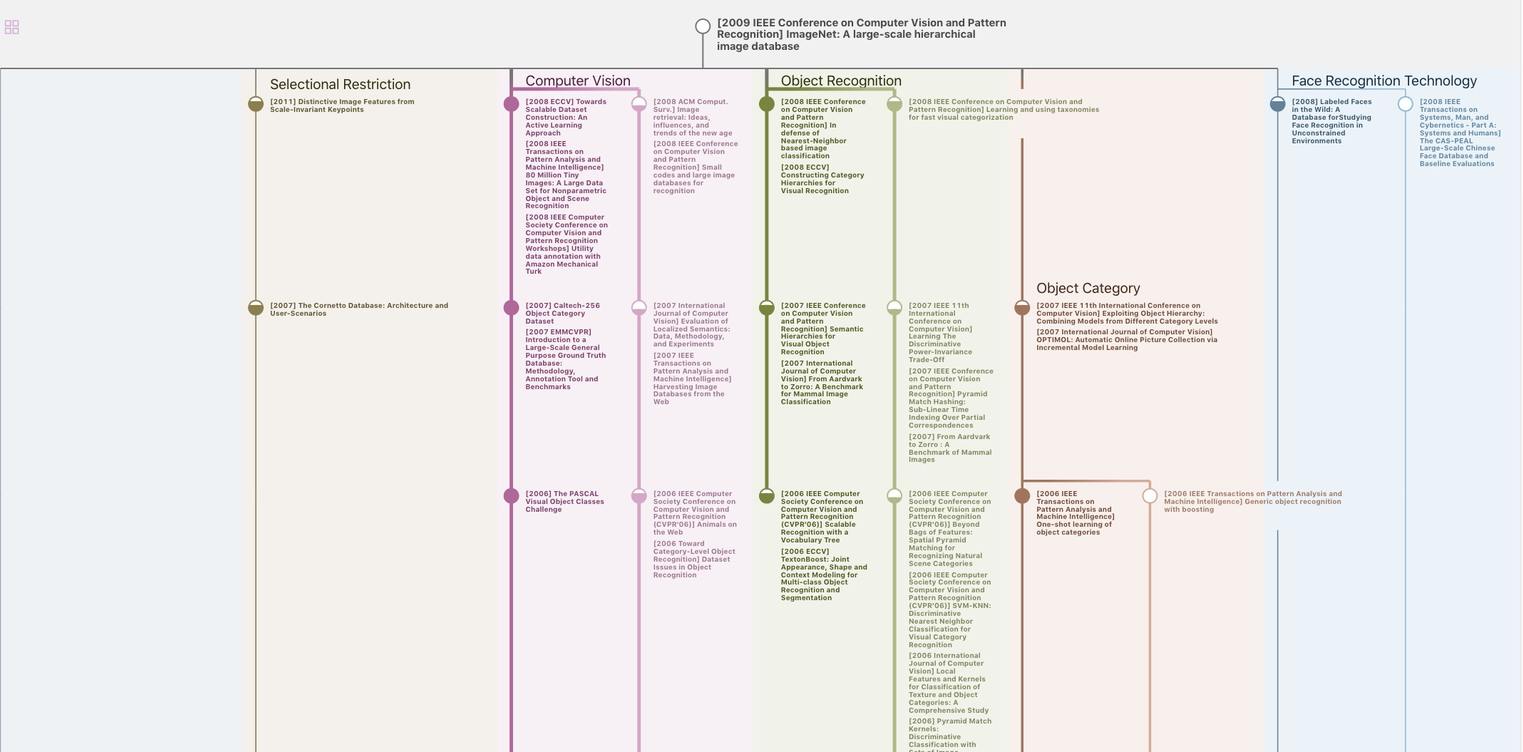
生成溯源树,研究论文发展脉络
Chat Paper
正在生成论文摘要