A Survey of the Four Pillars for Small Object Detection: Multiscale Representation, Contextual Information, Super-Resolution, and Region Proposal
IEEE Transactions on Systems, Man, and Cybernetics: Systems(2022)
摘要
Although great progress has been made in generic object detection by advanced deep learning techniques, detecting small objects from images is still a difficult and challenging problem in the field of computer vision due to the limited size, less appearance, and geometry cues, and the lack of large-scale datasets of small targets. Improving the performance of small object detection has a wider significance in many real-world applications, such as self-driving cars, unmanned aerial vehicles, and robotics. In this article, the first-ever survey of recent studies in deep learning-based small object detection is presented. Our review begins with a brief introduction of the four pillars for small object detection, including
multiscale representation, contextual information, super-resolution, and region-proposal
. Then, the collection of state-of-the-art datasets for small object detection is listed. The performance of different methods on these datasets is reported later. Moreover, the state-of-the-art small object detection networks are investigated along with a special focus on the differences and modifications to improve the detection performance comparing to generic object detection architectures. Finally, several promising directions and tasks for future work in small object detection are provided. Researchers can track up-to-date studies on this webpage available at:
https://github.com/tjtum-chenlab/SmallObjectDetectionList
.
更多查看译文
关键词
Contextual information,multiscale representation,region proposal,small object dataset,small object detection,super-resolution
AI 理解论文
溯源树
样例
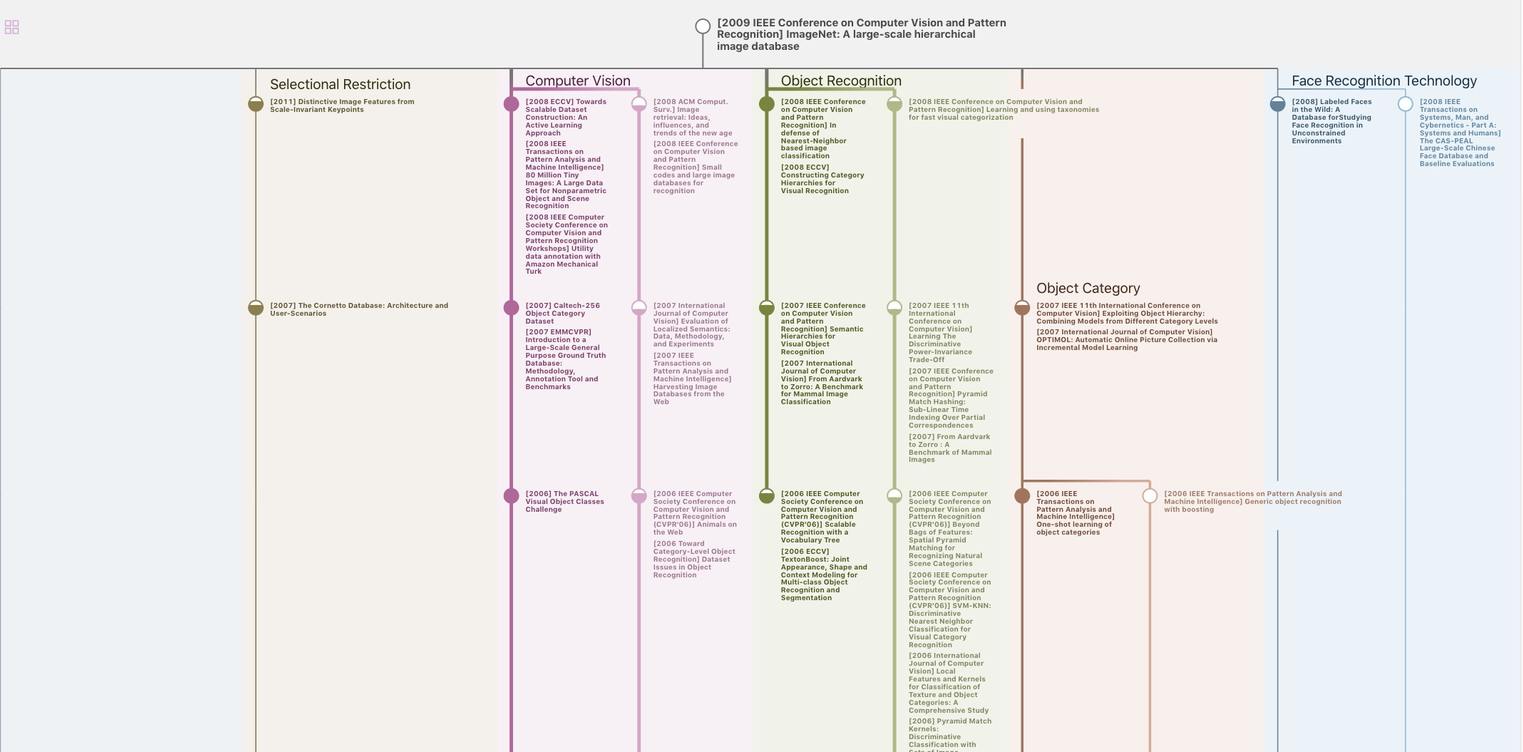
生成溯源树,研究论文发展脉络
Chat Paper
正在生成论文摘要